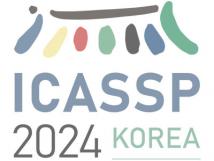
IEEE ICASSP 2024 - IEEE International Conference on Acoustics, Speech and Signal Processing (ICASSP) is the world’s largest and most comprehensive technical conference focused on signal processing and its applications. The IEEE ICASSP 2024 conference will feature world-class presentations by internationally renowned speakers, cutting-edge session topics and provide a fantastic opportunity to network with like-minded professionals from around the world. Visit the website.
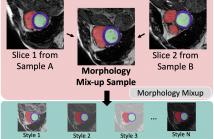
- Read more about MMS: Morphology-mixup Stylized Data Generation for Single Domain Generalization in Medical Image Segmentation
- Log in to post comments
Single-source domain generalization in medical image segmentation is a challenging yet practical task, as domain shift commonly exists across medical datasets.
Previous works have attempted to alleviate this problem through adversarial data augmentation or random-style transformation.
However, these approaches neither fully leverage medical information nor consider the morphological structure alterations.
To address these limitations and enhance the fidelity and diversity of the augmented data,
- Categories:
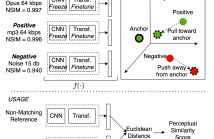
- Read more about NOMAD: Non-Matching Audio Distance
- Log in to post comments
This paper presents NOMAD (Non-Matching Audio Distance), a differentiable perceptual similarity metric that measures the distance of a degraded signal against non-matching references. The proposed method is based on learning deep feature embeddings via a triplet loss guided by the Neurogram Similarity Index Measure (NSIM) to capture degradation intensity. During inference, the similarity score between any two audio samples is computed through Euclidean distance of their embeddings. NOMAD is fully unsupervised and can be used in general perceptual audio tasks for audio analysis e.g.
- Categories:
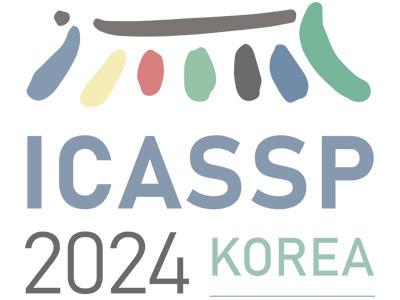
- Read more about LEARNING SPATIO-TEMPORAL RELATIONS WITH MULTI-SCALE INTEGRATED PERCEPTION FOR VIDEO ANOMALY DETECTION
- Log in to post comments
In weakly supervised video anomaly detection, it has been verified that anomalies can be biased by background noise. Previous works attempted to focus on local regions to exclude irrelevant information. However, the abnormal events in different scenes vary in size, and current methods struggle to consider local events of different scales concurrently. To this end, we propose a multi-scale integrated perception
- Categories:
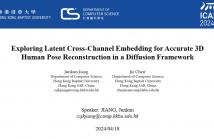
- Read more about Exploring Latent Cross-Channel Embedding for Accurate 3D Human Pose Reconstruction in a Diffusion Framework
- Log in to post comments
Monocular 3D human pose estimation poses significant challenges due to the inherent depth ambiguities that arise during the reprojection process from 2D to 3D. Conventional approaches that rely on estimating an over-fit projection matrix struggle to effectively address these challenges and often result in noisy outputs. Recent advancements in diffusion models have shown promise in incorporating structural priors to address reprojection ambiguities.
- Categories:
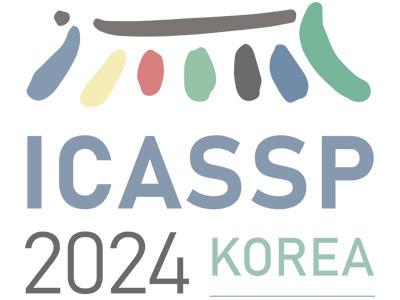
- Read more about A Machine-Learning Model for Detecting Depression, Anxiety, and Stress from Speech
- Log in to post comments
Predicting mental health conditions from speech has been widely explored in recent years. Most studies rely on a single sample from each subject to detect indicators of a particular disorder. These studies ignore two important facts: certain mental disorders tend to co-exist, and their severity tends to vary over time.
- Categories:
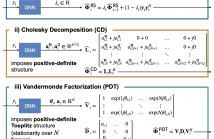
- Read more about Parameter Estimation Procedures for Deep Multi-Frame MVDR Filtering for Single-Microphone Speech Enhancement
- Log in to post comments
Aiming at exploiting temporal correlations across consecutive time frames in the short-time Fourier transform (STFT) domain, multi-frame algorithms for single-microphone speech enhancement have been proposed, which apply a complex- valued filter to the noisy STFT coefficients. Typically, the multi-frame filter coefficients are either estimated directly using deep neural networks or a certain filter structure is imposed, e.g., the multi-frame minimum variance distortionless response (MFMVDR) filter structure.
- Categories:
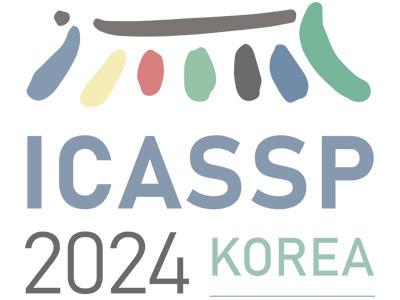
- Read more about DE NOVO MOLECULE GENERATION WITH GRAPH LATENT DIFFUSION MODEL
- Log in to post comments
De novo generation of molecules is a crucial task in drug discovery. The blossom of deep learning-based generative models, especially diffusion models, has brought forth promising advancements in de novo drug design by finding optimal molecules in a directed manner. However, due to the complexity of chemical space, existing approaches can only generate extremely small molecules. In this study, we propose a Graph Latent Diffusion Model (GLDM) that operates a diffusion model in the latent space modeled by a pretrained autoencoder.
- Categories:

- Read more about Self-supervised Speaker Verification with Adaptive Threshold and Hierarchical Training
- 1 comment
- Log in to post comments
This is a poster material of recent research accepted by IEEE ICASSP 2024.
Title: SELF-SUPERVISED SPEAKER VERIFICATION WITH ADAPTIVE THRESHOLD AND HIERARCHICAL TRAINING
For more inforamation, please check out the publication at IEEE Xplore:
https://ieeexplore.ieee.org/document/10448455
- Categories:
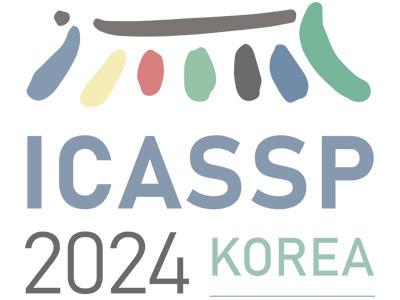
- Read more about Improving ASR Contextual Biasing using Guided Attention Loss
- Log in to post comments
In this paper, we propose a Guided Attention (GA) auxiliary training loss, which improves the effectiveness and robustness of automatic speech recognition (ASR) contextual biasing without introducing additional parameters. A common challenge in previous literature is that the word error rate (WER) reduction brought by contextual biasing diminishes as the number of bias phrases increases. To address this challenge, we employ a GA loss as an additional training objective besides the Transducer loss.
- Categories:
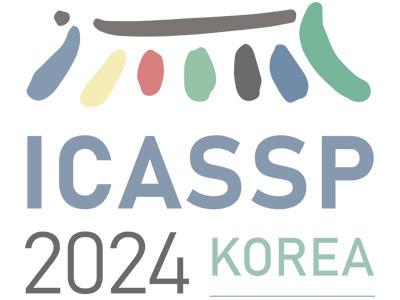
- Read more about FSPEN: An Ultra-Lightweight Network for Real Time Speech Enhancement
- Log in to post comments
Deep learning-based speech enhancement methods have shown promising result in recent years. However, in practical applications, the model size and computational complexity are important factors that limit their use in end-products. Therefore, in products that require real-time speech enhancement with limited resources, such as TWS headsets, hearing aids, IoT devices, etc., ultra-lightweight models are necessary. In this paper, an ultra-lightweight network FSPEN is proposed for real-time speech enhancement task.
poster.pptx

- Categories: