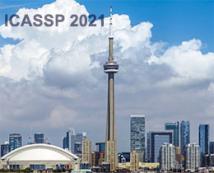
ICASSP 2021 - IEEE International Conference on Acoustics, Speech and Signal Processing is the world’s largest and most comprehensive technical conference focused on signal processing and its applications. The ICASSP 2021 conference will feature world-class presentations by internationally renowned speakers, cutting-edge session topics and provide a fantastic opportunity to network with like-minded professionals from around the world. Visit website.
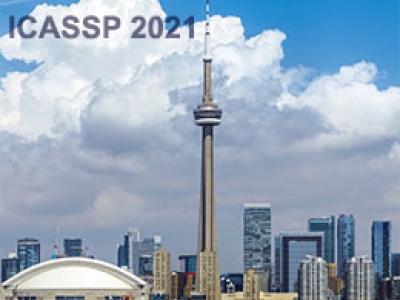
- Read more about Acoustic analysis and dataset of transitions between coupled rooms
- Log in to post comments
- Categories:
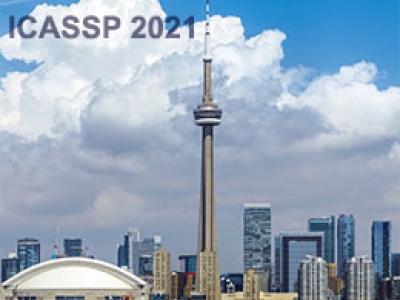
- Read more about PPT- Room Impulse Response Interpolation from a Sparse Set of Measurements Using a Modal Architecture
- Log in to post comments
In augmented reality applications, where room geometries and material properties are not readily available, it is desirable to get a representation of the sound field in a room from a limited set of available room impulse response measurements. In this paper, we propose a novel method for 2D interpolation of room modes from a sparse set of RIR measurements that are non-uniformly sampled within a space. We first obtain the mode parameters of a measured room.
ICASSP21_ppt_1473.pdf

- Categories:
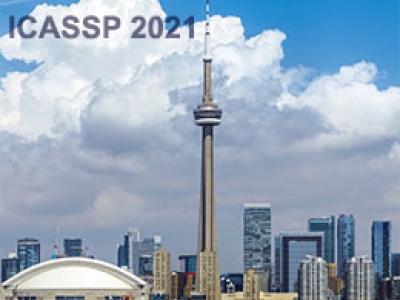
We summarise previous work showing that the basic sigmoid activation function arises as an instance of Bayes’s theorem, and that recurrence follows from the prior. We derive a layer- wise recurrence without the assumptions of previous work, and show that it leads to a standard recurrence with modest modifications to reflect use of log-probabilities. The resulting architecture closely resembles the Li-GRU which is the current state of the art for ASR. Although the contribution is mainly theoretical, we show that it is able to outperform the state of the art on the TIMIT and AMI datasets.
- Categories:
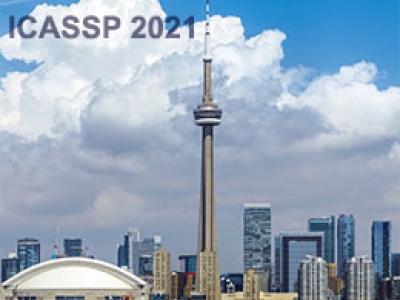
- Read more about Recurrent Phase Reconstruction Using Estimated Phase Derivatives from Deep Neural Networks
- Log in to post comments
This paper presents a deep neural network (DNN)-based system for phase reconstruction of speech signals solely from their magnitude spectrograms. The phase is very sensitive to time shifts. Therefore it is meaningful to estimate the phase derivatives instead of the phase directly, e.g., using DNNs and then apply a phase reconstruction method to recombine these estimates to a suitable phase spectrum. In this paper, we propose three changes for such a two-stage phase reconstruction system.
- Categories:
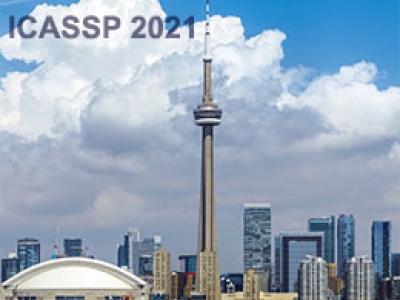
- Read more about Recurrent Phase Reconstruction Using Estimated Phase Derivatives from Deep Neural Networks
- Log in to post comments
This paper presents a deep neural network (DNN)-based system for phase reconstruction of speech signals solely from their magnitude spectrograms. The phase is very sensitive to time shifts. Therefore it is meaningful to estimate the phase derivatives instead of the phase directly, e.g., using DNNs and then apply a phase reconstruction method to recombine these estimates to a suitable phase spectrum. In this paper, we propose three changes for such a two-stage phase reconstruction system.
- Categories:
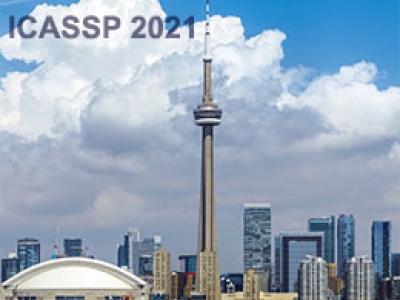
- Categories:
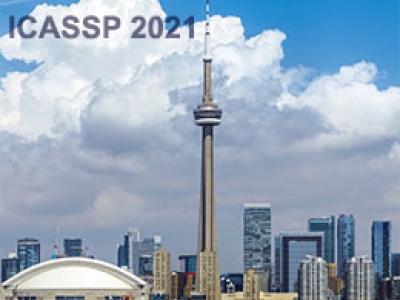
- Read more about GENERAL TOTAL VARIATION REGULARIZED SPARSE BAYESIAN LEARNING FOR ROBUST BLOCK-SPARSE SIGNAL RECOVERY
- Log in to post comments
Block-sparse signal recovery without knowledge of block sizes and boundaries, such as those encountered in multi-antenna mmWave channel models, is a hard problem for compressed sensing (CS) algorithms. We propose a novel Sparse Bayesian Learning (SBL) method for block-sparse recovery based on popular CS based regularizers with the function input variable related to total variation (TV). Contrary to conventional approaches that impose the regularization on the signal components, we regularize the SBL hyperparameters.
- Categories:
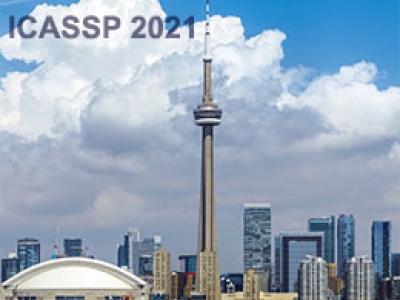
- Read more about Hierarchical Attention Fusion for Geo-Localization
- Log in to post comments
slides.pdf

poster.pdf

- Categories:
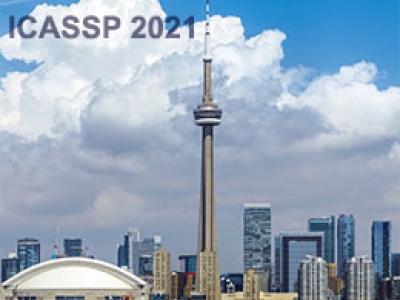
The identification of structural differences between a music performance and the score is a challenging yet integral step of audio-to-score alignment, an important subtask of music signal processing. We present a novel method to detect such differences between the score and performance for a given piece of music using progressively dilated convolutional neural networks. Our method incorporates varying dilation rates at different layers to capture both short-term and long-term context, and can be employed successfully in the presence of limited annotated data.
- Categories: