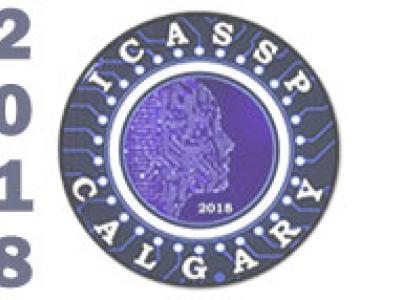
- Read more about Fast Projection onto the $\ell_{\infty,1}$-Mixed Norm Ball using Steffensen Root Search
- Log in to post comments
- Categories:
- Read more about MULTIPLE SOURCES IDENTIFICATION IN NETWORKS WITH PARTIAL TIMESTAMPS
- Log in to post comments
Poster.pdf

- Categories:
- Read more about DICTIONARY LEARNING IN THE ANALYSIS SPARSE REPRESENTATION WITH OPTIMIZATION ON STIEFEL MANIFOLD
- Log in to post comments
globalsip.pdf

- Categories:
- Read more about ON THE CONVERGENCE OF CONSTRAINED PARTICLE FILTERS
- Log in to post comments
The power of particle filters in tracking the state of non-linear and non-Gaussian systems stems not only from their simple numerical implementation but also from their optimality and convergence properties. In particle filtering, the posterior distribution of the state is approximated by a discrete mass of samples, called particles, that stochastically evolve in time according to the dynamics of the model and the observations. Particle filters have been shown to converge almost surely toward the optimal filter as the number of particles increases.
- Categories:
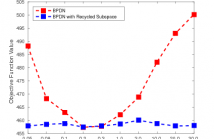
- Read more about ADMM Penalty Parameter Selection with Krylov Subspace Recycling Technique for Sparse Coding
- Log in to post comments
The alternating direction method of multipliers (ADMM) has been widely used for a very wide variety of imaging inverse problems. One of the disadvantages of this method, however, is the need to select an algorithm parameter, the penalty parameter, that has a significant effect on the rate of convergence of the algorithm. Although a number of heuristic methods have been proposed, as yet there is no general theory providing a good choice of this parameter for all problems.
- Categories:
- Read more about QUANTISATION EFFECTS IN PDMM: A FIRST STUDY FOR SYNCHRONOUS DISTRIBUTED AVERAGING
- Log in to post comments
Large-scale networks of computing units, often characterised by the absence of central control, have become commonplace in many applications. To facilitate data processing in these large-scale networks, distributed signal processing is required. The iterative behaviour of distributed processing algorithms combined with energy, computational power, and bandwidth limitations imposed by such networks, place tight constraints on the transmission capacities of the individual nodes.
- Categories:
- Read more about Performance Trade-off in an Adaptive IEEE 802.11ad Waveform Design for a Joint Automotive Radar and Communication System
- Log in to post comments
The IEEE 802.11ad waveform can be used for automotive radar by exploiting the Golay complementary sequences in the preamble of a frame. The performance of radar, however, is limited by the preamble structure. In this paper, we propose an adaptive preamble design that permits a trade-off between radar parameters’ estimation accuracy and communication rate. To quantify this trade-off, we propose a minimum mean square error (MMSE) metric based on rate distortion theory.
- Categories:
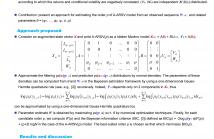
- Read more about Unsupervised learning of asymmetric high-order autoregressive stochastic volatility model
- Log in to post comments
We introduce a new estimation algorithm specifically designed for the latent high-order autoregressive models. It implements the concept of the filter-based maximum likelihood. Our approach is fully deterministic and is less computationally demanding than the traditional Monte Carlo Markov chain techniques. The simulation experiments confirm the interest of our approach.
- Categories:
- Read more about Energy Blowup for Truncated Stable LTI Systems
- Log in to post comments
In this paper we analyze the convergence behavior of a sampling based system approximation process, where the time variable is in the argument of the signal and not in the argument of the bandlimited impulse response. We consider the Paley-Wiener space $PW_\pi^2$ of bandlimited signals with finite energy and stable linear time-invariant (LTI) systems, and show that there are signals and systems such that the approximation process diverges in the $L^2$-norm, i.e., the norm of the signal space. We prove that the sets of signals and systems creating divergence are jointly spaceable, i.e., there exists an infinite dimensional closed subspace of $PW_\pi^2$ and an infinite dimensional closed subspace of the space of all stable LTI systems, such that the approximation process diverges for any non-zero pair of signal and system from these subspaces.
- Categories: