- Image/Video Storage, Retrieval
- Image/Video Processing
- Image/Video Coding
- Image Scanning, Display, and Printing
- Image Formation
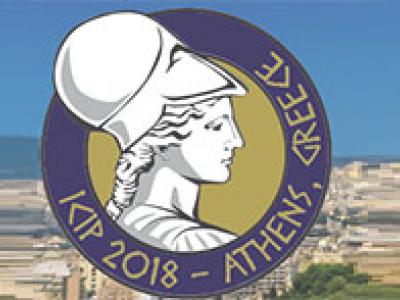
- Read more about A PRACTICAL CONVOLUTIONAL NEURAL NETWORK AS LOOP FILTER FOR INTRA FRAME
- Log in to post comments
Loop filters are used in video coding to remove artifacts or improve performance. Recent advances in deploying convolutional neural network (CNN) to replace traditional loop filters show large gains but with problems for practical application.First, different model is used for frames encoded with different quantization parameter (QP), respectively. It is expensive for hardware. Second, float points operation in CNN leads to inconsistency between encoding and decoding across different platforms. Third, redundancy within CNN model consumes precious computational resources.
- Categories:
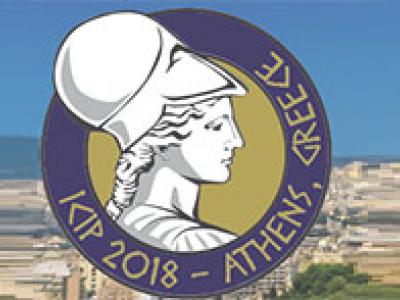
- Read more about SHPD: SURVEILLANCE HUMAN POSE DATASET AND PERFORMANCE EVALUATION FOR COARSE-GRAINED POSE ESTIMATION
- Log in to post comments
Pose estimation is highly valued in surveillance systems in the era of big data. However, current human pose datasets are limited in their coverage of the pose estimation challenges in outdoor surveillance scenarios. In this paper, we introduce a novel Surveillance Human Pose Dataset (SHPD).
poster-cqh.pdf

- Categories:
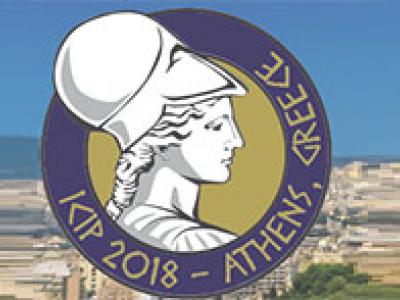
- Read more about Light Field Image Restoration for Vision in Scattering Media
- Log in to post comments
Recovering information from contrast-limited, SNR-limited, color-attenuated images in a scattering medium is of paramount importance for the autonomous functioning of robotic agents. The task is challenging due to the transient state of the medium, unknown medium parameters and in many cases the need for fully autonomous operation. This work presents a target-less, calibration-less method for restoring underwater light field images and requires no explicit model of the medium.
ICIPPoster.pdf

- Categories:
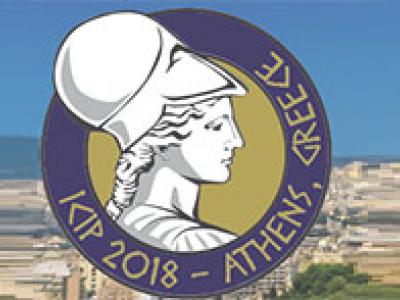
- Read more about Adaptive local image enhancement based on logarithmic mappings
- Log in to post comments
A new local image enhancement technique is proposed.
It is based on a logarithmic mapping function
that is adapted to the luminance
characteristics of the neighborhood of each pixel.
The method permits to simultaneously increase the luminance in dark regions
and decrease it in bright regions. The result is an image with an overall improved
contrast.
- Categories:
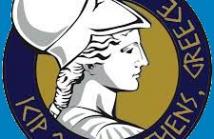
- Read more about MULTI-THRESHOLD LIP CONTOUR DETECTION
- Log in to post comments
In this work, we propose a novel, multi-threshold method for lip contour extraction from high-resolution static lip images acquired in an uncontrolled environment and emphasizing on the contour details.
The introduced method is a “Divide and conquer” approach. We broke the problem of lip contour extraction into two base sub-problems of locating the upper and lower lip contours using a novel threshold selection algorithm and combining them into the solution for the original lip contour.
- Categories:
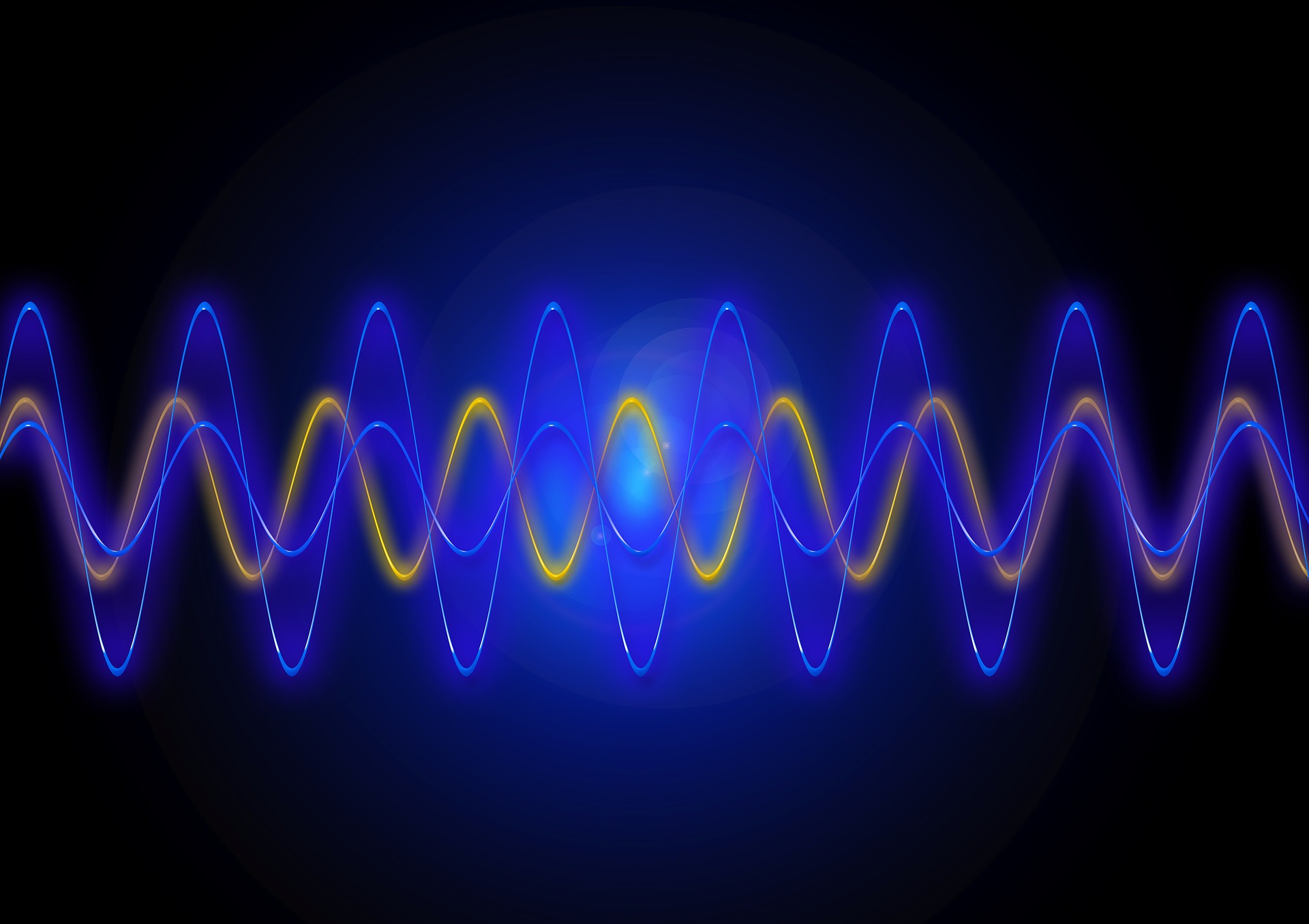
- Read more about Near-Constant Time Bilateral Filter for High-Dimensional Images
- Log in to post comments
- Categories:
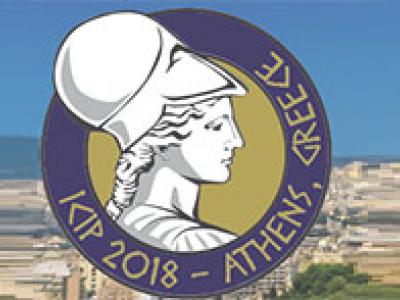
This paper presents a method for stabilizing both cylindrical and spherical panorama videos with a 360-degree field of view. We observe that rotation needs to be extremely smooth for 360 videos to maintain global motion coherency and avoid wobbling. Our method decouples the rotation from other motions and applies different strategies for smoothing them. The proposed approach is 2.5D as it estimates 3D rotations without involving 3D structure-from-motion methods. Therefore, it is more robust and can be performed in an incremental way.
- Categories:
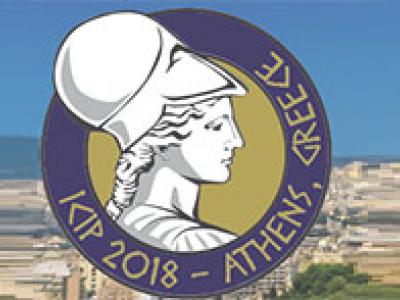
- Read more about GENERATING A PERSPECTIVE IMAGE FROM A PANORAMIC IMAGE BY THE SWUNG-TO-CYLINDER PROJECTION
- Log in to post comments
This paper proposes a swung-to-cylinder projection model for mapping a sphere to a plane. It can be used to create a semi-perspective image from a panoramic image. The model has two steps. In the first step, the sphere is projected onto a swung surface constructed by a circular profile and a rounded rectangular trajectory. In the second step, the projected image on the swung surface is mapped onto a cylinder through the perspective projection. We also propose methods for automatically determining proper parameters for the projection model based on image content.
- Categories:
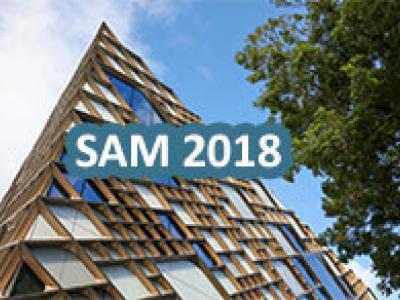
- Read more about Estimation of the regularization parameter of an on-line NMF with minimum volume constraint
- Log in to post comments
- Categories:
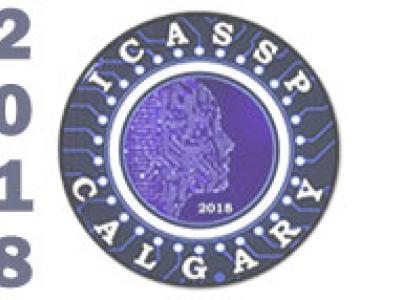
- Read more about RCDFNN: Robust Change Detection based on Convolutional Fusion Neural Network
- Log in to post comments
Video change detection, which plays an important role in computer vision, is far from being well resolved due to the complexity of diverse scenes in real world. Most of the current methods are designed based on hand-crafted features and perform well in some certain scenes but may fail on others. This paper puts up forward a deep learning based method to automatically fuse multiple basic detections into an optimal
- Categories: