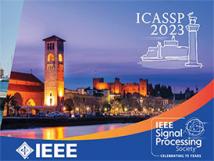
IEEE ICASSP 2023 - IEEE International Conference on Acoustics, Speech and Signal Processing is the world’s largest and most comprehensive technical conference focused on signal processing and its applications. The ICASSP 2023 conference will feature world-class presentations by internationally renowned speakers, cutting-edge session topics and provide a fantastic opportunity to network with like-minded professionals from around the world. Visit the website.
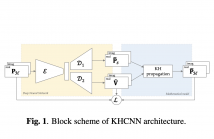
- Read more about PAPER - Grad-CAM-Inspired Interpretation of Nearfield Acoustic Holography using Physics-Informed Explainable Neural Network
- Log in to post comments
The interpretation and explanation of decision-making processes of neural networks are becoming a key factor in the deep learning field. Although several approaches have been presented for classification problems, the application to regression models needs to be further investigated. In this manuscript we propose a Grad-CAM-inspired approach for the visual explanation of neural network architecture for regression problems.
- Categories:
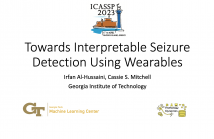
Seizure detection using machine learning is a critical problem for the timely intervention and management of epilepsy. We propose SeizFt, a robust seizure detection framework using EEG from a wearable device. It uses features paired with an ensemble of trees, thus enabling further interpretation of the model's results. The efficacy of the underlying augmentation and class-balancing strategy is also demonstrated. This study was performed for the Seizure Detection Challenge 2023, an ICASSP Grand Challenge.
SeizFt.pdf

- Categories:
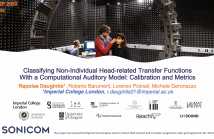
- Read more about Classifying Non-Individual Head-Related Transfer Functions with A Computational Auditory Model: Calibration And Metrics
- Log in to post comments
This study explores the use of a multi-feature Bayesian auditory sound localisation model to classify non-individual head-related transfer functions (HRTFs). Based on predicted sound localisation performance, these are grouped into ‘good’ and ‘bad’, and the ‘best’/‘worst’ is selected from each category. Firstly, we present a greedy algorithm for automated individual calibration of the model based on the individual sound localisation data.
- Categories:
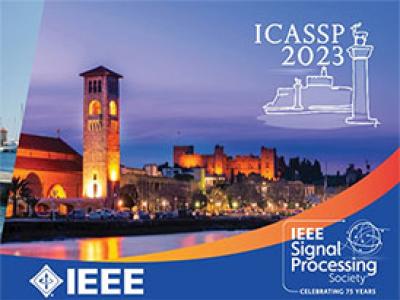
- Read more about Provably Convergent Plug & Play Linearized ADMM, applied to Deblurring Spatially Varying Kernels
- Log in to post comments
Plug & Play methods combine proximal algorithms with denoiser priors to solve inverse problems. These methods rely on the computability of the proximal operator of the data fidelity term. In this paper, we propose a Plug & Play framework based on linearized ADMM that allows us to bypass the computation of intractable proximal operators. We demonstrate the convergence of the algorithm and provide results on restoration tasks such as super-resolution and deblurring with non-uniform blur.
- Categories:
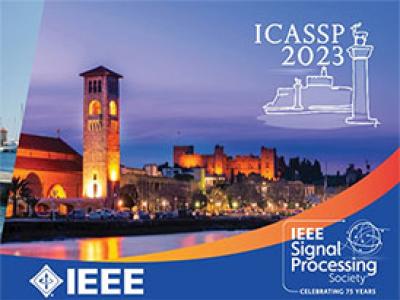
- Read more about A CLUSTERED FEDERATED LEARNING APPROACH FOR ESTIMATING THE QUALITY OF EXPERIENCE OF WEB USERS
- Log in to post comments
- Categories:
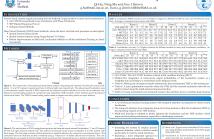
Despite there being clear evidence for attentional effects in biological spatial hearing, relatively few machine hearing systems exploit attention in binaural sound localisation. This paper addresses this issue by proposing a novel binaural machine hearing system with temporal attention for robust localisation of sound sources in noisy and reverberant conditions. A convolutional neural network is employed to extract noise-robust localisation features, which are similar to interaural phase difference, directly from phase spectra of the left and right ears for each frame.
- Categories:
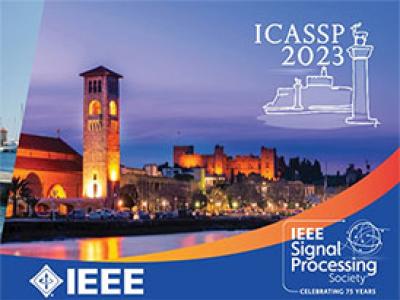
- Read more about Waveform design to improve the estimation of target parameters using the Fourier Transform method in a MIMO OFDM DFRC system
- Log in to post comments
Among the approaches used to jointly estimate the directions of arrival (DOAs) of K targets in a multiple-input multiple-output dual-function radar communication system, the method based on the identification of the K largest local maxima of the modulus of the Fourier transform (FT) of the signal received by the antennas has the advantage of having a low computational cost. However, the local maxima do not necessarily correspond to the values of interest. To avoid this problem, we present an operation mode making it possible to address the estimations of the DOAs separately.
- Categories:
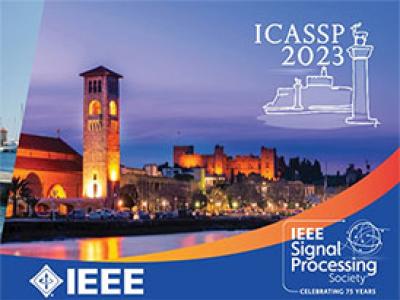
- Read more about Waveform design to improve the estimation of target parameters using the Fourier Transform method in a MIMO OFDM DFRC system
- Log in to post comments
Among the approaches used to jointly estimate the directions of arrival (DOAs) of K targets in a multiple-input multiple-output dual-function radar communication system, the method based on the identification of the K largest local maxima of the modulus of the Fourier transform (FT) of the signal received by the antennas has the advantage of having a low computational cost. However, the local maxima do not necessarily correspond to the values of interest. To avoid this problem, we present an operation mode making it possible to address the estimations of the DOAs separately.
- Categories:
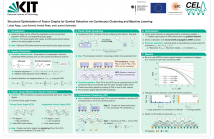
- Read more about Structural Optimization of Factor Graphs for Symbol Detection via Continuous Clustering and Machine Learning
- Log in to post comments
We propose a novel method to optimize the structure of factor graphs for graph-based inference. As an example inference task, we consider symbol detection on linear inter-symbol interference channels. The factor graph framework has the potential to yield low-complexity symbol detectors. However, the sum-product algorithm on cyclic factor graphs is suboptimal and its performance is highly sensitive to the underlying graph. Therefore, we optimize the structure of the underlying factor graphs in an end-to-end manner using machine learning.
poster.pdf

- Categories:
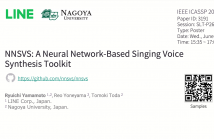
- Read more about NNSVS: A Neural Network-Based Singing Voice Synthesis Toolkit
- Log in to post comments
This paper describes the design of NNSVS, an open-source software for neural network-based singing voice synthesis research. NNSVS is inspired by Sinsy, an open-source pioneer in singing voice synthesis research, and provides many additional features such as multi-stream models, autoregressive fundamental frequency models, and neural vocoders. Furthermore, NNSVS provides extensive documentation and numerous scripts to build complete singing voice synthesis systems.
- Categories: