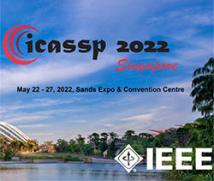
ICASSP 2022 - IEEE International Conference on Acoustics, Speech and Signal Processing is the world’s largest and most comprehensive technical conference focused on signal processing and its applications. The ICASSP 2022 conference will feature world-class presentations by internationally renowned speakers, cutting-edge session topics and provide a fantastic opportunity to network with like-minded professionals from around the world. Visit the website.
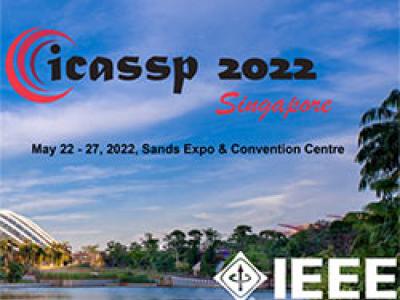
- Read more about ADAPTIVE ATTENTION GRAPH CAPSULE NETWORK
- Log in to post comments
From the perspective of the spatial domain, Graph Convolutional Network (GCN) is essentially a process of iteratively aggregating neighbor nodes. However, the existing GCNs using simple average or sum aggregation may neglect the characteristics of each node and the topology between nodes, resulting in a large amount of early-stage information lost during the graph convolution step. To tackle the above challenge, we innovatively propose an adaptive attention graph capsule network, named AA-GCN, for graph classification.
- Categories:
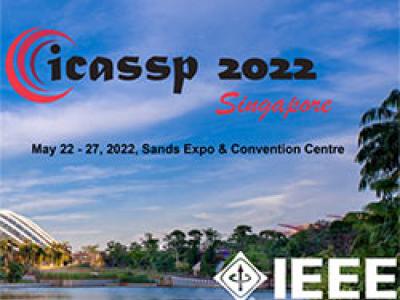
- Read more about ADAPTIVE ATTENTION GRAPH CAPSULE NETWORK
- Log in to post comments
From the perspective of the spatial domain, Graph Convolutional Network (GCN) is essentially a process of iteratively aggregating neighbor nodes. However, the existing GCNs using simple average or sum aggregation may neglect the characteristics of each node and the topology between nodes, resulting in a large amount of early-stage information lost during the graph convolution step. To tackle the above challenge, we innovatively propose an adaptive attention graph capsule network, named AA-GCN, for graph classification.
- Categories:
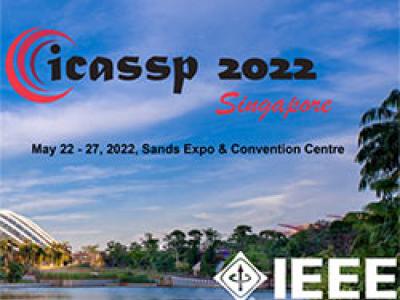
- Read more about Waveform Design for Wireless Power Transfer with Power Amplifier and Energy Harvester Non-Linearities
- Log in to post comments
Waveform optimization has recently been shown to be a key technique to boost the efficiency and range of far-field wireless power transfer (WPT). Current research has optimized transmit waveform adaptive to channel state information (CSI) and accounting for energy harvester (EH)’s non-linearity but under the assumption of linear high power amplifiers (HPA) at the transmitter. This paper proposes a channel-adaptive waveform design strategy that optimizes the transmitter's input waveform considering both HPA and EH non-linearities.
- Categories:
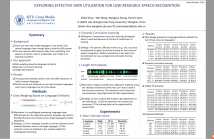
- Read more about EXPLORING EFFECTIVE DATA UTILIZATION FOR LOW-RESOURCE SPEECH RECOGNITION
- Log in to post comments
Automatic speech recognition (ASR) has suffered great performance degradation when facing low-resource languages with limited training data. In this work, we propose a series of training strategies to explore more effective data utilization for low-resource speech recognition. In low-resource scenarios, multilingual pretraining is of great help for the above purpose. We exploit relationships among different languages for better pretraining.
- Categories:
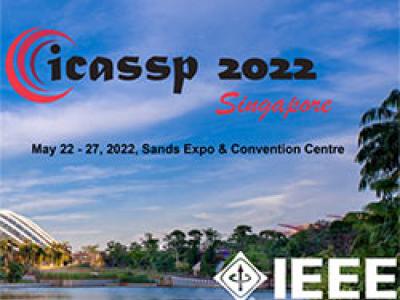
- Read more about Transfer Learning for Robust Low-Resource Children's Speech ASR with Transformers and Source-Filter Warping
- Log in to post comments
Automatic Speech Recognition (ASR) systems are known to exhibit difficulties when transcribing children's speech. This can mainly be attributed to the absence of large children’s speech corpora to train robust ASR models and the resulting domain mismatch when decoding children’s speech with systems trained on adult data. In this paper, we propose multiple enhancements to alleviate these issues. First, we propose a data augmentation technique based on the source-filter model of speech to close the domain gap between adult and children's speech.
- Categories:
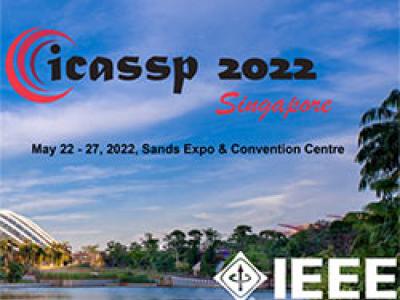
- Read more about Radar Target Detection Aided by Reconfigurable Intelligent Surfaces
- 1 comment
- Log in to post comments
- Categories:
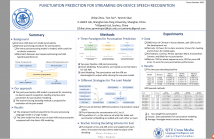
- Read more about Punctuation Prediction for Streaming On-Device Speech Recognition
- Log in to post comments
Punctuation prediction is essential for automatic speech recognition (ASR). Although many works have been proposed for punctuation prediction, the on-device scenarios are rarely discussed with an end-to-end ASR. The punctuation prediction task is often treated as a post-processing of ASR outputs, but the mismatch between natural language in training input and ASR hypotheses in testing is ignored. Besides, language models built with deep neural networks are too large for edge devices.
- Categories:
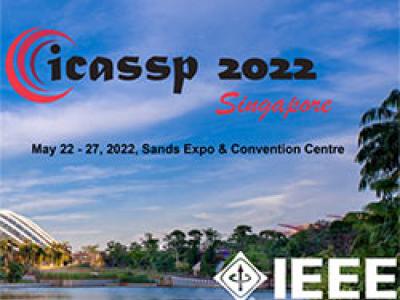
- Read more about RIS-aided monostatic MIMO Radar with co-located antenna
- 2 comments
- Log in to post comments
- Categories: