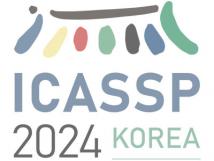
IEEE ICASSP 2024 - IEEE International Conference on Acoustics, Speech and Signal Processing (ICASSP) is the world’s largest and most comprehensive technical conference focused on signal processing and its applications. The IEEE ICASSP 2024 conference will feature world-class presentations by internationally renowned speakers, cutting-edge session topics and provide a fantastic opportunity to network with like-minded professionals from around the world. Visit the website.
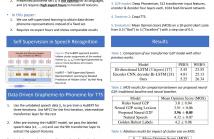
- Read more about DATA DRIVEN GRAPHEME-TO-PHONEME REPRESENTATIONS FOR A LEXICON-FREE TEXT-TO-SPEECH
- Log in to post comments
Grapheme-to-Phoneme (G2P) is an essential first step in any modern, high-quality Text-to-Speech (TTS) system. Most of the current G2P systems rely on carefully hand-crafted lexicons developed by experts. This poses a two-fold problem. Firstly, the lexicons are generated using a fixed phoneme set, usually, ARPABET or IPA, which might not be the most optimal way to represent phonemes for all languages. Secondly, the man-hours required to produce such an expert lexicon are very high.
- Categories:
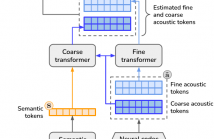
- Read more about Speaker anonymization using neural audio codec language models
- Log in to post comments
The vast majority of approaches to speaker anonymization involve the extraction of fundamental frequency estimates, linguistic features and a speaker embedding which is perturbed to obfuscate the speaker identity before an anonymized speech waveform is resynthesized using a vocoder.
Recent work has shown that x-vector transformations are difficult to control consistently: other sources of speaker information contained within fundamental frequency and linguistic features are re-entangled upon vocoding, meaning that anonymized speech signals still contain speaker information.
- Categories:
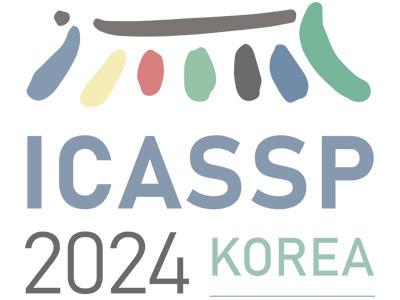
- Read more about HAZY REMOTE SENSING IMAGES SEMANTIC SEGMENTATION FOR WEAKLY ANNOTATION BASED ON SALIENCY-AWARE ALIGNMENT STRATEGY
- 1 comment
- Log in to post comments
The technique of semantic segmentation (SS) holds significant importance in the domain of remote sensing image (RSI) processing. The current research primarily encompasses two problems: 1) RSIs are easily affected by clouds and haze; 2) SS based on strong annotation requires vast human and time costs. In this paper, we propose a weakly supervised semantic segmentation (WSSS) method for hazy RSIs based on saliency-aware alignment strategy. Firstly, we design alignment network (AN) and target network (TN) with the same structure.
XU-poster.pdf

- Categories:
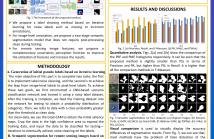
- Read more about SEMANTIC SEGMENTATION FOR MULTI-SCENE REMOTE SENSING IMAGES WITH NOISY LABELS BASED ON UNCERTAINTY PERCEPTION
- Log in to post comments
As the annotation of remote sensing images requires domain expertise, it is difficult to construct a large-scale and accurate annotated dataset. Image-level annotation data learning has become a research hotspot. In addition, due to the difficulty in avoiding mislabeling, label noise cleaning is also a concern. In this paper, a semantic segmentation method for remote sensing images based on uncertainty perception with noisy labels is proposed. The main contributions are three-fold.
- Categories:
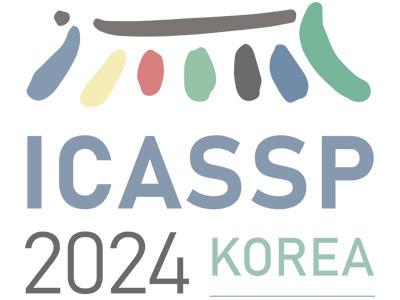
- Read more about Enhancing Generalization in Medical Visual Question Answering Tasks via Gradient-Guided Model Perturbation
- Log in to post comments
Leveraging pre-trained visual language models has become a widely adopted approach for improving performance in downstream visual question answering (VQA) applications. However, in the specialized field of medical VQA, the scarcity of available data poses a significant barrier to achieving reliable model generalization. Numerous methods have been proposed to enhance model generalization, addressing the issue from data-centric and model-centric perspectives.
poster_lhy.pdf

- Categories:
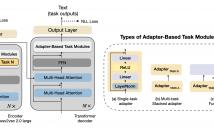
- Read more about An Adapter-Based Unified Model for Multiple Spoken Language Processing Tasks
- Log in to post comments
Self-supervised learning models have revolutionized the field of speech processing. However, the process of fine-tuning these models on downstream tasks requires substantial computational resources, particularly when dealing with multiple speech-processing tasks. In this paper, we explore the potential of adapter-based fine-tuning in developing a unified model capable of effectively handling multiple spoken language processing tasks. The tasks we investigate are Automatic Speech Recognition, Phoneme Recognition, Intent Classification, Slot Filling, and Spoken Emotion Recognition.
ICASSP 2024 - 3826.pdf

suresh_3826.pdf

- Categories:
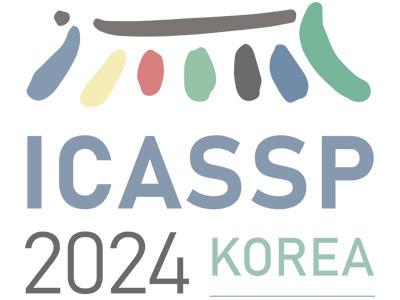
- Read more about CONTEXTUAL BIASING OF NAMED-ENTITIES WITH LARGE LANGUAGE MODELS
- Log in to post comments
We explore contextual biasing with Large Language Models (LLMs) to enhance Automatic Speech Recognition (ASR) in second-pass rescoring. Our approach introduces the utilization of prompts for LLMs during rescoring without the need for fine-tuning. These prompts incorporate a biasing list and a set of few-shot examples, serving as supplementary sources of information when evaluating the hypothesis score. Furthermore, we introduce multi-task training for LLMs to predict entity class and the subsequent token.
- Categories:
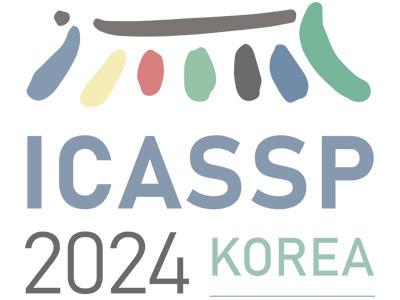
- Read more about RVAE-EM: Generative Speech Dereverberation Based On Recurrent Variational Auto-Encoder And Convolutive Transfer Function
- Log in to post comments
In indoor scenes, reverberation is a crucial factor in degrading the perceived quality and intelligibility of speech. In this work, we propose a generative dereverberation method. Our approach is based on a probabilistic model utilizing a recurrent variational auto-encoder (RVAE) network and the convolutive transfer function (CTF) approximation. Different from most previous approaches, the output of our RVAE serves as the prior of the clean speech.
posterA0.pdf

- Categories:
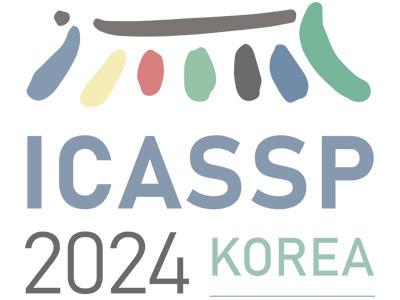
- Read more about S2E: Towards an End-to-End Entity Resolution Solution from Acoustic Signal
- Log in to post comments
Traditional cascading Entity Resolution (ER) pipeline suffers from propagated errors from upstream tasks. We address this is-sue by formulating a new end-to-end (E2E) ER problem, Signal-to-Entity (S2E), resolving query entity mentions to actionable entities in textual catalogs directly from audio queries instead of audio transcriptions in raw or parsed format. Additionally, we extend the E2E Spoken Language Understanding framework by introducing a novel dimension to ER research.
- Categories:
- Read more about TOWARDS FASTER END-TO-END DATA TRANSMISSION OVER VOICE CHANNELS
- Log in to post comments
As new technologies spread, phone fraud crimes have become master strategies to steal money and personal identities. Inspired by website authentication, we propose an end-to-end data modem over voice channels that can transmit the caller’s digital certificate to the callee for verification. Without assistance from telephony providers, it is difficult to carry useful information over voice channels. For example, voice activity detection may quickly classify the encoded signals as nonspeech signals and reject the input waveform.
会议海报-终稿.pdf

- Categories: