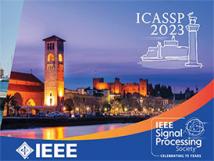
IEEE ICASSP 2023 - IEEE International Conference on Acoustics, Speech and Signal Processing is the world’s largest and most comprehensive technical conference focused on signal processing and its applications. The ICASSP 2023 conference will feature world-class presentations by internationally renowned speakers, cutting-edge session topics and provide a fantastic opportunity to network with like-minded professionals from around the world. Visit the website.
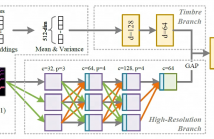
- Read more about TG-Critic: A Timbre-Guided Model for Reference-Independent Singing Evaluation
- Log in to post comments
Automatic singing evaluation independent of reference melody is a challenging task due to its subjective and multi-dimensional nature. As an essential attribute of singing voices, vocal timbre has a non-negligible effect and influence on human perception of singing quality. However, no research has been done to include timbre information explicitly in singing evaluation models. In this paper, a data-driven model TG-Critic is proposed to introduce timbre embeddings as one of the model inputs to guide the evaluation of singing quality.
- Categories:
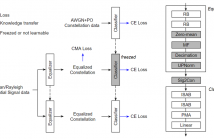
- Read more about EMC²-Net: Joint Equalization and Modulation Classification Based on Constellation Network
- Log in to post comments
Modulation classification (MC) is the first step performed at the receiver side unless the modulation type is explicitly indicated by the transmitter. Machine learning techniques have been widely used for MC recently. In this paper, we propose a novel MC technique dubbed as Joint Equalization and Modulation Classification based on Constellation Network (EMC²-Net). Unlike prior works that considered the constellation points as an image, the proposed EMC²-Net directly uses a set of 2D constellation points to perform MC.
- Categories:
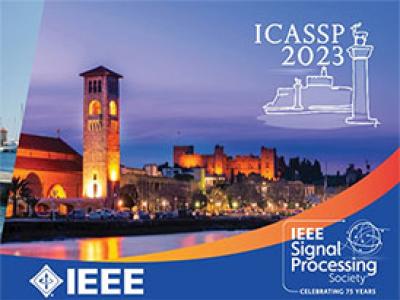
- Read more about Signal Analysis-Synthesis Using The Quantum Fourier Transform
- Log in to post comments
This paper presents the development of Quantum Fourier transform (QFT) education tools in the object-oriented Java-DSP (J-DSP) simulation environment. More specifically, QFT and Inverse QFT (IQFT) user-friendly J-DSP functions are developed to expose undergraduate students to quantum computing. These functions provide opportunities to examine QFT resolution, precision (qubits), and the effects of quantum measurement noise.
- Categories:
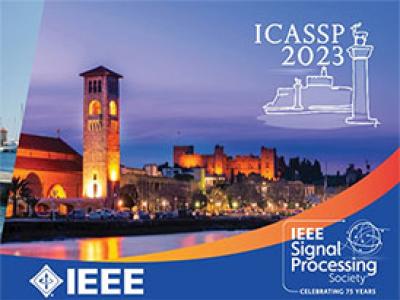
- Read more about GCT: GATED CONTEXTUAL TRANSFORMER FOR SEQUENTIAL AUDIO TAGGING
- Log in to post comments
- Categories:
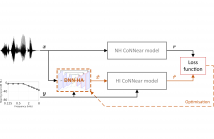
- Read more about DNN-HA: A DNN-based hearing-aid strategy for real-time processing
- Log in to post comments
Although hearing aids (HAs) can compensate for elevated hearing thresholds using sound amplification, they often fail to restore auditory perception in adverse listening conditions. Here, we present a deep-neural-network (DNN) HA processing strategy that can provide individualised sound processing for the audiogram of a listener using a single model architecture. Our multi-purpose HA model can be used for different individuals and can process audio inputs of 3.2 ms in <0.5 ms, thus paving the way for precise DNN-based treatments of hearing loss that can be embedded in hearing devices.
- Categories:

- Read more about Gluformer: Transformer-Based Personalized Glucose Forecasting with Uncertainty Quantification
- Log in to post comments
Deep learning models achieve state-of-the art results in predicting blood glucose trajectories, with a wide range of architectures being proposed. However, the adaptation of such models in clinical practice is slow, largely due to the lack of uncertainty quantification of provided predictions. In this work, we propose to model the future glucose trajectory conditioned on the past as an infinite mixture of basis distributions (i.e., Gaussian, Laplace, etc.).
- Categories:
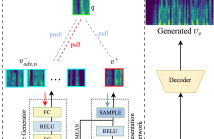
- Read more about CNEG-VC: Contrastive Learning using Hard Negative Example in Non-parallel Voice Conversion
- Log in to post comments
Contrastive learning has advantages for non-parallel voice conversion, but the previous conversion results could be better and more preserved. In previous techniques, negative samples were randomly selected in the features vector from different locations. A positive example could not be effectively pushed toward the query examples. We present contrastive learning in non-parallel voice conversion to solve this problem using hard negative examples. We named it CNEG-VC. Specifically, we teach the generator to generate negative examples. Our proposed generator has specific features.
- Categories:
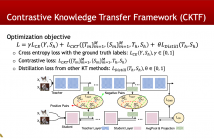
- Read more about A Contrastive Knowledge Transfer Framework for Model Compression and Transfer Learning
- 1 comment
- Log in to post comments
Knowledge Transfer (KT) achieves competitive performance and is widely used for image classification tasks in model compression and transfer learning. Existing KT works transfer the information from a large model ("teacher") to train a small model ("student") by minimizing the difference of their conditionally independent output distributions.
- Categories:
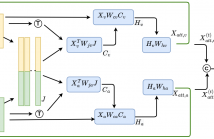
- Read more about Recursive Joint Attention for Audio-Visual Fusion in Regression-Based Emotion Recognition
- Log in to post comments
In video-based emotion recognition (ER), it is important to effectively leverage the complementary relationship among audio (A) and visual (V) modalities, while retaining the intramodal characteristics of individual modalities. In this paper, a recursive joint attention model is proposed along with long short-term memory (LSTM) modules for the fusion of vocal and facial expressions in regression-based ER.
- Categories:
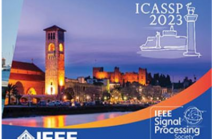
- Read more about Improved WiFI-based Respiration Tracking via Contrast Enhancement
- Log in to post comments
Respiratory rate tracking has gained more and more interest in the past few years because of its great potential in exploring different pathological conditions of human beings. Conventional approaches usually require dedicated wearable devices, making them intrusive and unfriendly to users. To tackle the issue, many WiFi-based respiration tracking systems have been proposed because of WiFi’s ubiquity, low-cost, and most importantly, contactlessness. However, most
- Categories: