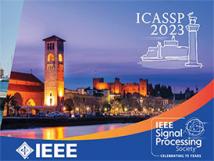
IEEE ICASSP 2023 - IEEE International Conference on Acoustics, Speech and Signal Processing is the world’s largest and most comprehensive technical conference focused on signal processing and its applications. The ICASSP 2023 conference will feature world-class presentations by internationally renowned speakers, cutting-edge session topics and provide a fantastic opportunity to network with like-minded professionals from around the world. Visit the website.
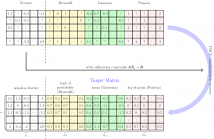
- Read more about Transductive Matrix Completion with Calibration for Multi-Task Learning
- Log in to post comments
Multi-task learning has attracted much attention due to growing multi-purpose research with multiple related data sources. Moreover, transduction with matrix completion is a useful method in multi-label learning. In this paper, we propose a transductive matrix completion algorithm that incorporates a calibration constraint for the features under the multi-task learning framework. The proposed algorithm recovers the incomplete feature matrix and target matrix simultaneously. Fortunately, the calibration information improves the completion results.
- Categories:
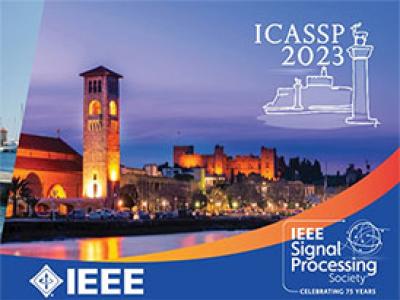
Point cloud completion aims to accurately estimate complete point clouds from partial observations. Existing methods often directly infer the missing points from the partial shape, but they suffer from limited structural information. To address this, we propose the Bilateral Coarse-to-Fine Network (BCFNet), which leverages 2D images as guidance to compensate for structural information loss. Our method introduces a multi-level codeword skip-connection to estimate structural details.
- Categories:
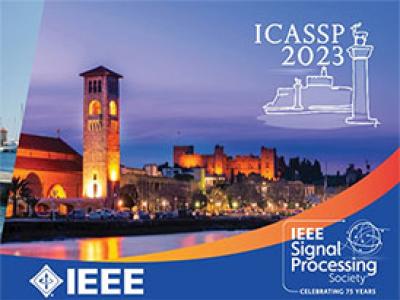
- Read more about MEET: A Monte Carlo Exploration-Exploitation Trade-off for Buffer Sampling
- Log in to post comments
Data selection is essential for any data-based optimization technique, such as Reinforcement Learning. State-of-the-art sampling strategies for the experience replay buffer improve the performance of the Reinforcement Learning agent. However, they do not incorporate uncertainty in the Q-Value estimation. Consequently, they cannot adapt the sampling strategies, including exploration and exploitation of transitions, to the complexity of the task.
MEET_Poster.pdf

icassp_2023 (7).pdf

- Categories:
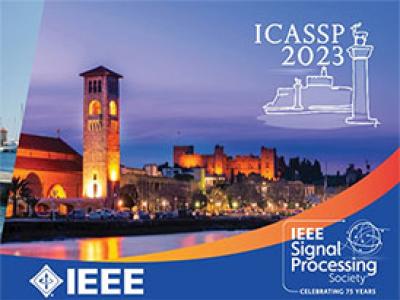
- Read more about DYNAMIC ALIGNMENT MASK CTC: IMPROVED MASK CTC WITH ALIGNED CROSS ENTROPY
- Log in to post comments
- Categories:
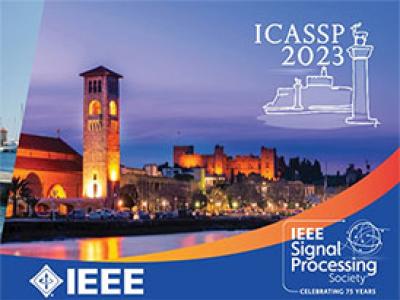
- Read more about QI-TTS: QUESTIONING INTONATION CONTROL FOR EMOTIONAL SPEECH SYNTHESIS
- Log in to post comments
- Categories:
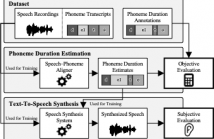
- Read more about Evaluating Speech–Phoneme Alignment and Its Impact on Neural Text-To-Speech Synthesis
- Log in to post comments
In recent years, the quality of text-to-speech (TTS) synthesis vastly improved due to deep-learning techniques, with parallel architectures, in particular, providing excellent synthesis quality at fast inference. Training these models usually requires speech recordings, corresponding phoneme-level transcripts, and the temporal alignment of each phoneme to the utterances. Since manually creating such fine-grained alignments requires expert knowledge and is time-consuming, it is common practice to estimate them using automatic speech–phoneme alignment methods.
- Categories:
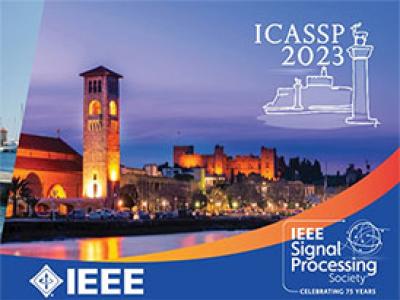
- Read more about FEDEEG__FEDERATED_EEG_DECODING_VIA_INTER_SUBJECT_STRUCTURE_MATCHING
- Log in to post comments
With sufficient centralized training data coming from multiple subjects, deep learning methods have achieved powerful EEG decoding performance. However, sending each individuals’ EEG data directly to a centralized server might cause privacy leakage. To overcome this issue, we present an inter-subject structure matching-based federated EEG decoding (FedEEG) framework. First, we introduce a center loss to each client (subject), which can learn multiple virtual class centers by averaging the corresponding class-specific EEG features.
- Categories:
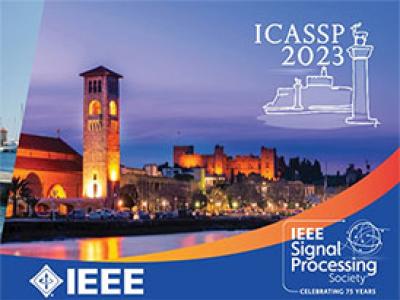
- Categories:
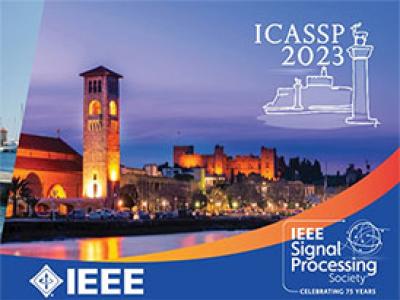
- Categories:
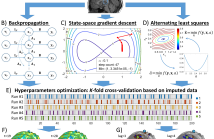
- Read more about DYNAMIC SOURCE LOCALIZATION AND FUNCTIONAL CONNECTIVITY ESTIMATION WITH STATE-SPACE MODELS: PRELIMINARY FEASIBILITY ANALYSIS - Preprint and Code
- Log in to post comments
Dynamic imaging of source and functional connectivity (FC) using electroencephalographic (EEG) signals is essential for understanding the brain and cognition with sufficiently affordable technology to be widely applicable for studying changes associated with healthy ageing and the progression of neuropathology. We present an application for group analysis of recently developed state-space models and algorithms for simultaneously estimating the large-scale EEG inverse and FC problems.
- Categories: