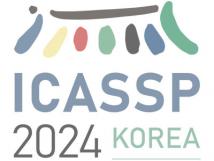
IEEE ICASSP 2024 - IEEE International Conference on Acoustics, Speech and Signal Processing (ICASSP) is the world’s largest and most comprehensive technical conference focused on signal processing and its applications. The IEEE ICASSP 2024 conference will feature world-class presentations by internationally renowned speakers, cutting-edge session topics and provide a fantastic opportunity to network with like-minded professionals from around the world. Visit the website.
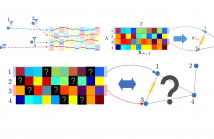
- Read more about Joint Signal Recovery and Graph Learning from Incomplete Time-Series
- Log in to post comments
Learning a graph from data is the key to taking advantage of graph signal processing tools. Most of the conventional algorithms for graph learning require complete data statistics, which might not be available in some scenarios. In this work, we aim to learn a graph from incomplete time-series observations. From another viewpoint, we consider the problem of semi-blind recovery of time-varying graph signals where the underlying graph model is unknown.
- Categories:
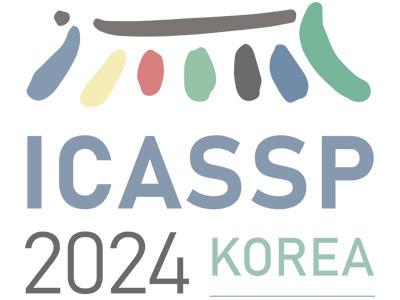
- Read more about A Parameterized Generative Adversarial Network Using Cyclic Projection for Explainable Medical Image Classification
- Log in to post comments
Although current data augmentation methods are successful to alleviate the data insufficiency, conventional augmentation are primarily intra-domain while advanced generative adversarial networks (GANs) generate images remaining uncertain, particularly in small-scale datasets. In this paper, we propose a parameterized GAN (ParaGAN) that effectively controls the changes of synthetic samples among domains and highlights the attention regions for downstream classification.
- Categories:
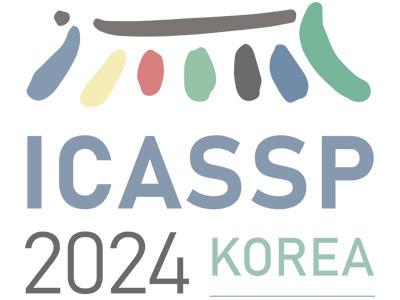
- Read more about LOW COST VARIABLE STEP-SIZE LMS WITH MAXIMUM SIMILARITY TO THE AFFINE PROJECTION ALGORITHM
- Log in to post comments
The LMS algorithm is widely employed in adaptive systems due to its robustness, simplicity, and reasonable performance. However, it is well known that this algorithm suffers from a slow convergence speed when dealing with colored reference signals. Numerous variants and alternative algorithms have been proposed to address this issue, though all of them entail an increase in computational cost. Among the proposed alternatives, the affine projection algorithm stands out. This algorithm has the peculiarity of starting from N data vectors of the reference signal.
- Categories:
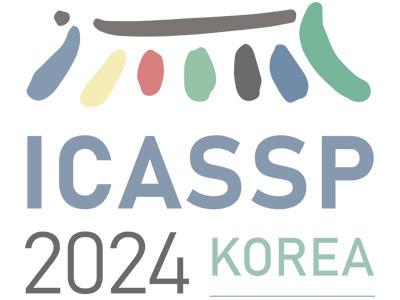
- Read more about Self-supervised Speaker Verification Employing a Novel Clustering Algorithm
- Log in to post comments
Clustering is an unsupervised learning technique, which leverages a large amount of unlabeled data to learn cluster-wise representations from speech. One of the most popular self-supervised techniques to train a speaker verification system is to predict the pseudo-labels using clustering algorithms and then train the speaker embedding network using the generated pseudo-labels in a discriminative manner. Therefore, pseudo-labels - driven self-supervised speaker verification systems' performance relies heavily on the accuracy of the adopted clustering algorithms.
- Categories:
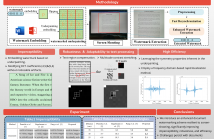
The widespread adoption of smartphones has introduced new challenges to document copyright protection, prompting the emergence of Screen-Shooting Resilient Document Watermarking (SSRDW) technology. In recent years, underpainting-based SSRDW techniques have proven to be highly effective. However, after careful study, we find that existing methods fail to simultaneously meet four essential criteria for SSRDW: high imperceptibility, strong
- Categories:
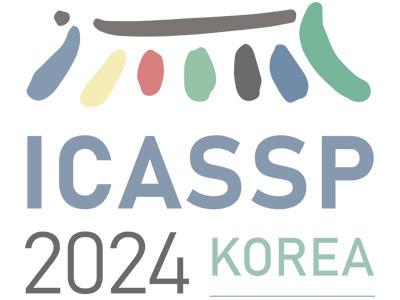
- Read more about FastGAT: Simple and Efficient Graph Attention Neural Network with Global-aware Adaptive Computational Node Attention
- Log in to post comments
Graph attention neural network (GAT) stands as a fundamental model within graph neural networks, extensively employed across various applications. It assigns different weights to different nodes for feature aggregation by comparing the similarity of features between nodes. However, as the amount and density of graph data increases, GAT's computational demands rise steeply. In response, we present FastGAT, a simpler and more efficient graph attention neural network with global-aware adaptive computational node attention.
- Categories:
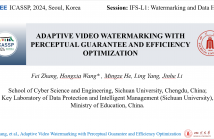
- Read more about ADAPTIVE VIDEO WATERMARKING WITH PERCEPTUAL GUARANTEE AND EFFICIENCY OPTIMIZATION
- Log in to post comments
Existing video watermarking embeds robust watermarks in each frame of the video for copyright protection and tracking. However, just as any content written on a blank paper is easily perceived, embedding watermarks in the texture-poor frames impairs imperceptibility. Common geometric attacks such as scaling and rotation pose a significant challenge to the existing video watermarking. Image watermarking based on moments is robust against geometric attacks.
- Categories:
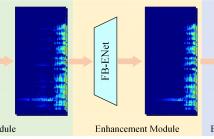
- Read more about RENet: A Time-Frequency Domain General Speech Restoration Network for ICASSP 2024 Speech Signal Improvement Challenge
- Log in to post comments
The ICASSP 2024 Speech Signal Improvement (SSI) Challenge seeks to address speech quality degradation problems in telecommunication systems. In this context, this paper proposes RENet, a time-frequency (T-F) domain method leveraging complex spectrum mapping to mitigate speech distortions. Specifically, the proposed RENet is a multi-stage network. First, TF-GridGAN was designed to recover the degraded speech with a generative adversarial network (GAN). Second, a full-band enhancement module was introduced to eliminate residual noises and artifacts existed in the output of TF-GridGAN.
- Categories:
- Read more about PERFORMANCE CONDITIONING FOR DIFFUSION-BASED MULTI-INSTRUMENT MUSIC SYNTHESIS
- Log in to post comments
Generating multi-instrument music from symbolic music representations is an important task in Music Information Retrieval (MIR). A central but still largely unsolved problem in this context is musically and acoustically informed control in the generation process. As the main contribution of this work, we propose enhancing control of multi-instrument synthesis by conditioning a generative model on a specific performance and recording environment, thus allowing for better guidance of timbre and style.
- Categories:
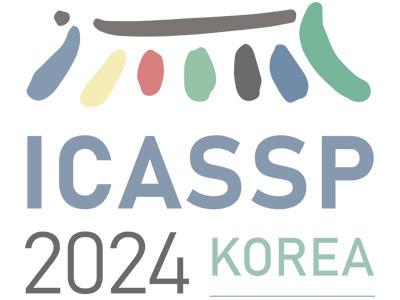
- Read more about Character Attribute Extraction from Movie Scripts using LLMs
- Log in to post comments
Narrative understanding is an integrative task of studying characters, plots, events, and relations in a story.
It involves natural language processing tasks such as named entity recognition and coreference resolution to identify the characters, semantic role labeling and argument mining to find character actions and events, information extraction and question answering to describe character attributes, causal analysis to relate different events, and summarization to find the main storyline.
- Categories: