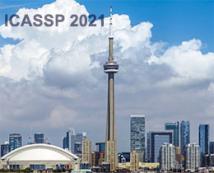
ICASSP 2021 - IEEE International Conference on Acoustics, Speech and Signal Processing is the world’s largest and most comprehensive technical conference focused on signal processing and its applications. The ICASSP 2021 conference will feature world-class presentations by internationally renowned speakers, cutting-edge session topics and provide a fantastic opportunity to network with like-minded professionals from around the world. Visit website.
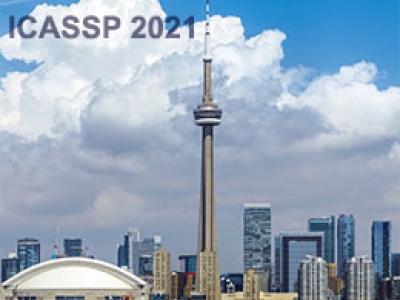
- Read more about SPARSE-CODED DYNAMIC MODE DECOMPOSITION ON GRAPH FOR PREDICTION OF RIVER WATER LEVEL DISTRIBUTION
- Log in to post comments
This work proposes a method for estimating dynamics on graph by using dynamic mode decomposition (DMD) and sparse approximation with graph filter banks (GFBs). The motivation of introducing DMD on graph is to predict multi-point river water levels for forecasting river flood and giving proper evacuation warnings. The proposed method represents a spatio-temporal variation of physical quantities on a graph as a time-evolution equation. Specifically, water level observation data available on the Internet is collected by web scraping.
- Categories:
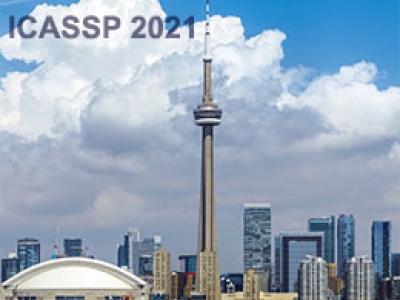
- Read more about G-ARRAYS: GEOMETRIC ARRAYS FOR EFFICIENT POINT CLOUD PROCESSING
- Log in to post comments
Slides.pdf

- Categories:
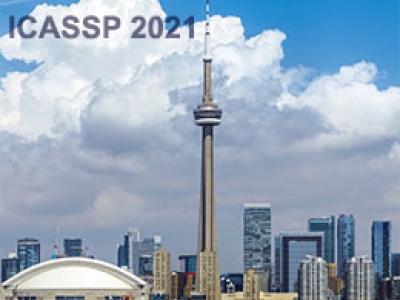
- Read more about Generating Human Readable Transcript for Automatic Speech Recognition with Pre-trained Language Model
- Log in to post comments
Modern Automatic Speech Recognition (ASR) systems can achieve high performance in terms of recognition accuracy. However, a perfectly accurate transcript still can be challenging to read due to disfluency, filter words, and other errata common in spoken communication. Many downstream tasks and human readers rely on the output of the ASR system; therefore, errors introduced by the speaker and ASR system alike will be propagated to the next task in the pipeline.
- Categories:
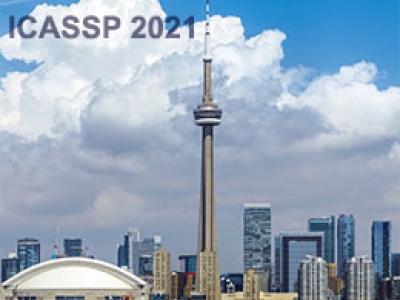
- Read more about END-TO-END MULTILINGUAL AUTOMATIC SPEECH RECOGNITION FOR LESS-RESOURCED LANGUAGES: THE CASE OF FOUR ETHIOPIAN LANGUAGES
- Log in to post comments
End-to-End (E2E) approach, which maps a sequence of input features into a sequence of grapheme or words, to Automatic Speech Recognition (ASR) is a hot research agenda. It is interesting for less-resourced languages since it avoids the use of pronunciation dictionary, which is one of the major components in the traditional ASR systems. However, like any deep neural network (DNN) approaches, E2E is data greedy. This makes the application of E2E to less-resourced languages questionable.
- Categories:
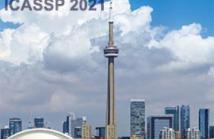
A scalable algorithm is derived for multilevel quantization of sensor observations in distributed sensor networks, which consist of a number of sensors transmitting a summary information of their observations to the fusion center for a final decision. The proposed algorithm is directly minimizing the overall error probability of the network without resorting to minimizing pseudo objective functions such as distances between probability distributions.
icassp2021_poster.pdf

- Categories:
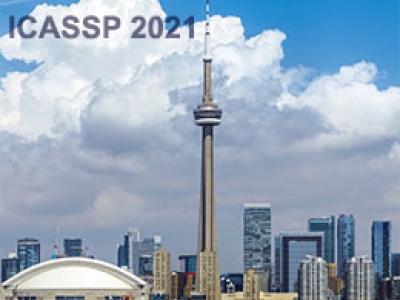
- Read more about Fast and Robust ADMM for Blind Super-resolution
- Log in to post comments
Though the blind super-resolution problem is nonconvex in nature, recent advance shows the feasibility of a convex formulation which gives the unique recovery guarantee. However, the convexification procedure is coupled with a huge computational cost and is therefore of great interest to investigate fast algorithms. To do so, we adapt an operator splitting approach ADMM and combine it with a novel preconditioning scheme. Numerical results show that the convergence rate is significantly improved by around two orders of magnitudes compared to the currently most adopted solver CVX.
- Categories:
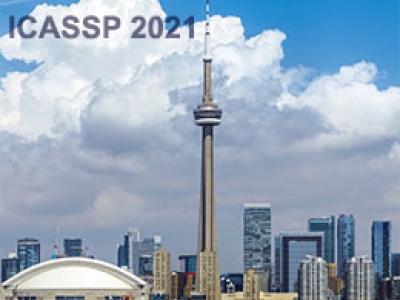
- Read more about AUDITORY FILTERBANKS BENEFIT UNIVERSAL SOUND SOURCE SEPARATION
- Log in to post comments
- Categories:
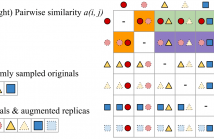
- Read more about Neural Audio Fingerprint for High-specific Audio Retrieval based on Contrastive Learning
- Log in to post comments
Most of existing audio fingerprinting systems have limitations to be used for high-specific audio retrieval at scale. In this work, we generate a low-dimensional representation from a short unit segment of audio, and couple this fingerprint with a fast maximum inner-product search. To this end, we present a contrastive learning framework that derives from the segment-level search objective. Each update in training uses a batch consisting of a set of pseudo labels, randomly selected original samples, and their augmented replicas.
- Categories:
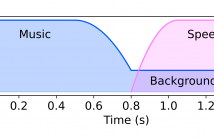
- Read more about ARTIFICIALLY SYNTHESISING DATA FOR AUDIO CLASSIFICATION AND SEGMENTATION TO IMPROVE SPEECH AND MUSIC DETECTION IN RADIO BROADCAST
- Log in to post comments
Segmenting audio into homogeneous sections such as music and speech helps us understand the content of audio. It is useful as a pre-processing step to index, store, and modify audio recordings, radio broadcasts and TV programmes. Deep learning models for segmentation are generally trained on copyrighted material, which cannot be shared. Annotating these datasets is time-consuming and expensive and therefore, it significantly slows down research progress. In this study, we present a novel procedure that artificially synthesises data that resembles radio signals.
- Categories: