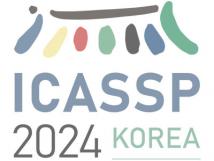
IEEE ICASSP 2024 - IEEE International Conference on Acoustics, Speech and Signal Processing (ICASSP) is the world’s largest and most comprehensive technical conference focused on signal processing and its applications. The IEEE ICASSP 2024 conference will feature world-class presentations by internationally renowned speakers, cutting-edge session topics and provide a fantastic opportunity to network with like-minded professionals from around the world. Visit the website.
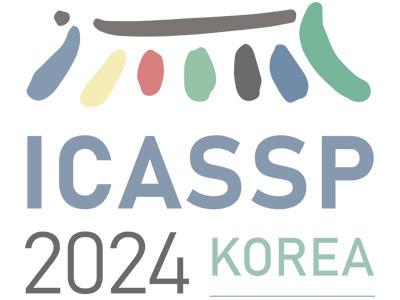
- Read more about EEG-BASED FAST AUDITORY ATTENTION DETECTION IN REAL-LIFE SCENARIOS USING TIME-FREQUENCY ATTENTION MECHANISM
- 1 comment
- Log in to post comments
Auditory attention detection (AAD) based on electroencephalogram (EEG) helps recognize the target speaker in a cocktail party scenario, advancing auditory brain-computer interface development. Previous EEG studies on AAD were largely based on data collected in laboratory settings. In this study, we investigated the AAD with EEG data collected when subjects were walking and sitting in real-life scenarios. To improve the detection accuracy, we proposed the time-frequency attention mechanism to the convolution neural network on EEG data.
- Categories:
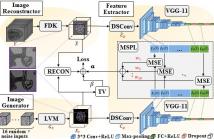
- Read more about STABLE OPTIMIZATION FOR LARGE VISION MODEL BASED DEEP IMAGE PRIOR IN CONE-BEAM CT RECONSTRUCTION
- Log in to post comments
Large Vision Model (LVM) has recently demonstrated great potential for medical imaging tasks, potentially enabling image enhancement for sparse-view Cone-Beam Computed Tomography (CBCT), despite requiring a substantial amount of data for training. Meanwhile, Deep Image Prior (DIP) effectively guides an untrained neural network to generate high-quality CBCT images without any training data. How- ever, the original DIP method relies on a well-defined forward model and a large-capacity backbone network, which is no- toriously difficult to converge.
- Categories:
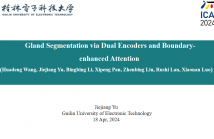
- Read more about GLAND SEGMENTATION VIA DUAL ENCODERS AND BOUNDARY-ENHANCED ATTENTION
- Log in to post comments
Accurate and automated gland segmentation on pathological images can assist pathologists in diagnosing the malignancy of colorectal adenocarcinoma. However, due to various gland shapes, severe deformation of malignant glands, and overlapping adhesions between glands. Gland segmentation has always been very challenging. To address these problems, we propose a DEA model. This model consists of two branches: the backbone encoding and decoding network and the local semantic extraction network.
- Categories:
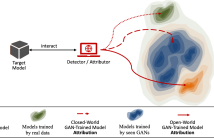
- Read more about Detection and Attribution of Models Trained on Generated Data
- Log in to post comments
Generative Adversarial Networks (GANs) have become widely used in model training, as they can improve performance and/or protect sensitive information by generating data. However, this also raises potential risks, as malicious GANs may compromise or sabotage models by poisoning their training data. Therefore, it is important to verify the origin of a model’s training data for accountability purposes. In this work, we take the first step in the forensic analysis of models trained on GAN-generated data. Specifically, we first detect whether a model is trained on GAN-generated or real data.
- Categories:
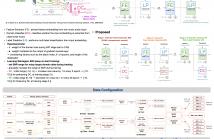
- Read more about MUSIC AUTO-TAGGING WITH ROBUST MUSIC REPRESENTATION LEARNED VIA DOMAIN ADVERSARIAL TRAINING
- Log in to post comments
Music auto-tagging is crucial for enhancing music discovery and recommendation. Existing models in Music Information Retrieval (MIR) struggle with real-world noise such as environmental and speech sounds in multimedia content. This study proposes a method inspired by speech-related tasks to enhance music auto-tagging performance in noisy settings. The approach integrates Domain Adversarial Training (DAT) into the music domain, enabling robust music representations that withstand noise.
- Categories:
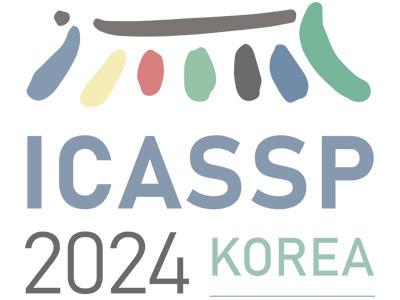
- Read more about VOCAL FOLD DYNAMICS FOR AUTOMATIC DETECTION OF AMYOTROPHIC LATERAL SCLEROSIS FROM VOICE
- Log in to post comments
Amyotrophic Lateral Sclerosis (ALS) is a neurodegenerative disease that affects motor neurons and causes speech and respiratory dysfunctions. Current diagnostic methods are com- plicated, thus motivating the development of an efficient and objective diagnostic aid. We hypothesize that analyses of features capturing the essential characteristics of the biomechanical process of voice production can distinguish ALS patients from non-ALS controls. In this paper, we represent voices with algorithmically estimated vocal fold dynamics from physical models of phonation.
- Categories:
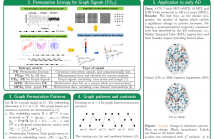
- Read more about Poster for "Graph-based permutation patterns for the analysis of task-related fMRI signals on DTI networks in mild cognitive impairment"
- Log in to post comments
Permutation Entropy (PE) is a powerful nonlinear analysis technique for univariate time series. Recently, Permutation Entropy for Graph signals (PE_G) has been proposed to extend PE to data residing on irregular domains. However, PE_G is limited as it provides a single value to characterise a whole graph signal. Here, we introduce a novel approach to evaluate graph signals at the vertex level: graph-based permutation patterns. Synthetic datasets show the efficacy of our method.
- Categories:
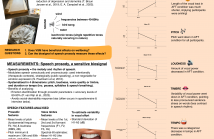
- Read more about ASSESSING VIBROACOUSTIC SOUND MASSAGE THROUGH THE BIOSIGNAL OF HUMAN SPEECH: EVIDENCE OF IMPROVED WELLBEING
- Log in to post comments
Stress has notorious and debilitating effects on individuals and entire industries alike, with instances of stress continuing to rise post-pandemic. We investigate here (1) if the new technology of Vibroacoustic Sound Massage (VSM) has beneficial effects on user wellbeing and (2) if we can measure these effects based on the biosignal of speech prosody. Forty participants read a text before and after VSM treatment (45 min). The 80 readings were subjected to a multi-parameteric acoustic-prosodic analysis.
- Categories:
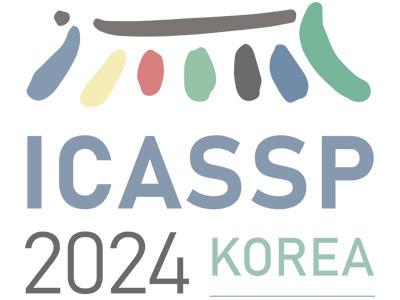
- Read more about GLMB 3D SPEAKER TRACKING WITH VIDEO-ASSISTED MULTI-CHANNEL AUDIO OPTIMIZATION FUNCTIONS
- Log in to post comments
Speaker tracking plays a significant role in numerous real-world human robot interaction (HRI) applications. In recent years, there has been a growing interest in utilizing multi-sensory information, such as complementary audio and visual signals, to address the challenges of speaker tracking. Despite the promising results, existing approaches still encounter difficulties in accurately determining the speaker’s true location, particularly in adverse conditions such as
- Categories:
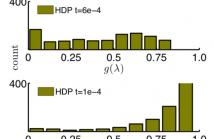
- Read more about High-order Tensor Pooling with Attention for Action Recognition
- Log in to post comments
We aim at capturing high-order statistics of feature vectors formed by a neural network, and propose end-to-end second- and higher-order pooling to form a tensor descriptor. Tensor descriptors require a robust similarity measure due to low numbers of aggregated vectors and the burstiness phenomenon, when a given feature appears more/less frequently than statistically expected. The Heat Diffusion Process (HDP) on a graph Laplacian is closely related to the Eigenvalue Power Normalization (EPN) of the covariance/auto-correlation matrix, whose inverse forms a loopy graph Laplacian.
- Categories: