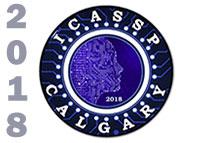
ICASSP is the world’s largest and most comprehensive technical conference focused on signal processing and its applications. The 2019 conference will feature world-class presentations by internationally renowned speakers, cutting-edge session topics and provide a fantastic opportunity to network with like-minded professionals from around the world. Visit website.
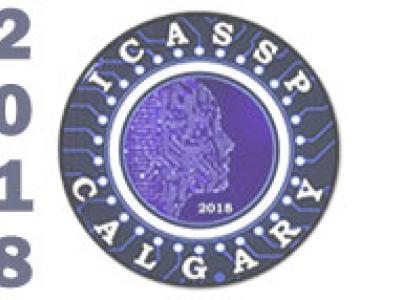
- Read more about RECOGNIZING ZERO-RESOURCED LANGUAGES BASED ON MISMATCHED MACHINE TRANSCRIPTIONS
- Log in to post comments
Mismatched crowdsourcing based probabilistic human transcription has been proposed recently for training and adapting acoustic models for zero-resourced languages where we do not have any native transcriptions. This paper describes a machine transcription based phone recognition system for recognizing zero-resourced languages and compares it with baseline systems of MAP adaptation and semi-supervised self training.
Poster.pdf

- Categories:
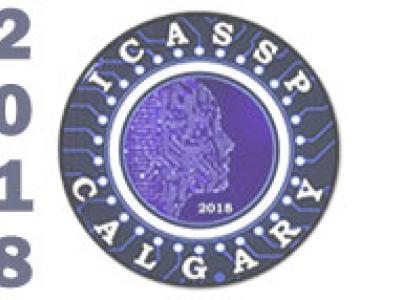
- Read more about VOICE CONVERSION THROUGH RESIDUAL WARPING IN A SPARSE, ANCHOR-BASED REPRESENTATION OF SPEECH
- Log in to post comments
In previous work we presented a Sparse, Anchor-Based Representation of speech (SABR) that uses phonemic “anchors” to represent an utterance with a set of sparse non-negative weights. SABR is speaker-independent: combining weights from a source speaker with anchors from a target speaker can be used for voice conversion. Here, we present an extension of the original SABR that significantly improves voice conversion synthesis.
- Categories:
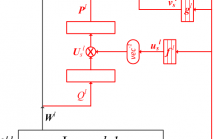
- Read more about Domain and speaker adaptation for Cortana Speech Recognition
- Log in to post comments
Voice assistant represents one of the most popular and important scenarios for speech recognition. In this paper, we propose two adaptation approaches to customize a multi-style well-trained acoustic model towards its subsidiary domain of Cortana assistant. First, we present anchor-based speaker adaptation by extracting the speaker information, i-vector or d-vector embeddings, from the anchor segments of `Hey Cortana'. The anchor embeddings are mapped to layer-wise parameters to control the transformations of both weight matrices and biases of multiple layers.
- Categories:
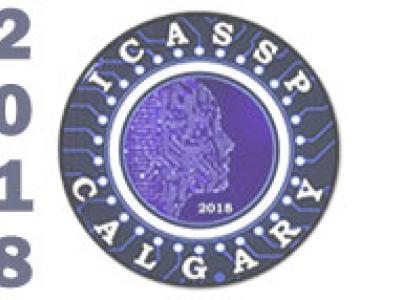
- Read more about Cooperative Tracking using Marginal Diffusion Particle Filters
- Log in to post comments
This paper formulates the general Adapt-then-Combine (ATC) and Random Exchange (RndEx) diffusion filters for an arbitrary nonlinear state-space model. Subsequently, we propose two novel marginal Particle Filter implementations of the general ATC and RndEx filters using respectively a pure Sequential Monte Carlo (SMC) strategy and a hybrid Gaussian/SMC methodology. The proposed algorithms are assessed via simulation in a numerical example of cooperative target tracking with received-signal-strength (RSS) sensors.
- Categories:
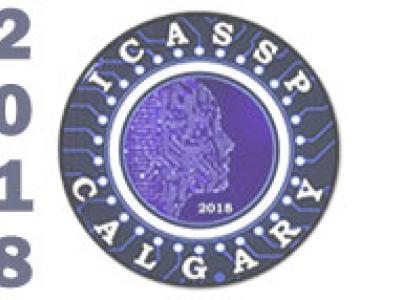
- Read more about Greedy Algorithm With Approximation Ratio For Sampling Noisy Graph Signals
- Log in to post comments
We study the optimal sampling set selection problem in sampling a noisy $k$-bandlimited graph signal. To minimize the effect of noise when trying to reconstruct a $k$-bandlimited graph signal from $m$ samples, the optimal sampling set selection problem has been shown to be equivalent to finding a $m \times k$ submatrix with the maximum smallest singular value, $\sigma_{\min}$ \cite{chen2015discrete}. As the problem is NP-hard, we present a greedy algorithm inspired by a similar submatrix selection problem known in computer science and to which we add a local search refinement.
- Categories:
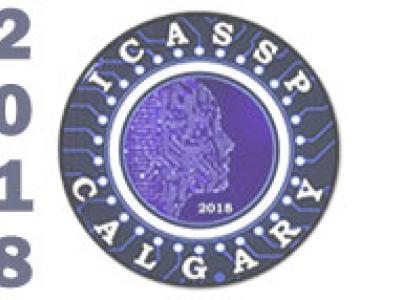
- Read more about InstListener: An Expressive Parameter Estimation System Imitating Human Performances of Monophonic Musical Instruments
- Log in to post comments
We present InstListener, a system that takes an expressive mono- phonic solo instrument performance by a human performer as the input and imitates its audio recordings by using an existing MIDI (Musical Instrument Digital Interface) synthesizer. It automatically analyzes the input and estimates, for each musical note, expressive performance parameters such as the timing, duration, discrete semitone-level pitch, amplitude, continuous pitch contour, and continuous amplitude contour.
- Categories:
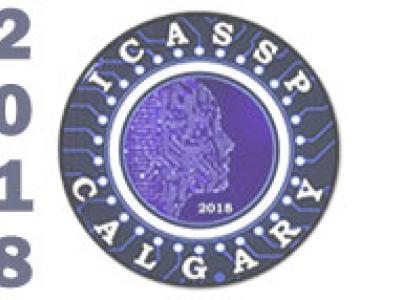
- Read more about Discriminative Probabilistic Framework for Generalized Multi-Instance Learning
- Log in to post comments
Multiple-instance learning is a framework for learning from data consisting of bags of instances labeled at the bag level. A common assumption in multi-instance learning is that a bag label is positive if and only if at least one instance in the bag is positive. In practice, this assumption may be violated. For example, experts may provide a noisy label to a bag consisting of many instances, to reduce labeling time.
- Categories:
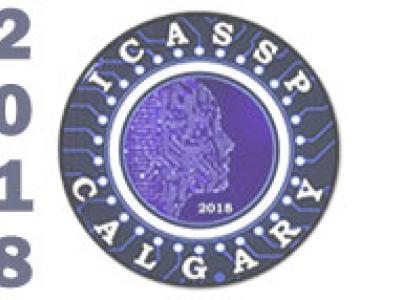
- Read more about SOLVING LINEAR INVERSE PROBLEMS USING GAN PRIORS: AN ALGORITHM WITH PROVABLE GUARANTEES
- Log in to post comments
In recent works, both sparsity-based methods as well as learning-based methods have proven to be successful in solving several challenging linear inverse problems. However, sparsity priors for natural signals and images suffer from poor discriminative capability, while learning-based methods seldom provide concrete theoretical guarantees. In this work, we advocate the idea of replacing hand-crafted priors, such as sparsity, with a Generative Adversarial Network (GAN) to solve linear inverse problems such as compressive sensing.
- Categories:
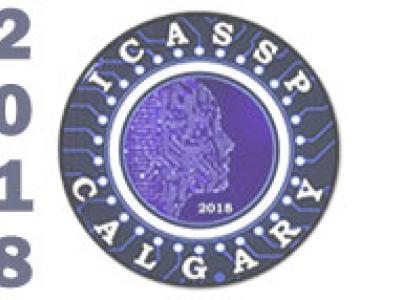
We propose a novel adversarial multi-task learning scheme, aiming at actively curtailing the inter-talker feature variability while maximizing its senone discriminability so as to enhance the performance of a deep neural network (DNN) based ASR system. We call the scheme speaker-invariant training (SIT). In SIT, a DNN acoustic model and a speaker classifier network are jointly optimized to minimize the senone (tied triphone state) classification loss, and simultaneously mini-maximize the speaker classification loss.
- Categories:
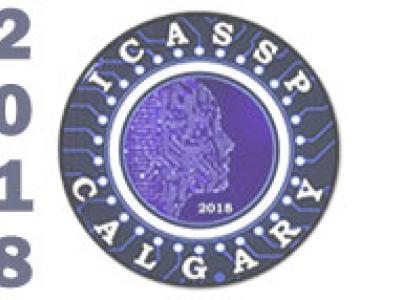
- Read more about Improving Semi-Supervised Classification for Low-Resource Speech Interaction Applications
- Log in to post comments
We propose a semi-supervised learning method to improve classification performance in scenarios with limited labeled
data. We employ adaptation strategies such as entropy-filtering and self-training, and show that our method achieves
- Categories: