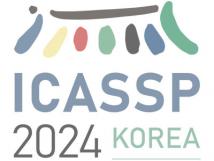
IEEE ICASSP 2024 - IEEE International Conference on Acoustics, Speech and Signal Processing (ICASSP) is the world’s largest and most comprehensive technical conference focused on signal processing and its applications. The IEEE ICASSP 2024 conference will feature world-class presentations by internationally renowned speakers, cutting-edge session topics and provide a fantastic opportunity to network with like-minded professionals from around the world. Visit the website.
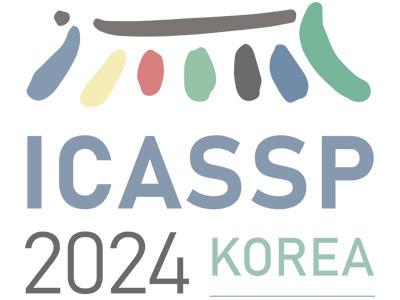
- Read more about Learning a Low-Rank Feature Representation: Achieving Better Trade-Off Between Stability and Plasticity in Continual Learning
- Log in to post comments
In continual learning, networks confront a trade-off between stability and plasticity when trained on a sequence of tasks. To bolster plasticity without sacrificing stability, we propose a novel training algorithm called LRFR. This approach optimizes network parameters in the null space of the past tasks’ feature representation matrix to guarantee the stability. Concurrently, we judiciously select only a subset of neurons in each layer of the network while training individual tasks to learn the past tasks’ feature representation matrix in low-rank.
LRFR_13Apr.pdf

- Categories:

- Read more about MLPs Compass: What is Learned When MLPs are Combined with PLMs?
- Log in to post comments
While Transformer-based pre-trained language models and their variants exhibit strong semantic representation capabilities, the question of comprehending the information gain derived from the additional components of PLMs remains an open question in this field. Motivated by recent efforts that prove Multilayer-Perceptrons (MLPs) modules achieving robust structural capture capabilities, even outperforming Graph Neural Networks (GNNs), this paper aims to quantify whether simple MLPs can further enhance the already potent ability of PLMs to capture linguistic information.
poster-MLP.pdf

- Categories:
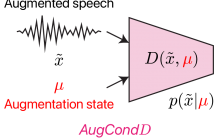
- Read more about Training Generative Adversarial Network-Based Vocoder with Limited Data Using Augmentation-Conditional Discriminator
- Log in to post comments
A generative adversarial network (GAN)-based vocoder trained with an adversarial discriminator is commonly used for speech synthesis because of its fast, lightweight, and high-quality characteristics. However, this data-driven model requires a large amount of training data incurring high data-collection costs. To address this issue, we propose an augmentation-conditional discriminator (AugCondD) that receives the augmentation state as input in addition to speech, thereby assessing input speech according to augmentation state, without inhibiting the learning of the original non-augmented distribution. Experimental results indicate that AugCondD improves speech quality under limited data conditions while achieving comparable speech quality under sufficient data conditions.
- Categories:
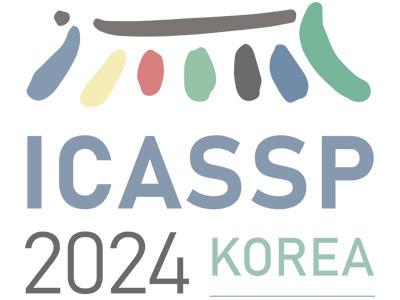
- Read more about Patient-Specific Modeling of Daily Activity Patterns for Unsupervised Detection of Psychotic and Non-Psychotic Relapses
- Log in to post comments
In this paper, we present our submission to the 2nd e-Prevention Grand Challenge hosted at ICASSP 2024. The objective posed in the challenge was to identify psychotic and non- psychotic relapses in patients using biosignals captured by wearable sensors. Our proposed solution is an unsupervised anomaly detection approach based on Transformers. We train individual models for each patient to predict the timestamps of biosignal measurements on non-relapse days, implicitly modeling normal daily routines.
- Categories:
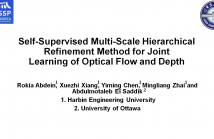
- Read more about SELF-SUPERVISED MULTI-SCALE HIERARCHICAL REFINEMENT METHOD FOR JOINT LEARNING OF OPTICAL FLOW AND DEPTH
- Log in to post comments
Recurrently refining the optical flow based on a single highresolution feature demonstrates high performance. We exploit the strength of this strategy to build a novel architecture for the joint learning of optical flow and depth. Our proposed architecture is improved to work in the case of training on unlabeled data, which is extremely challenging. The loss is computed for the iterations carried out over a single high-resolution feature, where the reconstruction loss fails to optimize the accuracy particularity in occluded regions.
- Categories:
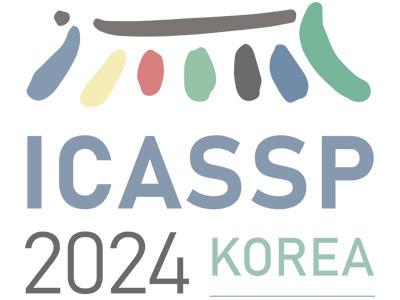
- Read more about Unravel Anomalies: An End-to-end Seasonal-Trend Decomposition Approach for Time Series Anomaly Detection
- Log in to post comments
Traditional Time-series Anomaly Detection (TAD) methods often struggle with the composite nature of complex time-series data and a diverse array of anomalies. We introduce TADNet, an end-to-end TAD model that leverages Seasonal-Trend Decomposition to link various types of anomalies to specific decomposition components, thereby simplifying the analysis of complex time-series and enhancing detection performance. Our training methodology, which includes pre-training on a synthetic dataset followed by fine-tuning, strikes a balance between effective decomposition and precise anomaly detection.
- Categories:
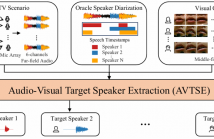
- Read more about THE MULTIMODAL INFORMATION BASED SPEECH PROCESSING (MISP) 2023 CHALLENGE: AUDIO-VISUAL TARGET SPEAKER EXTRACTION
- Log in to post comments
Previous Multimodal Information based Speech Processing (MISP) challenges mainly focused on audio-visual speech recognition (AVSR) with commendable success. However, the most advanced back-end recognition systems often hit performance limits due to the complex acoustic environments. This has prompted a shift in focus towards the Audio-Visual Target Speaker Extraction (AVTSE) task for the MISP 2023 challenge in ICASSP 2024 Signal Processing Grand Challenges.
misp2023ppt.pptx

- Categories:
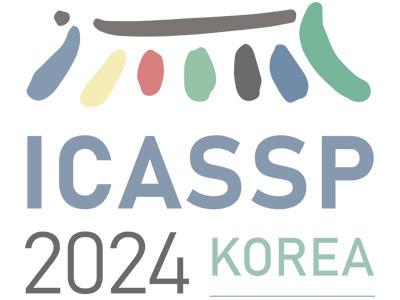
- Read more about LANGUAGE-FREE COMPOSITIONAL ACTION GENERATION VIA DECOUPLING REFINEMENT
- 1 comment
- Log in to post comments
Composing simple actions into complex actions is crucial yet challenging. Existing methods largely rely on language annotations to discern composable latent semantics, which is costly and labor-intensive. In this study, we introduce a novel framework to generate compositional actions without language auxiliaries. Our approach consists of three components: Action Coupling, Conditional Action Generation, and Decoupling Refinement. Action Coupling integrates two subactions to generate pseudo-training examples.
- Categories:
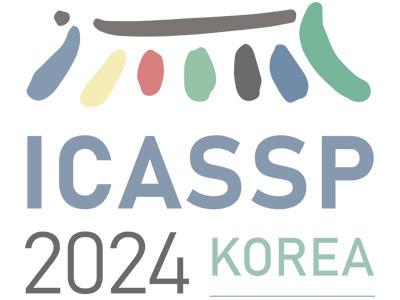
- Read more about LIGHTING IMAGE/VIDEO STYLE TRANSFER METHODS BY ITERATIVE CHANNEL PRUNING
- Log in to post comments
Deploying style transfer methods on resource-constrained devices is challenging, which limits their real-world applicability. To tackle this issue, we propose using pruning techniques to accelerate various visual style transfer methods. We argue that typical pruning methods may not be well-suited for style transfer methods and present an iterative correlation-based channel pruning (ICCP) strategy for encoder-transform-decoder-based image/video style transfer models.
- Categories:
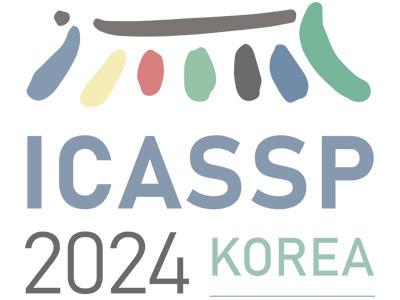
- Read more about SELF-SUPERVISED LEARNING FOR SLEEP STAGE CLASSIFICATION WITH TEMPORALAUGMENTATION AND FALSE NEGATIVE SUPPRESSION
- Log in to post comments
Self-supervised learning has been gaining attention in the field of sleep stage classification. It learns representations with unlabeled electroencephalography (EEG) signals, which alleviates the cost of labeling for specialists. However, most self-supervised approaches assume only the two augmented views from the same EEG sample is a positive pair, which suffers from the false negative problem. Therefore, we propose a new model named Temporal Augmentation and False Negative Suppression (TA-FNS) to solve the problem. Specifically, it first generates two augmented views for each EEG sample.
Poster.pdf

- Categories: