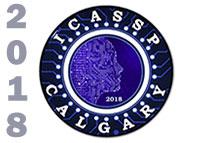
ICASSP is the world’s largest and most comprehensive technical conference focused on signal processing and its applications. The 2019 conference will feature world-class presentations by internationally renowned speakers, cutting-edge session topics and provide a fantastic opportunity to network with like-minded professionals from around the world. Visit website.
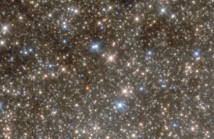
- Read more about Convolutional group-sparse coding and source localization - Poster
- Log in to post comments
In this paper, we present a new interpretation of non-negatively constrained convolutional coding problems as blind deconvolution problems with spatially variant point spread function. In this light, we propose an optimization framework that generalizes our previous work on non-negative group sparsity for convolutional models. We then link these concepts to source localization problems that arise in scientific imaging, and provide a visual example on an image derived from data captured by the Hubble telescope.
- Categories:
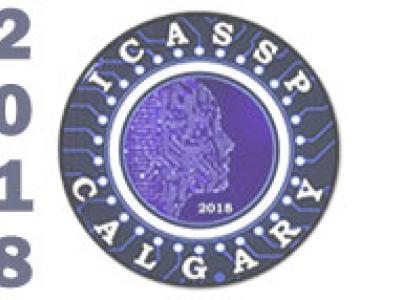
- Read more about AUTOMATIC MOTION ARTIFACT DETECTION FOR WHOLE-BODY MAGNETIC RESONANCE IMAGING
- Log in to post comments
Magnetic resonance (MR) plays an important role in medical imaging. It can be flexibly tuned towards different applications for deriving a meaningful diagnosis. However, its long acquisition times and flexible parametrization make it on the other hand prone to artifacts which obscure the underlying image content or can be misinterpreted as anatomy. Patient-induced motion artifacts are still one of the major extrinsic factors which degrade image quality.
- Categories:
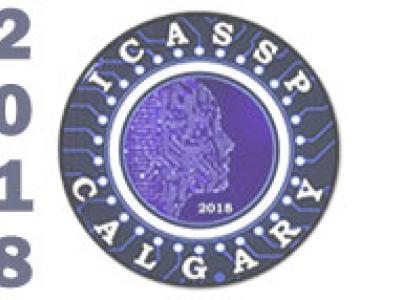
- Read more about IMPROVING DISPARITY MAP ESTIMATION FOR MULTI-VIEW NOISY IMAGES
- Log in to post comments
A robust multi-view disparity estimation algorithm for noisy images is presented. The proposed algorithm constructs 3D focus image stacks (3DFIS) by projecting and stacking multi-view images and estimates a disparity map based on the 3DFIS. To make the algorithm robust to noise and occlusion, a texture-based view selection and patch size variation scheme based on texture map is proposed.
- Categories:
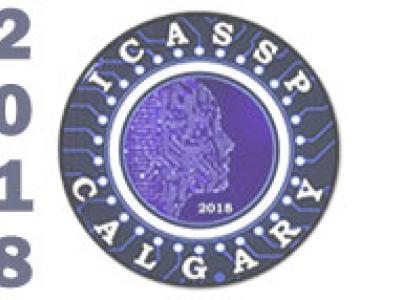
- Read more about TIME-FREQUENCY MASKING-BASED SPEECH ENHANCEMENT USING GENERATIVE ADVERSARIAL NETWORK
- Log in to post comments
- Categories:
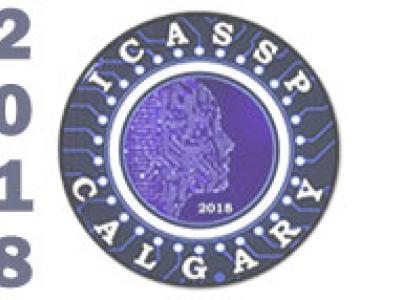
- Read more about Sequence Modeling in Unsupervised Single-channel Overlapped Speech Recognition
- Log in to post comments
Unsupervised single-channel overlapped speech recognition is one
of the hardest problems in automatic speech recognition (ASR). The
problems can be modularized into three sub-problems: frame-wise
interpreting, sequence level speaker tracing and speech recognition.
Nevertheless, previous acoustic models formulate the correlation between sequential labels implicitly, which limit the modeling effect.
In this work, we include explicit models for the sequential label
correlation during training. This is relevant to models given by both
- Categories:
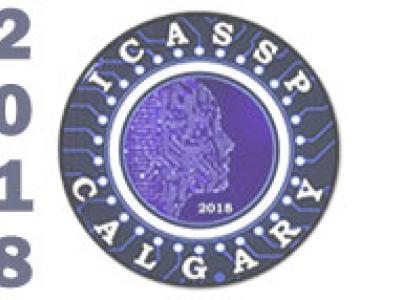
- Read more about OCTAGONAL-AXIS RASTER PATTERN FOR IMPROVED TEST ZONE SEARCH MOTION ESTIMATION
- Log in to post comments
Test Zone Search (TZS) is considered the current state-of-the-art fast Motion Estimation algorithm because it presents
- Categories:
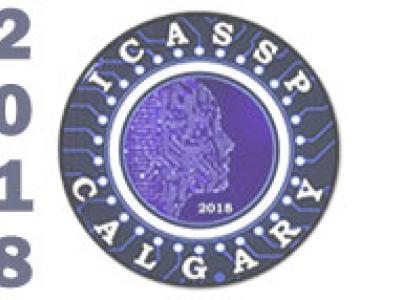
- Categories:
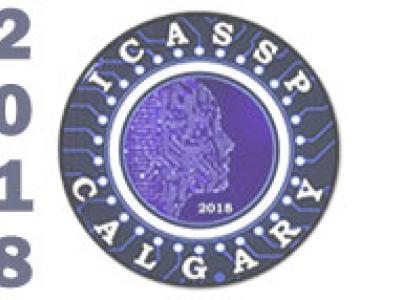
- Read more about On Modular Training of Neural Acoustics-to-Word Model for LVCSR
- Log in to post comments
End-to-end (E2E) automatic speech recognition (ASR) systems directly map acoustics to words using a unified model. Previous works
mostly focus on E2E training a single model which integrates acoustic and language model into a whole. Although E2E training benefits
from sequence modeling and simplified decoding pipelines, large
amount of transcribed acoustic data is usually required, and traditional acoustic and language modelling techniques cannot be utilized. In this paper, a novel modular training framework of E2E ASR
- Categories:
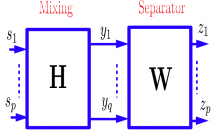
In this article, we propose a Bounded Component Analysis (BCA) approach for the separation of the convolutive mixtures of sparse sources. The corresponding algorithm is derived from a geometric objective function defined over a completely deterministic setting. Therefore, it is applicable to sources which can be independent or dependent in both space and time dimensions. We show that all global optima of the proposed objective are perfect separators. We also provide numerical examples to illustrate the performance of the algorithm.
- Categories: