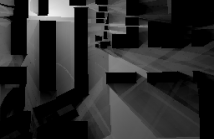
- Read more about Presentation Slides of "The First Pathloss Radio Map Prediction Challenge"
- Log in to post comments
To foster research and facilitate fair comparisons among recently proposed pathloss radio map prediction methods, we have launched the ICASSP 2023 First Pathloss Radio Map Prediction Challenge.
In this short overview, we briefly describe the pathloss prediction problem, the provided datasets, the challenge task and the challenge evaluation methodology. Finally, we present the results of the challenge.
- Categories:
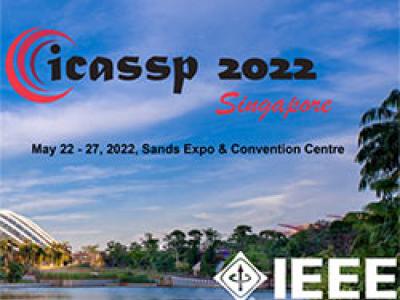
- Read more about IMPQ: Reduced Complexity Neural Networks via Granular Precision Assignment
- Log in to post comments
- Categories:
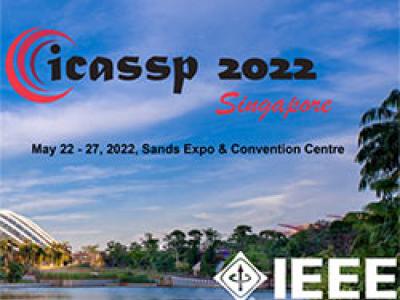
- Read more about Mixture Model Auto-Encoders: Deep Clustering through Dictionary Learning
- Log in to post comments
mixmate.pdf

- Categories:
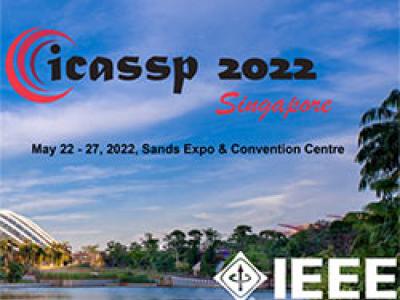
- Read more about Neural Collapse in Deep Homogeneous Classifiers and the Role of Weight Decay
- Log in to post comments
Neural Collapse is a phenomenon recently discovered in deep classifiers where the last layer activations collapse onto their class means, while the means and last layer weights take on the structure of dual equiangular tight frames. In this paper we present results showing the role of weight decay in the emergence of Neural Collapse in deep homogeneous networks. We show that certain near-interpolating minima of deep networks satisfy the Neural Collapse condition, and this can be derived from the gradient flow on the regularized square loss.
- Categories:
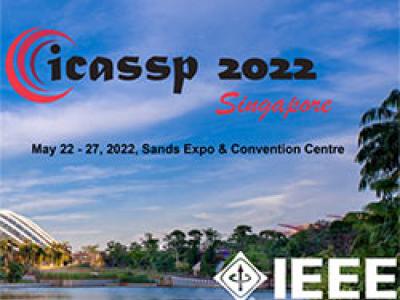
- Read more about Temporal Knowledge Distillation for On-device Audio Classification
- Log in to post comments
- Categories:
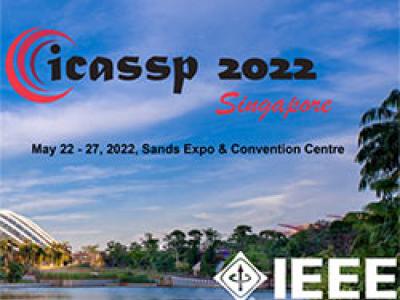
- Read more about Temporal Knowledge Distillation for On-device Audio Classification
- Log in to post comments
- Categories:
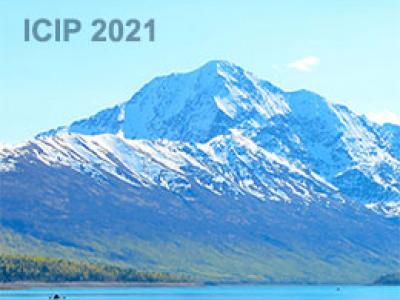
- Read more about Motivating bilevel approaches to filter learning: A case study
- Log in to post comments
The recent trend in regularization methods for inverse problems is to replace handcrafted sparsifying operators with data-driven approaches. Although using such machine learning techniques often improves image reconstruction methods, the results can depend significantly on the learning methodology. This paper compares two supervised learning methods. First, the paper considers a transform learning approach and, to learn the transform, introduces a variant on the Procrustes method for wide matrices with orthogonal rows. Second, we consider a bilevel convolutional filter learning approach.
- Categories:
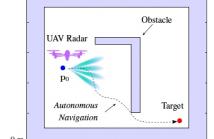
In this paper we consider a joint detection, mapping and navigation problem by an unmanned aerial vehicle (UAV) with real-time learning capabilities. We formulate this problem as a Markov decision process (MDP), where the UAV is equipped with a THz radar capable to electronically scan the environment with high accuracy and to infer its probabilistic occupancy map. The navigation task amounts to maximizing the desired mapping accuracy and coverage and to decide whether targets (e.g., people carrying radio devices) are present or not.
- Categories:
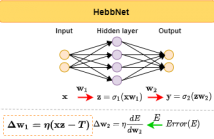
- Read more about HEBBNET: A SIMPLIFIED HEBBIAN LEARNING FRAMEWORK TO DO BIOLOGICALLY PLAUSIBLE LEARNING
- Log in to post comments
Backpropagation has revolutionized neural network training however, its biological plausibility remains questionable. Hebbian learning, a completely unsupervised and feedback free learning technique is a strong contender for a biologically plausible alternative. However, so far, it has neither achieved high accuracy performance vs. backprop, nor is the training procedure simple. In this work, we introduce a new Hebbian learning based neural network, called Hebb-Net.
Video_slides.pdf

- Categories:
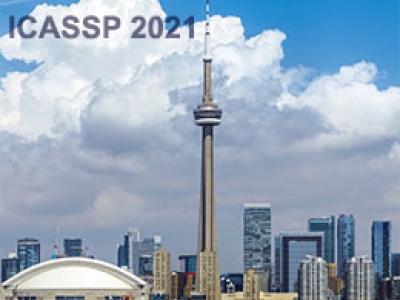
- Read more about A Unified Approach to Translate Classical Bandit algorithms to Structured Bandits
- Log in to post comments
- Categories: