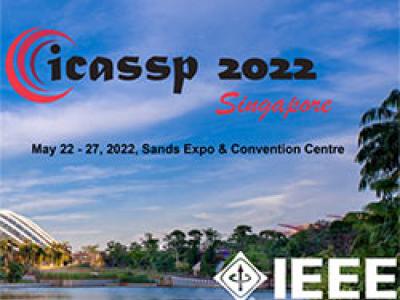
- Read more about Entrainment Analysis for Assessment of Autistic Speech Prosody Using Bottleneck Features of Deep Neural Network
- Log in to post comments
In the present study, we quantify entrainment characteristics of conversation with the aim of automatic assessment of the severity of autism spectrum disorder (ASD). We focus on pairs of utterances immediate before and after turn-takings, which have prosodic/acoustic similarities.
- Categories:
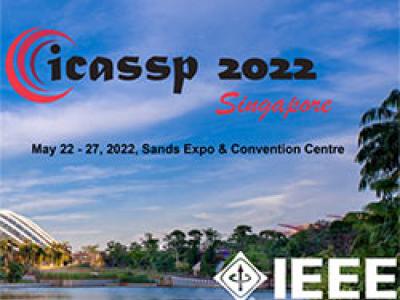
- Read more about Massive Unsourced Random Access Based on Bilinear Vector Approximate Message Passing Poster
- Log in to post comments
- Categories:
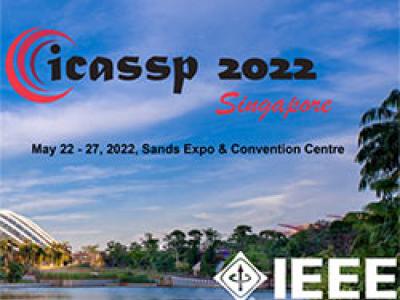
- Read more about MASSIVE UNSOURCED RANDOM ACCESS BASED ON BILINEAR VECTOR APPROXIMATE MESSAGE PASSING presentation
- Log in to post comments
- Categories:
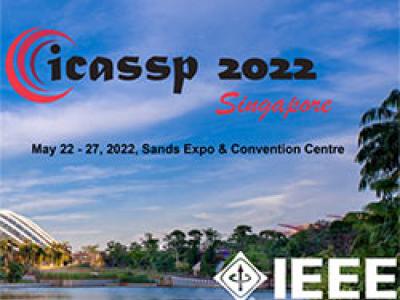
- Read more about A KNOWLEDGE/DATA ENHANCED METHOD FOR JOINT EVENT AND TEMP RELATION EXTRACTIONORAL
- Log in to post comments
Understanding temporal relations (TempRels) between events is an important task that could benefit many downstream NLP applications. This task inevitably faces the challenges of both a limited amount of high-quality training data and a very biased distribution of TempRels. These problems will substantially hurt the performance of extraction systems because they are inclined to predict dominant TempRels when training with a limited amount of data.
- Categories:
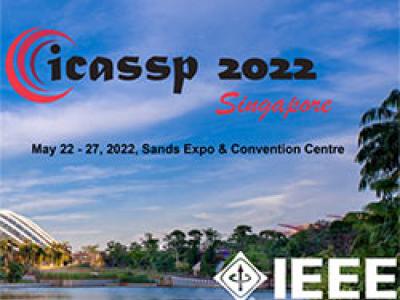
- Read more about Provable Sample Complexity Guarantees for Learning of Continuous-Action Graphical Games with Nonparametric Utilities
- Log in to post comments
- Categories:
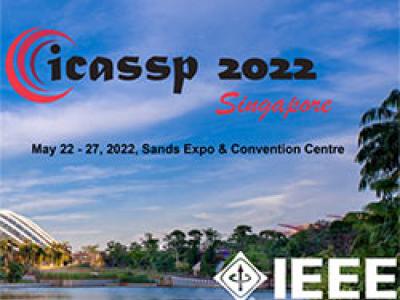
- Read more about Information Theoretic Limits for Standard and One-bit Compressed Sensing with Graph-structured Sparsity
- Log in to post comments
- Categories:
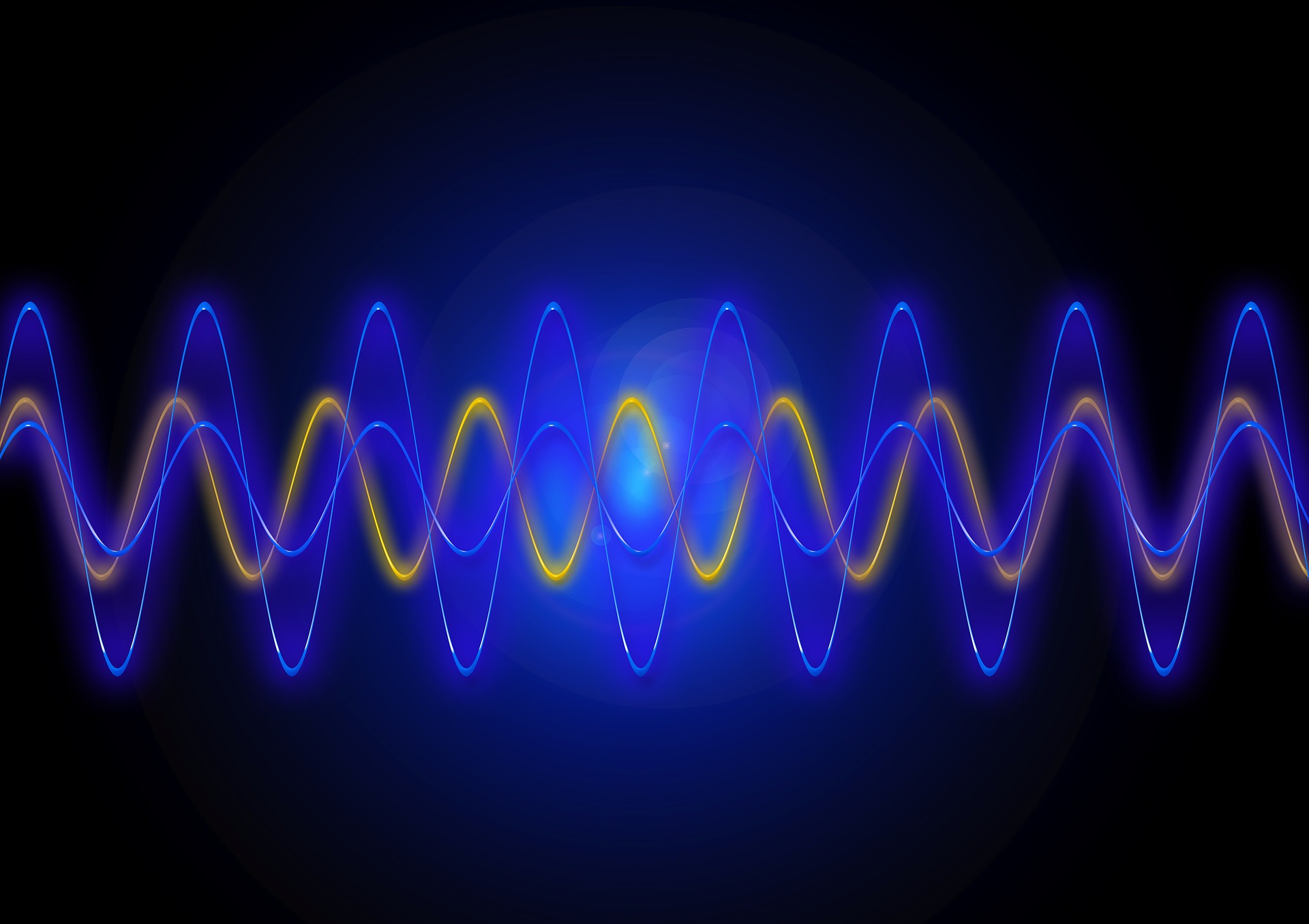
- Read more about Domain Generalized Few-Shot Image Classification Via Meta Regularization Network
- Log in to post comments
1435_poster.pdf

- Categories:
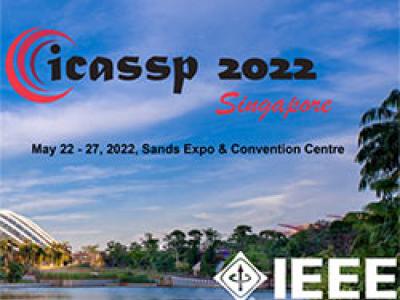
- Read more about Integration of Pre-trained Networks with Continuous Token Interface For End-to-End Spoken Language Understanding
- Log in to post comments
Most End-to-End (E2E) Spoken Language Understanding (SLU) networks leverage the pre-trained Automatic Speech Recognition (ASR) networks but still lack the capability to understand the semantics of utterances, crucial for the SLU task. To solve this, recently proposed studies use pre-trained Natural Language Understanding (NLU) networks. However, it is not trivial to fully utilize both pre-trained networks; many solutions were proposed, such as Knowledge Distillation (KD), cross-modal shared embedding, and network integration with Interface.
- Categories:
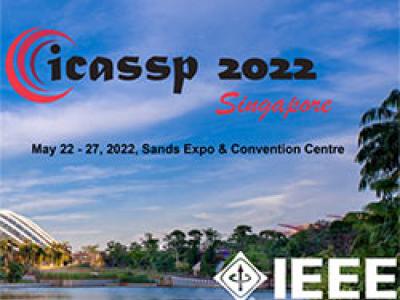
- Read more about OPENFEAT: Improving Speaker Identification by Open-set Few-shot Embedding Adaptation with Transformer
- Log in to post comments
Household speaker identification with few enrollment utterances is an important yet challenging problem, especially when household members share similar voice characteristics and room acoustics. A common embedding space learned from a large number of speakers is not universally applicable for the optimal identification of every speaker in a household.
- Categories:
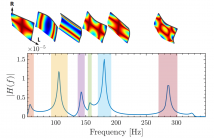
- Read more about On the Prediction of the Frequency Response of a Wooden Plate from its Mechanical Parameters
- Log in to post comments
Inspired by deep learning applications in structural mechanics, we focus on how to train two predictors to model the relation between the vibrational response of a prescribed point of a wooden plate and its material properties. In particular, the eigenfrequencies of the plate are estimated via multilinear regression, whereas their amplitude is predicted by a feedforward neural network.
- Categories: