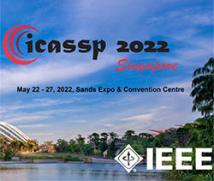
ICASSP 2022 - IEEE International Conference on Acoustics, Speech and Signal Processing is the world’s largest and most comprehensive technical conference focused on signal processing and its applications. The ICASSP 2022 conference will feature world-class presentations by internationally renowned speakers, cutting-edge session topics and provide a fantastic opportunity to network with like-minded professionals from around the world. Visit the website.
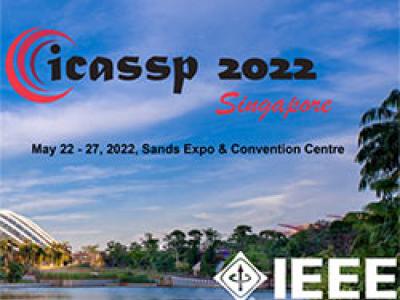
- Read more about DISENTANGLED SPEAKER EMBEDDING FOR ROBUST SPEAKER VERIFICATION
- Log in to post comments
Entanglement of speaker features and redundant features may lead to poor performance when evaluating speaker verification systems on an unseen domain. To address this issue, we propose an InfoMax domain separation and adaptation network (InfoMax–DSAN) to disentangle the domain-specific features and domain-invariant speaker features based on domain adaptation techniques. A frame-based mutual information neural estimator is proposed to maximize the mutual information between frame-level features and input acoustic features, which can help retain more useful information.
poster.pdf

- Categories:
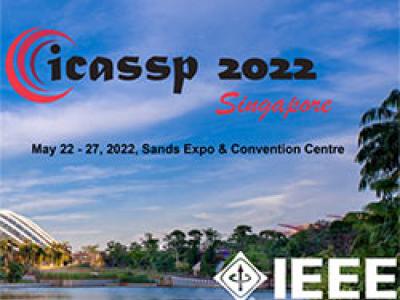
- Read more about Poster - KARASINGER: SCORE-FREE SINGING VOICE SYNTHESIS WITH VQ-VAE USING MEL-SPECTROGRAMS
- Log in to post comments
- Categories:
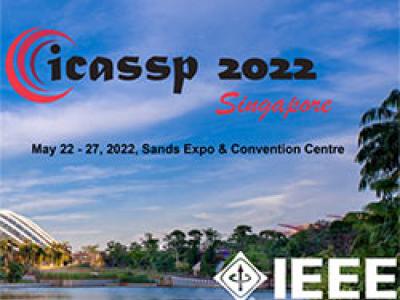
- Read more about Slides - KARASINGER: SCORE-FREE SINGING VOICE SYNTHESIS WITH VQ-VAE USING MEL-SPECTROGRAMS
- Log in to post comments
- Categories:
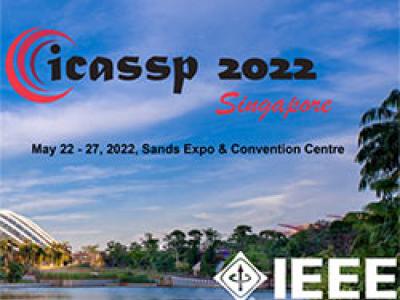
- Read more about DISENTANGLED SPEAKER EMBEDDING FOR ROBUST SPEAKER VERIFICATION
- Log in to post comments
Entanglement of speaker features and redundant features may lead to poor performance when evaluating speaker verification systems on an unseen domain. To address this issue, we propose an InfoMax domain separation and adaptation network (InfoMax–DSAN) to disentangle the domain-specific features and domain-invariant speaker features based on domain adaptation techniques. A frame-based mutual information neural estimator is proposed to maximize the mutual information between frame-level features and input acoustic features, which can help retain more useful information.
slides.pdf

- Categories:
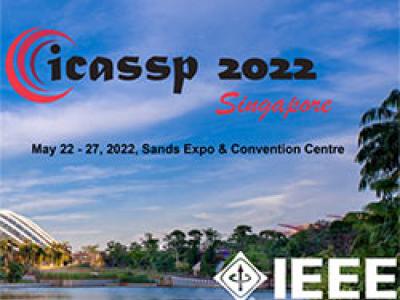
- Read more about A Generalized Kernel Risk Sensitive Loss for Robust Two-dimensional Singular Value Decomposition
- Log in to post comments
Two-dimensional singular value decomposition (2DSVD) is an important dimensionality reduction algorithm which has inherent advantage in preserving the structure of 2D images. However, 2DSVD algorithm is based on the squared error loss, which may exaggerate the projection errors in the presence of outliers. To solve this problem, we propose a generalized kernel risk sensitive loss for measuring the projection error in 2DSVD(GKRSL-2DSVD). The outliers information will be automatically eliminated during optimization.
- Categories:
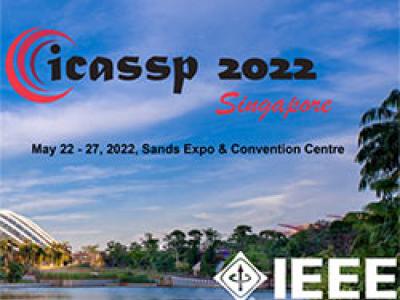
- Read more about Robust speaker verification using Population-based Data Augmentation Poster
- Log in to post comments
- Categories:
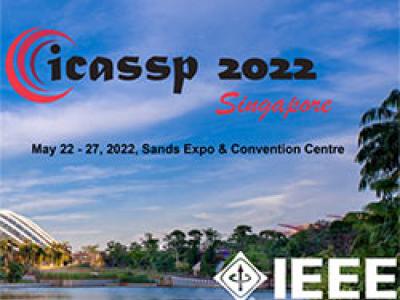
- Read more about Interactive Feature Fusion for End-to-End Noise-Robust Speech Recognition
- Log in to post comments
Speech enhancement (SE) aims to suppress the additive noise from noisy speech signals to improve the speech's perceptual quality and intelligibility. However, the over-suppression phenomenon in the enhanced speech might degrade the performance of downstream automatic speech recognition (ASR) task due to the missing latent information. To alleviate such problem, we propose an interactive feature fusion network (IFF-Net) for noise-robust speech recognition to learn complementary information from the enhanced feature and original noisy feature.
Hu_2783.pdf

- Categories:
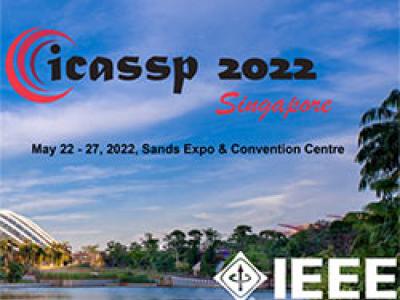
- Read more about Robust TDOA Source Localization Based on Lagrange Programming Neural Network
- Log in to post comments
This is the poster for the SPL paper: SAM-7.5: Robust TDOA Source Localization Based on Lagrange Programming Neural Network to be presented in the upcoming ICASSP 2022 conference in Singapore.
More details can be found at 10.1109/LSP.2021.3082035.
Thank you for your time.
- Categories:
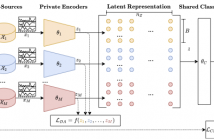
- Read more about DOMAIN-INVARIANT REPRESENTATION LEARNING FROM EEG WITH PRIVATE ENCODERS
- Log in to post comments
Deep learning based electroencephalography (EEG) signal processing methods are known to suffer from poor test-time generalization due to the changes in data distribution. This becomes a more challenging problem when privacy-preserving representation learning is of interest such as in clinical settings. To that end, we propose a multi-source learning architecture where we extract domain-invariant representations from dataset-specific private encoders.
- Categories:
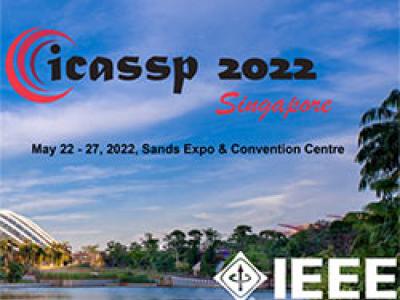
- Read more about Personalized PageRank Graph Attention Networks
- Log in to post comments
There has been a rising interest in graph neural networks (GNNs) for representation learning over the past few years. GNNs provide a general and efficient framework to learn from graph-structured data. However, GNNs typically only use the information of a very limited neighborhood for each node to avoid over-smoothing. A larger neighborhood would be desirable to provide the model with more information.
- Categories: