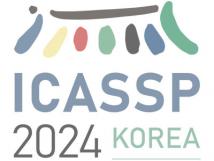
IEEE ICASSP 2024 - IEEE International Conference on Acoustics, Speech and Signal Processing (ICASSP) is the world’s largest and most comprehensive technical conference focused on signal processing and its applications. The IEEE ICASSP 2024 conference will feature world-class presentations by internationally renowned speakers, cutting-edge session topics and provide a fantastic opportunity to network with like-minded professionals from around the world. Visit the website.
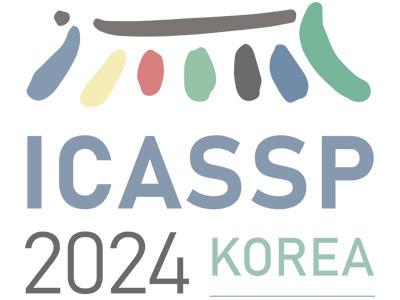
- Read more about Poster for the paper "Buffered Gaussian Modeling For Vectorized HD Map Construction"
- Log in to post comments
Vectorized high-definition (HD) map construction is an important and challenging task for autonomous driving. End-to-end models have been developed recently to enable online map construction. Existing works have difficulty in generating complex geometric shapes and lack comprehensive evaluation metrics. To tackle these challenges, we introduce buffered IoU as a novel metric for vectorized map construction, which is clearly defined and applicable to real-world situations. Inspired by methods of rotated object detection, we further propose a novel technique called Buffered Gaussian Modeling.
- Categories:
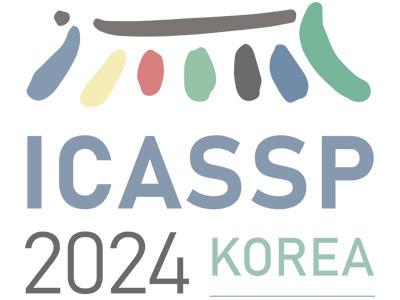
- Read more about Lightning Talk- Situation-Aware Tranmit Beamforming for Automotive radar
- Log in to post comments
Millimeter-wave radar is a common sensor modality used in automotive driving for target detection and perception. These radars can benefit from side information on the environment being sensed, such as lane topologies or data from other sensors. Existing radars do not leverage this information to adapt waveforms or perform prior-aware inference. In this paper, we model the side information as an occupancy map and design transmit beamformers that are customized to the map. Our method maximizes the probability of detection in regions with a higher uncertainty on the presence of a target.
- Categories:
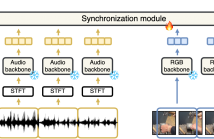
- Read more about Poster: Synchformer: Efficient Synchronization from Sparse Cues
- Log in to post comments
Our objective is audio-visual synchronization with a focus on ‘in-the-wild’ videos, such as those on YouTube, where synchronization cues can be sparse. Our contributions include a novel audio-visual synchronization model, and training that decouples feature extraction from synchronization modelling through multi-modal segment-level contrastive pre-training. This approach achieves state-of-the-art performance in both dense and sparse settings.
- Categories:
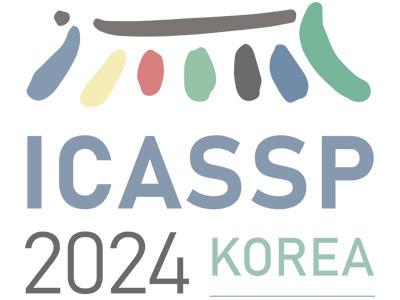
- Read more about INVESTIGATING THE CLUSTERS DISCOVERED BY PRE-TRAINED AV-HUBERT
- Log in to post comments
Self-supervised models, such as HuBERT and its audio-visual version AV-HuBERT, have demonstrated excellent performance on various tasks. The main factor for their success is the pre-training procedure, which requires only raw data without human transcription. During the self-supervised pre-training phase, HuBERT is trained to discover latent clusters in the training data, but these clusters are discarded, and only the last hidden layer is used by the conventional finetuning step.
- Categories:
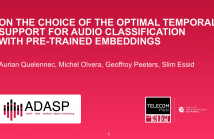
- Read more about ON THE CHOICE OF THE OPTIMAL TEMPORAL SUPPORT FOR AUDIO CLASSIFICATION WITH PRE-TRAINED EMBEDDINGS
- Log in to post comments
Current state-of-the-art audio analysis systems rely on pre-trained embedding models, often used off-the-shelf as (frozen) feature extractors. Choosing the best one for a set of tasks is the subject of many recent publications. However, one aspect often overlooked in these works is the influence of the duration of audio input considered to extract an embedding, which we refer to as Temporal Support (TS). In this work, we study the influence of the TS for well-established or emerging pre-trained embeddings, chosen to represent different types of architectures and learning paradigms.
- Categories:
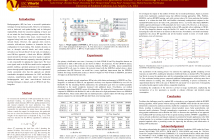
- Read more about UNLOCKING DEEP LEARNING: A BP-FREE APPROACH FOR PARALLEL BLOCK-WISE TRAINING OF NEURAL NETWORKS
- Log in to post comments
Backpropagation (BP) has been a successful optimization technique for deep learning models. However, its limitations, such as backward- and update-locking, and its biological implausibility, hinder the concurrent updating of layers and do not mimic the local learning processes observed in the human brain. To address these issues, recent research has suggested using local error signals to asynchronously train network blocks. However, this approach often involves extensive trial-and-error iterations to determine the best configuration for local training.
- Categories:
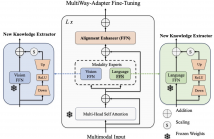
- Read more about MultiWay-Adapter: Adapting Multimodal Large Language Models for scalable image-text retrieval
- Log in to post comments
As Multimodal Large Language Models (MLLMs) grow in size, adapting them to specialized tasks becomes increasingly challenging due to high computational and memory demands. While efficient adaptation methods exist, in practice they suffer from shallow inter-modal alignment, which severely hurts model effectiveness. To tackle these challenges, we introduce the MultiWay-Adapter (MWA), which deepens inter-modal alignment, enabling high transferability with minimal tuning effort.
- Categories:
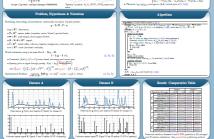
- Read more about PENDANTSS: PEnalized Norm-Ratios Disentangling Additive Noise, Trend and Sparse Spikes
- Log in to post comments
Denoising, detrending, deconvolution: usual restoration tasks, traditionally decoupled. Coupled formulations entail complex ill-posed inverse problems. We propose PENDANTSS for joint trend removal and blind deconvolution of sparse peak-like signals. It blends a parsimonious prior with the hypothesis that smooth trend and noise can somewhat be separated by low-pass filtering.
- Categories:
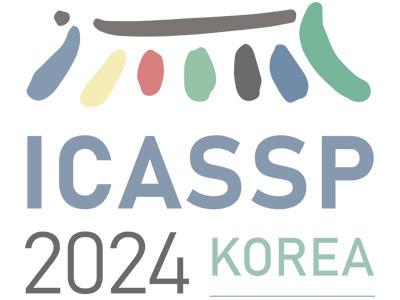
- Read more about LEARNING FROM TAXONOMY: MULTI-LABEL FEW-SHOT CLASSIFICATION FOR EVERYDAY SOUND RECOGNITION
- 1 comment
- Log in to post comments
Humans categorise and structure perceived acoustic signals into hierarchies of auditory objects. The semantics of these objects are thus informative in sound classification, especially in few-shot scenarios. However, existing works have only represented audio semantics as binary labels (e.g., whether a recording contains \textit{dog barking} or not), and thus failed to learn a more generic semantic relationship among labels. In this work, we introduce an ontology-aware framework to train multi-label few-shot audio networks with both relative and absolute relationships in an audio taxonomy.
- Categories:
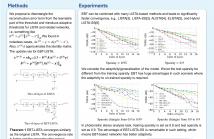
- Read more about LEARNED ISTA WITH ERROR-BASED THRESHOLDING FOR ADAPTIVE SPARSE CODING
- Log in to post comments
Drawing on theoretical insights, we advocate an error-based thresholding (EBT) mechanism for learned ISTA (LISTA), which utilizes a function of the layer-wise reconstruction error to suggest a specific threshold for each observation in the shrinkage function of each layer. We show that the proposed EBT mechanism well disentangles the learnable parameters in the shrinkage functions from the reconstruction errors, endowing the obtained models with improved adaptivity to possible data variations.
poster.pdf

- Categories: