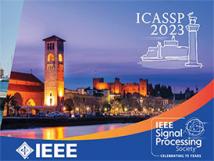
IEEE ICASSP 2023 - IEEE International Conference on Acoustics, Speech and Signal Processing is the world’s largest and most comprehensive technical conference focused on signal processing and its applications. The ICASSP 2023 conference will feature world-class presentations by internationally renowned speakers, cutting-edge session topics and provide a fantastic opportunity to network with like-minded professionals from around the world. Visit the website.
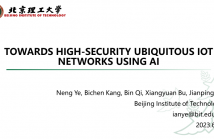
The on-going paradigm shift knocking on the door of future wireless communication system is ubiquitous Internet of Things (IoT), and the maturity of which will be hindered by the challenges related to security. Artificial intelligence (AI) is proficient in solving intractable optimization problems in a data-based way, which provides a new idea for network security and physical-layer guarantee. In this paper, we divide the ubiquitous IoT networks into cyberspace and electromagnetic space, and identify the threat models.
poster.pdf

- Categories:
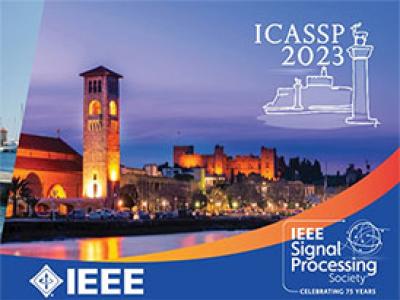
- Read more about ViTASD: Robust Vision Transformer Baselines for Autism Spectrum Disorder Facial Diagnosis
- Log in to post comments
Autism spectrum disorder (ASD) is a lifelong neurodevelopmental disorder with very high prevalence around the world. Research progress in the field of ASD facial analysis in pediatric patients has been hindered due to a lack of well-established baselines. In this paper, we propose the use of the Vision Transformer (ViT) for the computational analysis of pediatric ASD. The presented model, known as ViTASD, distills knowledge from large facial expression datasets and offers model structure transferability.
370_Poster.pdf

- Categories:
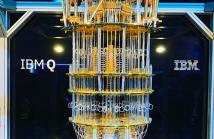
- Read more about SIGNAL PROCESSING AND QUANTUM STATE TOMOGRAPHY ON NOISY DEVICES
- Log in to post comments
Quantum State Tomography (QST) is a fundamental tool for quantum signal processing. However, in real noisy quantum devices construction of the state's density matrix via QST can utilize a large amount of resources. Here, we discuss some signal processing techniques that are currently applied to this resource issue, and implement on current quantum chips a modification that can assist in reducing resources. An application of QST to quantum entanglement distillation is provided for further insight.
- Categories:
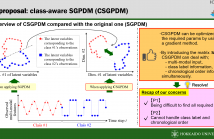
- Read more about Class-aware Shared Gaussian Process Dynamic Model
- Log in to post comments
A new method of Gaussian process dynamic model (GPDM), named class-aware shared GPDM (CSGPDM), is presented in this paper. One of the most difference between our CSGPDM and existing GPDM is considering class information which helps to build the class label-based latent space being effective for the following class-related tasks. In terms of representation learning, CSGPDM is optimized by considering not only a non-linear relationship but also time-series relation and discriminative information of each class label.
- Categories:
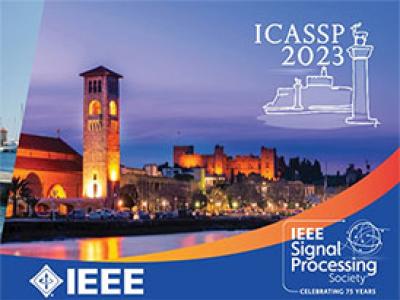
- Read more about A study of audio mixing methods for piano transcription in violin-piano ensembles
- Log in to post comments
- Categories:
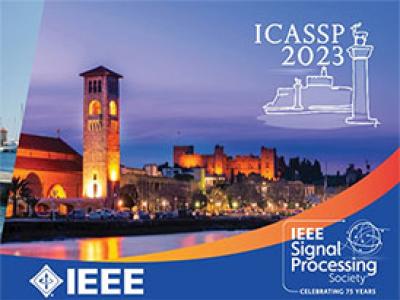
- Read more about SPEECH MODELING WITH A HIERARCHICAL TRANSFORMER DYNAMICAL VAE
- Log in to post comments
The dynamical variational autoencoders (DVAEs) are a family of latent-variable deep generative models that extends the VAE to model a sequence of observed data and a corresponding sequence of latent vectors. In almost all the DVAEs of the literature, the temporal dependencies within each sequence and across the two sequences are modeled with recurrent neural networks.
- Categories:
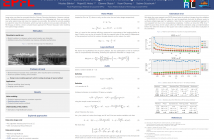
- Read more about PoGaIN: Poisson-Gaussian Image Noise Modeling from Paired Samples
- Log in to post comments
Image noise can often be accurately fitted to a Poisson-Gaussian distribution. However, estimating the distribution parameters from a noisy image only is a challenging task. Here, we study the case when paired noisy and noise-free samples are accessible. No method is currently available to exploit the noise-free information, which may help to achieve more accurate estimations. To fill this gap, we derive a novel, cumulant-based, approach for Poisson-Gaussian noise modeling from paired image samples.
- Categories:
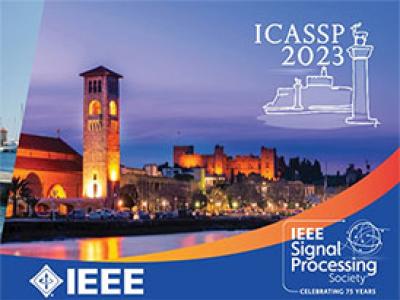
- Read more about IMAGE SEGMENTATION FOR IMPROVED LOSSLESS SCREEN CONTENT COMPRESSION
- Log in to post comments
In recent years, it has been found that screen content images (SCI) can be effectively compressed based on appropriate probability modelling and suitable entropy coding methods such as arithmetic coding. The key objective is determining the best probability distribution for each pixel position. This strategy works particularly well for images with synthetic (textual) content. However, usually screen content images not only consist of synthetic but also pictorial (natural) regions. These images require diverse models of probability distributions to be optimally compressed.
- Categories:
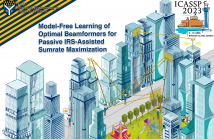
- Read more about Model-Free Learning of Optimal Beamformers for Passive IRS-Assisted Sumrate Maximization
- Log in to post comments
Although Intelligent Reflective Surfaces (IRSs) are a cost-effective technology promising high spectral efficiency in future wireless networks, obtaining optimal IRS beamformers is a challenging problem with several practical limitations. Assuming fully-passive, sensing- free IRS operation, we introduce a new data-driven Zeroth-order Stochastic Gradient Ascent (ZoSGA) algorithm for sumrate optimization in an IRS-aided downlink setting.
ZoSGA_Poster.pdf

- Categories:
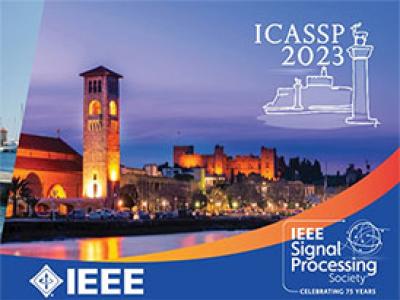
- Read more about A study on the invariance in security whatever the dimension of images for the steganalysis by deep-learning
- Log in to post comments
In this paper, we study the performance invariance of convolutional neural networks when confronted with variable image sizes in the context of a more ”wild steganalysis”. First, we propose two algorithms and definitions for a fine experimental protocol with datasets owning ”similar difficulty” and ”similar security”. The ”smart crop 2” algorithm allows the introduction of the Nearly Nested Image Datasets (NNID) that ensure ”a similar difficulty” between various datasets, and a dichotomous research algorithm allows a ”similar security”.
- Categories: