- Acoustic Modeling for Automatic Speech Recognition (SPE-RECO)
- General Topics in Speech Recognition (SPE-GASR)
- Large Vocabulary Continuous Recognition/Search (SPE-LVCR)
- Lexical Modeling and Access (SPE-LEXI)
- Multilingual Recognition and Identification (SPE-MULT)
- Resource constrained speech recognition (SPE-RCSR)
- Robust Speech Recognition (SPE-ROBU)
- Speaker Recognition and Characterization (SPE-SPKR)
- Speech Adaptation/Normalization (SPE-ADAP)
- Speech Analysis (SPE-ANLS)
- Speech Coding (SPE-CODI)
- Speech Enhancement (SPE-ENHA)
- Speech Perception and Psychoacoustics (SPE-SPER)
- Speech Production (SPE-SPRD)
- Speech Synthesis and Generation, including TTS (SPE-SYNT)
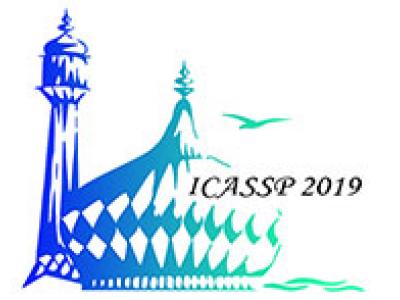
- Read more about Attentive Adversarial Learning for Domain-Invariant Training
- Log in to post comments
Adversarial domain-invariant training (ADIT) proves to be effective in suppressing the effects of domain variability in acoustic modeling and has led to improved performance in automatic speech recognition (ASR). In ADIT, an auxiliary domain classifier takes in equally-weighted deep features from a deep neural network (DNN) acoustic model and is trained to improve their domain-invariance by optimizing an adversarial loss function.
- Categories:
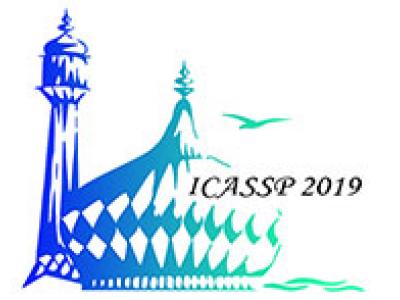
- Read more about Adversarial Speaker Verification
- Log in to post comments
The use of deep networks to extract embeddings for speaker recognition has proven successfully. However, such embeddings are susceptible to performance degradation due to the mismatches among the training, enrollment, and test conditions. In this work, we propose an adversarial speaker verification (ASV) scheme to learn the condition-invariant deep embedding via adversarial multi-task training. In ASV, a speaker classification network and a condition identification network are jointly optimized to minimize the speaker classification loss and simultaneously mini-maximize the condition loss.
- Categories:
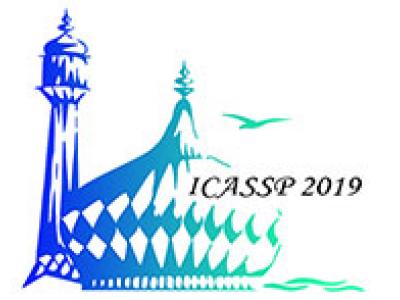
- Read more about Conditional Teacher-Student Learning
- Log in to post comments
The teacher-student (T/S) learning has been shown to be effective for a variety of problems such as domain adaptation and model compression. One shortcoming of the T/S learning is that a teacher model, not always perfect, sporadically produces wrong guidance in form of posterior probabilities that misleads the student model towards a suboptimal performance.
- Categories:
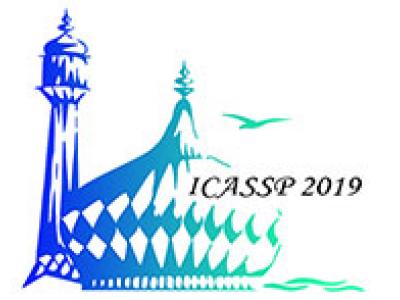
- Read more about Role Specific Lattice Rescoring for Speaker Role Recognition from Speech Recognition Outputs
- Log in to post comments
The language patterns followed by different speakers who play specific roles in conversational interactions provide valuable cues for the task of Speaker Role Recognition (SRR). Given the speech signal, existing algorithms typically try to find such patterns in the output of an Automatic Speech Recognition (ASR) system. In this work we propose an alternative way of revealing role-specific linguistic characteristics, by making use of role-specific ASR outputs, which are built by suitably rescoring the lattice produced after a first pass of ASR decoding.
- Categories:
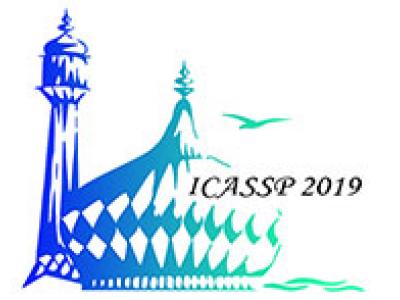
- Read more about Retrieving Speech Samples with Similar Emotional Content Using a Triplet Loss Function
- Log in to post comments
The ability to identify speech with similar emotional content is valuable to many applications, including speech retrieval, surveillance, and emotional speech synthesis. While current formulations in speech emotion recognition based on classification or regression are not appropriate for this task, solutions based on preference learning offer appealing approaches for this task. This paper aims to find speech samples that are emotionally similar to an anchor speech sample provided as a query. This novel formulation opens interesting research questions.
- Categories:
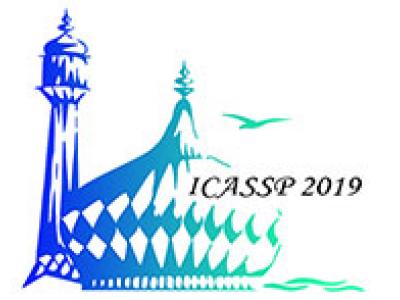
- Read more about SPEAKER AGNOSTIC FOREGROUND SPEECH DETECTION FROM AUDIO RECORDINGS IN WORKPLACE SETTINGS FROM WEARABLE RECORDERS
- Log in to post comments
Audio-signal acquisition as part of wearable sensing adds an important dimension for applications such as understanding human behaviors. As part of a large study on work place behaviors, we collected audio data from individual hospital staff using custom wearable recorders. The audio features collected were limited to preserve privacy of the interactions in the hospital. A first step towards audio processing is to identify the foreground speech of the person wearing the audio badge.
- Categories:
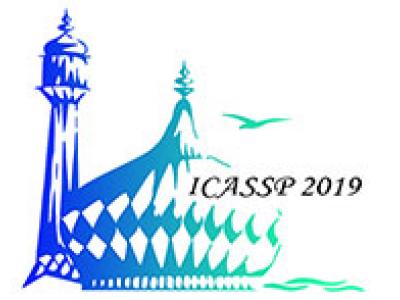
- Read more about MULTI-BAND PIT AND MODEL INTEGRATION FOR IMPROVED MULTI-CHANNEL SPEECH SEPARATION
- Log in to post comments
- Categories:
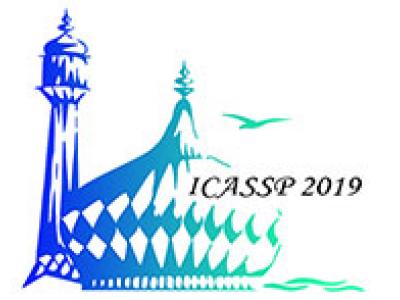
- Read more about PhoneSpoof: A new dataset for spoofing attack detection in telephone channel
- Log in to post comments
The results of spoofing detection systems proposed during ASVspoof Challenges 2015 and 2017 confirmed the perspective in detection of unforseen spoofing trials in microphone channel. However, telephone channel presents much more challenging conditions for spoofing detection, due to limited bandwidth, various coding standards and channel effects. Research on the topic has thus far only made use of program codecs and other telephone channel emulations. Such emulations does not quite match the real telephone spoofing attacks.
- Categories:
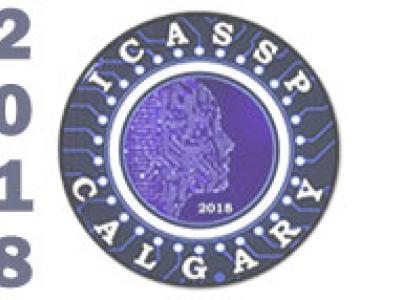
- Read more about Comparison of speech tasks for automatic classification of patients with amyotrophic lateral sclerosis and healthy subjects
- Log in to post comments
In this work, we consider the task of acoustic and articulatory feature based automatic classification of Amyotrophic Lateral Sclerosis (ALS) patients and healthy subjects using speech tasks. In particular, we compare the roles of different types of speech tasks, namely rehearsed speech, spontaneous speech and repeated words for this purpose. Simultaneous articulatory and speech data were recorded from 8 healthy controls and 8 ALS patients using AG501 for the classification experiments.
- Categories: