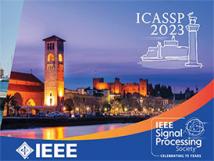
IEEE ICASSP 2023 - IEEE International Conference on Acoustics, Speech and Signal Processing is the world’s largest and most comprehensive technical conference focused on signal processing and its applications. The ICASSP 2023 conference will feature world-class presentations by internationally renowned speakers, cutting-edge session topics and provide a fantastic opportunity to network with like-minded professionals from around the world. Visit the website.
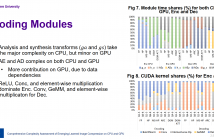
- Read more about Comprehensive Complexity Assessment of Emerging Learned Image Compression on CPU And GPU
- Log in to post comments
Learned Compression (LC) is the emerging technology for compressing image and video content, using deep neural networks. Despite being new, LC methods have already gained a compression efficiency comparable to state-of-the-art image compression, such as HEVC or even VVC. However, the existing solutions often require a huge computational complexity, which discourages their adoption in international standards or products.
- Categories:
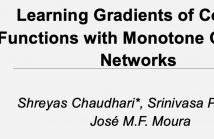
- Read more about Learning Gradients of Convex Functions with Monotone Gradient Networks
- Log in to post comments
While much effort has been devoted to deriving and analyzing effective convex formulations of signal processing problems, the gradients of convex functions also have critical applications ranging from gradient-based optimization to optimal transport. Recent works have explored data-driven methods for learning convex objective functions, but learning their monotone gradients is seldom studied. In this work, we propose C-MGN and M-MGN, two monotone gradient neural network architectures for directly learning the gradients of convex functions.
- Categories:
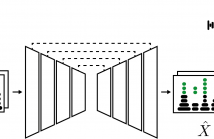
- Categories:
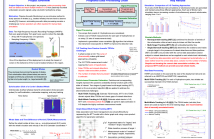
- Read more about PASSIVE ACOUSTIC TRACKING OF WHALES IN 3-D
- Log in to post comments
Passive acoustic monitoring (PAM) is a nonintrusive approach to studying behaviors of vocalizing marine organisms underwater that otherwise would remain unexplored. In this paper, we propose a data processing chain that can detect and track multiple whales in 3-D from passively recorded underwater acoustic signals. In particular, time-difference-of-arrival (TDOA) measurements of echolocation clicks are extracted from a volumetric hydrophone array's acoustic data by using a noise-whitening cross-correlation.
- Categories:
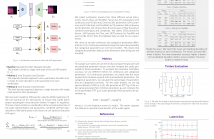
- Read more about Exploring Approaches to Multi-Task Automatic Synthesizer Programming
- Log in to post comments
Automatic Synthesizer Programming is the task of transforming an audio signal that was generated from a virtual instrument, into the parameters of a sound synthesizer that would generate this signal. In the past, this could only be done for one virtual instrument. In this paper, we expand the current literature by exploring approaches to automatic synthesizer programming for multiple virtual instruments. Two different approaches to multi-task automatic synthesizer programming are presented. We find that the joint-decoder approach performs best.
- Categories:
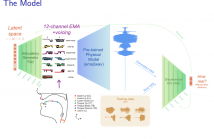
- Read more about Articulation GAN: Unsupervised Modeling of Articulatory Learning
- Log in to post comments
Generative deep neural networks are widely used for speech synthesis, but most existing models directly generate waveforms or spectral outputs. Humans, however, produce speech by controlling articulators, which results in the production of speech sounds through physical properties of sound propagation. We introduce the Articulatory Generator to the Generative Adversarial Network paradigm, a new unsupervised generative model of speech production/synthesis.
- Categories:
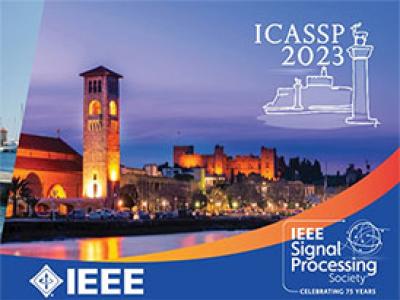
- Read more about Multi-dimensional Signal Recovery Using Low-rank Deconvolution
- Log in to post comments
In this work we present Low-rank Deconvolution, a powerful framework for low-level feature-map learning for efficient signal representation with application to signal recovery. Its formulation in multi-linear algebra inherits properties from convolutional sparse coding and low-rank approximation methods as in this setting signals are decomposed in a set of filters convolved with a set of low-rank tensors. We show its advantages by learning compressed video representations and solving image in-painting problems.
- Categories:
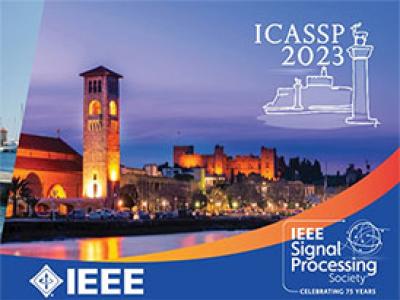
- Read more about MMCosine: Multi-Modal Cosine Loss Towards Balanced Audio-Visual Fine-Grained Learning
- Log in to post comments
Audio-visual learning helps to comprehensively understand the world by fusing practical information from multiple modalities. However, recent studies show that the imbalanced optimization of uni-modal encoders in a joint-learning model is a bottleneck to enhancing the model`s performance. We further find that the up-to-date imbalance-mitigating methods fail on some audio-visual fine-grained tasks, which have a higher demand for distinguishable feature distribution.
- Categories:
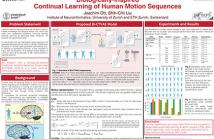
- Read more about Biologically-Inspired Continual Learning of Human Motion Sequences
- Log in to post comments
This work proposes a model for continual learning on tasks involving temporal sequences, specifically, human motions. It improves on a recently proposed brain-inspired replay model (BI-R) by building a biologically-inspired conditional temporal variational autoencoder (BI-CTVAE), which instantiates a latent mixture-of-Gaussians for class representation. We investigate a novel continual-learning-to-generate (CL2Gen) scenario where the model generates motion sequences of different classes. The generative accuracy of the model is tested over a set of tasks.
- Categories:
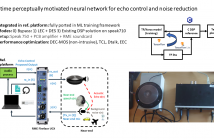
- Read more about Real-time perceptually motivated neural network for echo control and noise reduction
- Log in to post comments
Echo and background noise are the major obstacles in today’s user sound experience for devices like a speakerphone or video bar. We propose real-time perceptually motivated neural network-based echo control and noise reduction. The demonstrated method relies on a linear acoustic echo canceller (LAEC) combined with a neural network as a post-filter which incorporates perceptual mapping in both feature representation and loss function. The proposed method relies on mic and far-end signals for the LAEC stage, while the LAEC output, mic and echo estimate are inputs to the post-filter.
- Categories: