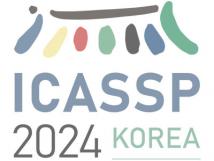
IEEE ICASSP 2024 - IEEE International Conference on Acoustics, Speech and Signal Processing (ICASSP) is the world’s largest and most comprehensive technical conference focused on signal processing and its applications. The IEEE ICASSP 2024 conference will feature world-class presentations by internationally renowned speakers, cutting-edge session topics and provide a fantastic opportunity to network with like-minded professionals from around the world. Visit the website.
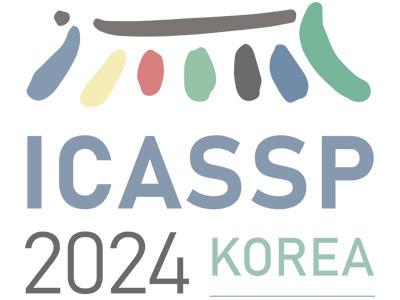
- Read more about Flattening Singular Values of Factorized Convolution for Medical Images
- Log in to post comments
Convolutional neural networks (CNNs) have long been the paradigm of choice for robust medical image processing (MIP). Therefore, it is crucial to effectively and efficiently deploy CNNs on devices with different computing capabil- ities to support computer-aided diagnosis. Many methods employ factorized convolutional layers to alleviate the bur- den of limited computational resources at the expense of expressiveness.
- Categories:
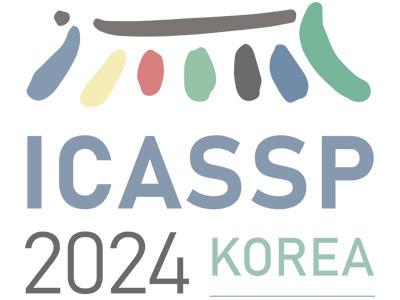
- Read more about Personalised Anomaly Detectors and Prototypical Representations for Relapse Detection from Wearable-Based Digital Phenotyping
- Log in to post comments
We describe our contribution to the 2nd e-Prevention challenge, which focuses on the unsupervised non-psychotic (Track 1) and psychotic (Track 2) relapse detection using wearable-based digital phenotyping. We exploit the measurements gathered from the gyroscope, the accelerometer, and the heart rate-related sensors embedded in a smartwatch. We also include the available sleep information in our experiments. Four dedicated autoencoders are trained to learn embedded representations from each one of the considered modalities.
- Categories:
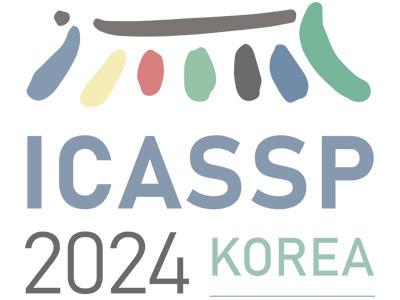
- Read more about Tunisian Code Switched ASR Presentation
- Log in to post comments
Crafting an effective Automatic Speech Recognition (ASR) solution for dialects demands innovative approaches that not only address the data scarcity issue but also navigate the intricacies of linguistic diversity. In this paper, we address the aforementioned ASR challenge, focusing on the Tunisian dialect. First, textual and audio data is collected and in some cases annotated.
- Categories:
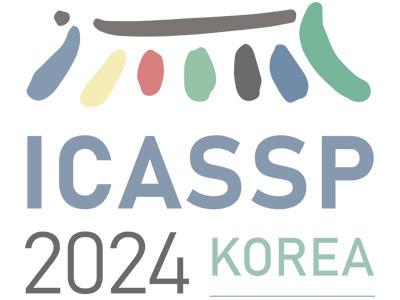
- Read more about TALKNCE: IMPROVING ACTIVE SPEAKER DETECTION WITH TALK-AWARE CONTRASTIVE LEARNING
- Log in to post comments
The goal of this work is Active Speaker Detection (ASD), a task to determine whether a person is speaking or not in a series of video frames.
Previous works have dealt with the task by exploring network architectures while learning effective representations has been less explored.
In this work, we propose TalkNCE, a novel talk-aware contrastive loss. The loss is only applied to part of the full segments where a person on the screen is actually speaking.
- Categories:
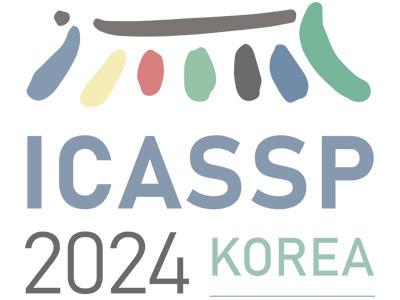
- Read more about SCORE-BASED DIFFUSION MODELS FOR PHOTOACOUSTIC TOMOGRAPHY IMAGE RECONSTRUCTION
- Log in to post comments
Photoacoustic tomography (PAT) is a rapidly-evolving medical imaging modality that combines optical absorption contrast with ultrasound imaging depth. One challenge in PAT is image reconstruction with inadequate acoustic signals due to limited sensor coverage or due to the density of the transducer array. Such cases call for solving an ill-posed inverse reconstruction problem. In this work, we use score-based diffusion models to solve the inverse problem of reconstructing an image from limited PAT measurements.
- Categories:
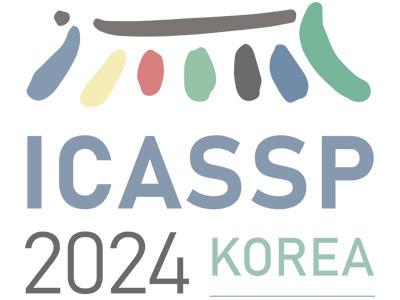
- Read more about MUSICLDM: ENHANCING NOVELTY IN TEXT-TO-MUSIC GENERATION USING BEAT-SYNCHRONOUS MIXUP STRATEGIES
- Log in to post comments
Diffusion models have shown promising results in cross-modal generation tasks, including text-to-image and text-to-audio generation. However, generating music, as a special type of audio, presents unique challenges due to limited availability of music data and sensitive issues related to copyright and plagiarism. In this paper, to tackle these challenges, we first construct a state-of-the-art text-to-music model, MusicLDM, that adapts Stable Diffusion and AudioLDM architectures to the music domain.
- Categories:
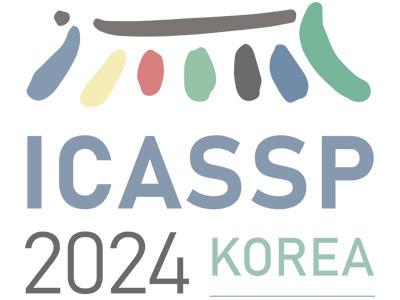
- Read more about Channel Estimation in Underdetermined Systems Utilizing Variational Autoencoders
- Log in to post comments
In this work, we propose to utilize a variational autoencoder (VAE) for channel estimation (CE) in underdetermined (UD) systems. The basis of the method forms a recently proposed concept in which a VAE is trained on channel state information (CSI) data and used to parameterize an approximation to the mean squared error (MSE)-optimal estimator. The contributions in this work extend the existing framework from fully-determined (FD) to UD systems, which are of high practical relevance.
- Categories:
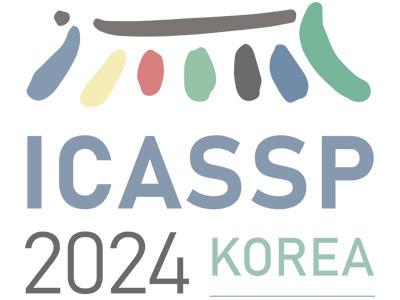
- Read more about MDX-GAN: ENHANCING PERCEPTUAL QUALITY IN MULTI-CLASS SOURCE SEPARATION VIA ADVERSARIAL TRAINING
- Log in to post comments
Audio source separation aims to extract individual sound sources from an audio mixture. Recent studies on source separation focus primarily on minimizing signal-level distance, typically measured by source-to-distortion ratio (SDR). However, scant attention has been given to the perceptual quality of the separated tracks. In this paper, we propose MDX-GAN, an efficient and high-fidelity audio source separator based on MDX-Net for multiple sound classes. We leverage different training objectives to enhance the perceptual quality of audio source separation.
- Categories:
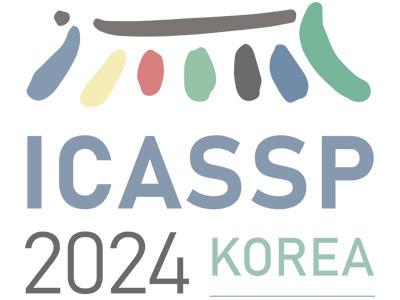
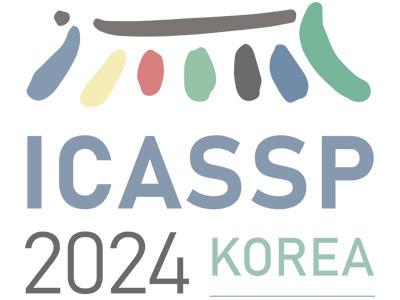
- Read more about DIFFUSION-BASED SPEECH ENHANCEMENT IN MATCHED AND MISMATCHED CONDITIONS USING A HEUN-BASED SAMPLER
- Log in to post comments
Diffusion models are a new class of generative models that have recently been applied to speech enhancement successfully. Previous works have demonstrated their superior performance in mismatched conditions compared to state-of-the art discriminative models. However, this was investigated with a single database for training and another one for testing, which makes the results highly dependent on the particular databases. Moreover, recent developments from the image generation literature remain largely unexplored for speech enhancement.
- Categories: