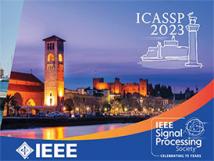
IEEE ICASSP 2023 - IEEE International Conference on Acoustics, Speech and Signal Processing is the world’s largest and most comprehensive technical conference focused on signal processing and its applications. The ICASSP 2023 conference will feature world-class presentations by internationally renowned speakers, cutting-edge session topics and provide a fantastic opportunity to network with like-minded professionals from around the world. Visit the website.
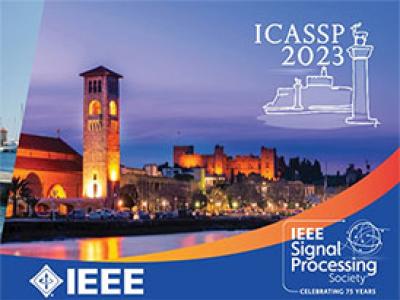
- Read more about QuantPipe: Applying Adaptive Post-Training Quantization for Distributed Transformer Pipelines in Dynamic Edge Environments
- Log in to post comments
Pipeline parallelism has achieved great success in deploying large-scale transformer models in cloud environments, but has received less attention in edge environments. Unlike in cloud scenarios with high-speed and stable network interconnects, dynamic bandwidth in edge systems can degrade distributed pipeline performance. We address this issue withQuantPipe, a communication-efficient distributed edge system that introduces post-training quantization (PTQ) to compress the communicated tensors.
- Categories:
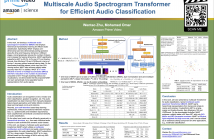
- Read more about Multiscale Audio Spectrogram Transformer for Efficient Audio Classification
- Log in to post comments
Audio event has a hierarchical architecture in both time and frequency and can be grouped together to construct more abstract semantic audio classes. In this work, we develop a multiscale audio spectrogram Transformer (MAST) that employs hierarchical representation learning for efficient audio classification. Specifically, MAST employs one-dimensional (and two-dimensional) pooling operators along the time (and frequency domains) in different stages, and progressively reduces the number of tokens and increases the feature dimensions.
- Categories:
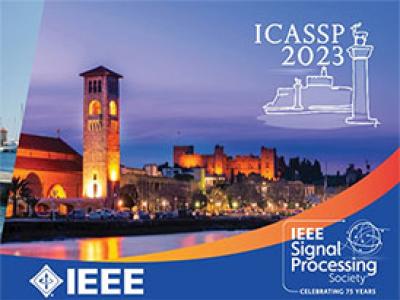
- Read more about InfoShape: Task-Based Neural Data Shaping via Mutual Information
- Log in to post comments
The use of mutual information as a tool in private data sharing has remained an open challenge due to the difficulty of its estimation in practice. In this paper, we propose InfoShape, a task-based encoder that aims to remove unnecessary sensitive information from training data while maintaining enough relevant information for a particular ML training task. We achieve this goal by utilizing mutual information estimators that are based on neural networks, in order to measure two performance metrics, privacy and utility.
- Categories:
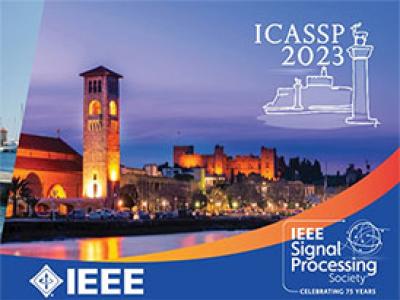
- Read more about Self-supervised learning of audio representations using angular contrastive loss
- Log in to post comments
- Categories:
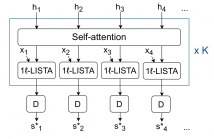
- Read more about Designing Transformer networks for sparse recovery of sequential data using deep unfolding
- Log in to post comments
Deep unfolding models are designed by unrolling an optimization algorithm into a deep learning network. These models have shown faster convergence and higher performance compared to the original optimization algorithms. Additionally, by incorporating domain knowledge from the optimization algorithm, they need much less training data to learn efficient representations. Current deep unfolding networks for sequential sparse recovery consist of recurrent neural networks (RNNs), which leverage the similarity between consecutive signals.
- Categories:
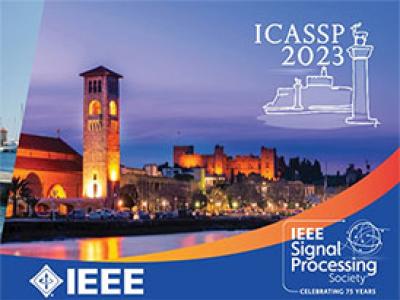
- Read more about Mixer: DNN Watermarking using Image Mixup
- Log in to post comments
It is crucial to protect the intellectual property rights of DNN models prior to their deployment. The
DNN should perform two main tasks: its primary task and watermarking task. This paper proposes
a lightweight, reliable, and secure DNN watermarking that attempts to establish strong ties between
these two tasks. The samples triggering the watermarking task are generated using image Mixup
either from training or testing samples. This means that there is an infinity of triggers not limited to the
- Categories:
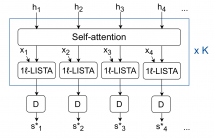
- Read more about Designing Transformer networks for sparse recovery of sequential data using deep unfolding: Presentation
- Log in to post comments
Deep unfolding models are designed by unrolling an optimization algorithm into a deep learning network. These models have shown faster convergence and higher performance compared to the original optimization algorithms. Additionally, by incorporating domain knowledge from the optimization algorithm, they need much less training data to learn efficient representations. Current deep unfolding networks for sequential sparse recovery consist of recurrent neural networks (RNNs), which leverage the similarity between consecutive signals.
- Categories:
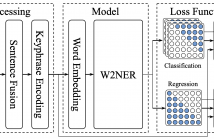
- Categories:
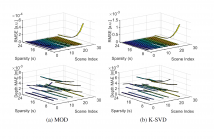
- Read more about Transient Dictionary Learning for Compressed Time-of-Flight Imaging
- Log in to post comments
Time-of-Flight imaging aims to retrieve the 3D geometry of a scene from the delay that a modulated light waveform experiences when interacting with the former.
Multi-path interference, arising from translucent objects or concave geometries, poses a challenge when the problem is to be solved from few measurements.
- Categories: