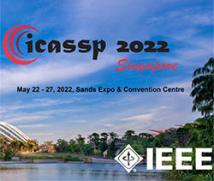
ICASSP 2022 - IEEE International Conference on Acoustics, Speech and Signal Processing is the world’s largest and most comprehensive technical conference focused on signal processing and its applications. The ICASSP 2022 conference will feature world-class presentations by internationally renowned speakers, cutting-edge session topics and provide a fantastic opportunity to network with like-minded professionals from around the world. Visit the website.
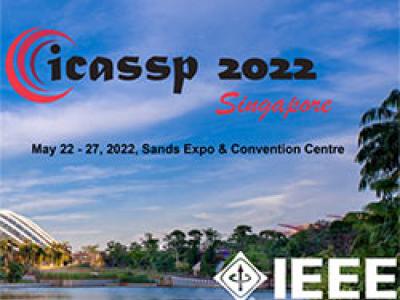
- Read more about DENOISING-GUIDED DEEP REINFORCEMENT LEARNING FOR SOCIAL RECOMMENDATION
- Log in to post comments
Social recommendation (SR) aims to enhance the performance of recommendations by incorporating social information. However, such information is not always reliable, e.g., some of the friends may share similar preferences with the user on a specific item, while others may be irrelevant to this item due to domain differences. Therefore, modeling all of the user's social relationships without considering the relevance of friends will introduce noises to the social context.
DRL4So.pptx

- Categories:
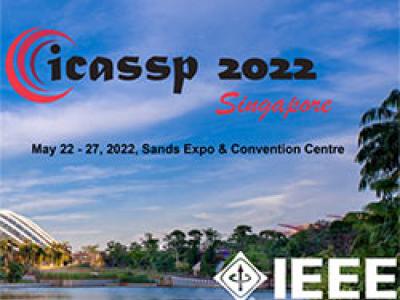
- Read more about DENOISING-ORIENTED DEEP HIERARCHICAL REINFORCEMENT LEARNING FOR NEXT-BASKET RECOMMENDATION
- Log in to post comments
Next basket recommendation aims to provide users a basket of items on the next visit by considering the sequence of their historical baskets. However, since a user's purchase interests vary over time, historical baskets often contain many irrelevant items to his/her next choices. Therefore, it is necessary to denoise the sequence of historical baskets and reserve the indeed relevant items to enhance the recommendation performance.
HRL4Ba.pptx

- Categories:
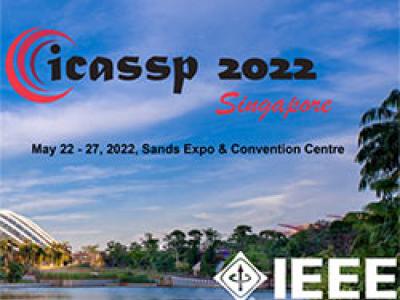
- Read more about Extracting and Distilling Direction-adaptive Knowledge for Lightweight Object Ddetection in Remote Sensing Images
- Log in to post comments
Recently, some lightweight convolutional neural network (CNN) models have been proposed for airborne or spaceborne remote sensing object detection (RSOD) tasks. However, these lightweight detectors suffer from performance degradation due to the compromise of limited computing resources on embedded devices. In order to narrow this performance gap, a direction-adaptive knowledge extraction and distillation (DKED) method is proposed.
Poster_Paper8521.pdf

- Categories:
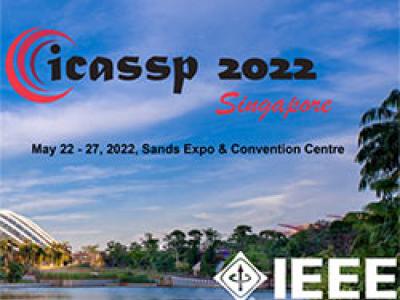
- Read more about Integration of Pre-trained Networks with Continuous Token Interface For End-to-End Spoken Language Understanding
- Log in to post comments
Most End-to-End (E2E) Spoken Language Understanding (SLU) networks leverage the pre-trained Automatic Speech Recognition (ASR) networks but still lack the capability to understand the semantics of utterances, crucial for the SLU task. To solve this, recently proposed studies use pre-trained Natural Language Understanding (NLU) networks. However, it is not trivial to fully utilize both pre-trained networks; many solutions were proposed, such as Knowledge Distillation (KD), cross-modal shared embedding, and network integration with Interface.
- Categories:
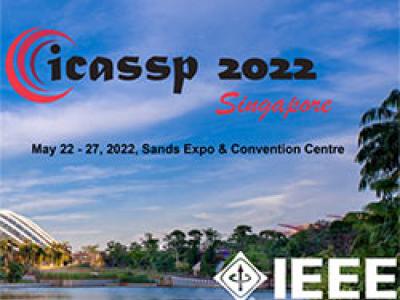
- Read more about Temporal Dynamic Convolutional Neural Network for Text-Independent Speaker Verification and Phonemic Analysis
- Log in to post comments
- Categories:
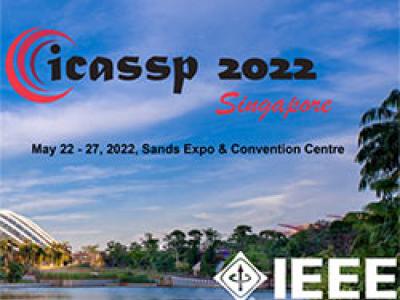
- Read more about ON THE USE OF GEODESIC TRIANGLES BETWEEN GAUSSIAN DISTRIBUTIONS FOR CLASSIFICATION PROBLEMS
- Log in to post comments
slides.pdf

- Categories:
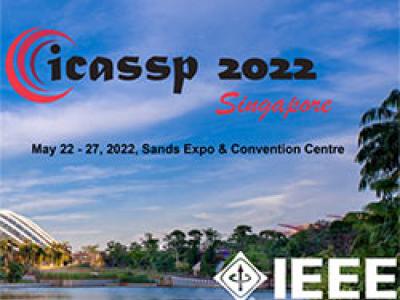
- Read more about ON THE USE OF GEODESIC TRIANGLES BETWEEN GAUSSIAN DISTRIBUTIONS FOR CLASSIFICATION PROBLEMS
- Log in to post comments
poster.pdf

- Categories:
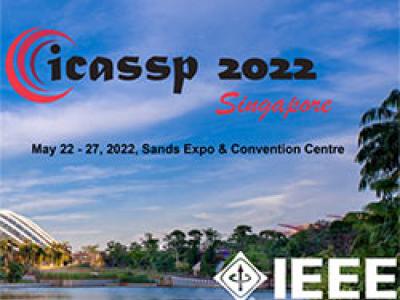
- Read more about OPENFEAT: Improving Speaker Identification by Open-set Few-shot Embedding Adaptation with Transformer
- Log in to post comments
Household speaker identification with few enrollment utterances is an important yet challenging problem, especially when household members share similar voice characteristics and room acoustics. A common embedding space learned from a large number of speakers is not universally applicable for the optimal identification of every speaker in a household.
- Categories:
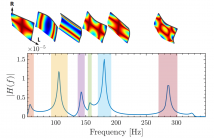
- Read more about On the Prediction of the Frequency Response of a Wooden Plate from its Mechanical Parameters
- Log in to post comments
Inspired by deep learning applications in structural mechanics, we focus on how to train two predictors to model the relation between the vibrational response of a prescribed point of a wooden plate and its material properties. In particular, the eigenfrequencies of the plate are estimated via multilinear regression, whereas their amplitude is predicted by a feedforward neural network.
- Categories:
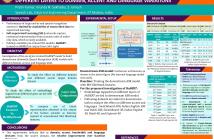
- Read more about INVESTIGATION OF ROBUSTNESS OF HUBERT FEATURES FROM DIFFERENT LAYERS TO DOMAIN, ACCENT AND LANGUAGE VARIATIONS
- Log in to post comments
In this paper, we investigate the use of pre-trained HuBERT model to build downstream Automatic Speech Recognition (ASR) models using data that have differences in domain, accent and even language. We use the standard ESPnet recipe with HuBERT as pretrained models whose output is fed as input features to a downstream Conformer model built from target domain data. We compare the performance of HuBERT pre-trained features with the baseline Conformer model built with Mel-filterbank features.
- Categories: