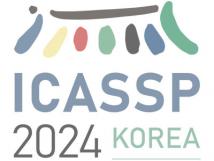
IEEE ICASSP 2024 - IEEE International Conference on Acoustics, Speech and Signal Processing (ICASSP) is the world’s largest and most comprehensive technical conference focused on signal processing and its applications. The IEEE ICASSP 2024 conference will feature world-class presentations by internationally renowned speakers, cutting-edge session topics and provide a fantastic opportunity to network with like-minded professionals from around the world. Visit the website.
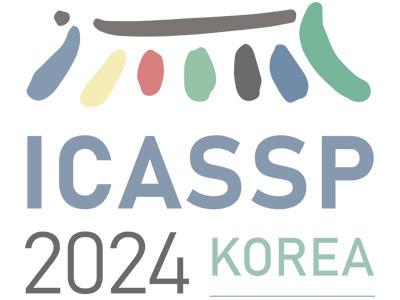
- Read more about TB-RESNET: BRIDGING THE GAP FROM TDNN TO RESNET IN AUTOMATIC SPEAKER VERIFICATION WITH TEMPORAL-BOTTLENECK ENHANCEMENT
- Log in to post comments
This paper focuses on the transition of automatic speaker verification systems from time delay neural networks (TDNN) to ResNet-based networks. TDNN-based systems use a statistics pooling layer to aggregate temporal information which is suitable for two-dimensional tensors. Even though ResNet-based models produce three-dimensional tensors, they continue to incorporate the statistics pooling layer.
- Categories:
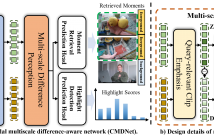
- Read more about Cross-modal Multiscale Difference-aware Network for Joint Moment Retrieval and Highlight Detection
- Log in to post comments
Since the goals of both Moment Retrieval (MR) and Highlight Detection (HD) are to quickly obtain the required content from the video according to user needs, several works have attempted to take advantage of the commonality between both tasks to design transformer-based networks for joint MR and HD. Although these methods achieve impressive performance, they still face some problems: \textbf{a)} Semantic gaps across different modalities. \textbf{b)} Various durations of different query-relevant moments and highlights. \textbf{c)} Smooth transitions among diverse events.
CMDNet_04_13.pdf

- Categories:
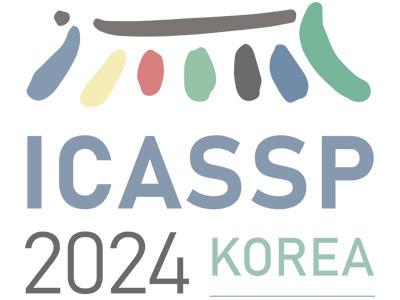
- Read more about Less peaky and more accurate CTC forced alignment by label priors
- 1 comment
- Log in to post comments
Connectionist temporal classification (CTC) models are known to have peaky output distributions. Such behavior is not a problem for automatic speech recognition (ASR), but it can cause inaccurate forced alignments (FA), especially at finer granularity, e.g., phoneme level. This paper aims at alleviating the peaky behavior for CTC and improve its suitability for forced alignment generation, by leveraging label priors, so that the scores of alignment paths containing fewer blanks are boosted and maximized during training.
aligner.pdf

- Categories:
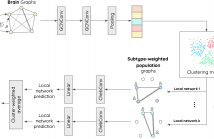
- Read more about Subtype-specific biomarkers of Alzheimer's disease from anatomical and functional connectomes via graph neural networks
- Log in to post comments
Heterogeneity is present in Alzheimer’s disease (AD), making it challenging to study. To address this, we propose a graph neural network (GNN) approach to identify disease subtypes from magnetic resonance imaging (MRI) and functional MRI (fMRI) scans. Subtypes are identified by encoding the patients’ scans in brain graphs (via cortical similarity networks) and clustering the representations learnt by the GNN.
- Categories:
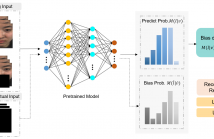
- Read more about CAUSALLY UNCOVERING BIAS IN VIDEO MICRO-EXPRESSION RECOGNITION
- Log in to post comments
Detecting microexpressions presents formidable challenges, primarily due to their fleeting nature and the limited diversity in existing datasets. Our studies find that these datasets exhibit a pronounced bias towards specific ethnicities and suffer from significant imbalances in terms of both class and gender representation among the samples. These disparities create fertile ground for various biases to permeate deep learning models, leading to skewed results and inadequate portrayal of specific demographic groups.
- Categories:
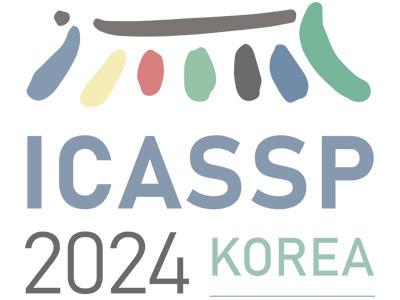
Deep learning-based methods provide remarkable performance in a number of computational imaging problems. Examples include end-to-end trained networks that map measurements to unknown signals, plug-and-play (PnP) methods that use pretrained denoisers as image prior, and model-based unrolled networks that train artifact removal blocks. Many of these methods lack robustness and fail to generalize with distribution shifts in data, measurements, and noise. In this paper, we present a simple framework to perform domain adaptation as data and measurement distribution shifts.
- Categories:
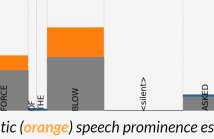
- Read more about [Poster] Crowdsourced and Automatic Speech Prominence Estimation
- Log in to post comments
The prominence of a spoken word is the degree to which an average native listener perceives the word as salient or emphasized relative to its context. Speech prominence estimation is the process of assigning a numeric value to the prominence of each word in an utterance. These prominence labels are useful for linguistic analysis, as well as training automated systems to perform emphasis-controlled text-to-speech or emotion recognition. Manually annotating prominence is time-consuming and expensive, which motivates the development of automated methods for speech prominence estimation.
- Categories:
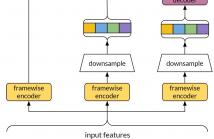
- Read more about [Paper] Crowdsourced and Automatic Speech Prominence Estimation
- Log in to post comments
The prominence of a spoken word is the degree to which an average native listener perceives the word as salient or emphasized relative to its context. Speech prominence estimation is the process of assigning a numeric value to the prominence of each word in an utterance. These prominence labels are useful for linguistic analysis, as well as training automated systems to perform emphasis-controlled text-to-speech or emotion recognition. Manually annotating prominence is time-consuming and expensive, which motivates the development of automated methods for speech prominence estimation.
- Categories:
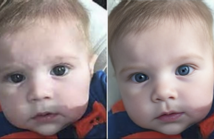
Presentation attacks on biometric systems have long created significant security risks. The increase in the adoption of age verification systems, which ensure that only age-appropriate content is consumed online, raises the question of vulnerability of such systems to replay presentation attacks. In this paper, we analyze the vulnerability of face age verification to simple replay attacks and assess whether presentation attack detection (PAD) systems created for biometrics can be effective at detecting similar attacks on age verification.
- Categories:
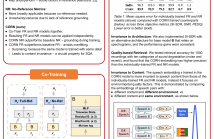
- Read more about CORN: Co-Trained Full- And No-Reference Speech Quality Assessment
- Log in to post comments
Perceptual evaluation constitutes a crucial aspect of various audio-processing tasks. Full reference (FR) or similarity-based metrics rely on high-quality reference recordings, to which lower-quality or corrupted versions of the recording may be compared for evaluation. In contrast, no-reference (NR) metrics evaluate a recording without relying on a reference. Both the FR and NR approaches exhibit advantages and drawbacks relative to each other.
2310.09388.pdf

- Categories: