- Transducers
- Spatial and Multichannel Audio
- Source Separation and Signal Enhancement
- Room Acoustics and Acoustic System Modeling
- Network Audio
- Audio for Multimedia
- Audio Processing Systems
- Audio Coding
- Audio Analysis and Synthesis
- Active Noise Control
- Auditory Modeling and Hearing Aids
- Bioacoustics and Medical Acoustics
- Music Signal Processing
- Loudspeaker and Microphone Array Signal Processing
- Echo Cancellation
- Content-Based Audio Processing
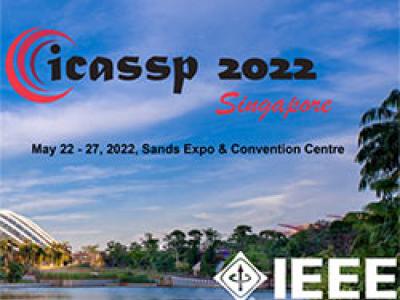
- Read more about HYBRID ATTENTION-BASED PROTOTYPICAL NETWORKS FOR FEW-SHOT SOUND CLASSIFICATION
- Log in to post comments
In recent years, prototypical networks have been widely used
in many few-shot learning scenarios. However, as a metric-
based learning method, their performance often degrades in
the presence of bad or noisy embedded features, and outliers
in support instances. In this paper, we introduce a hybrid at-
tention module and combine it with prototypical networks for
few-shot sound classification. This hybrid attention module
consists of two blocks: a feature-level attention block, and
- Categories:
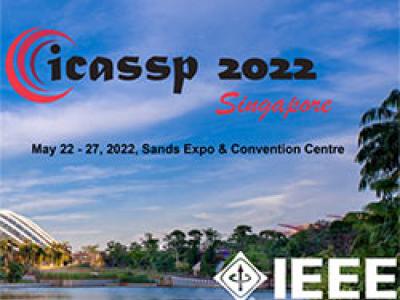
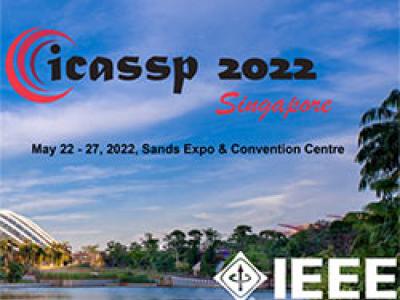
- Read more about Conformer-Based Self-Supervised Learning for Non-Speech Audio Tasks
- Log in to post comments
Representation learning from unlabeled data has been of major interest in artificial intelligence research. While self-supervised speech representation learning has been popular in the speech research community, very few works have comprehensively analyzed audio representation learning for non-speech audio tasks. In this paper, we propose a self-supervised audio representation learning method and apply it to a variety of downstream non-speech audio tasks.
- Categories:
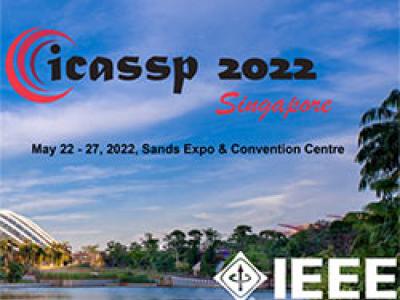
- Read more about TOWARDS FASTER CONTINUOUS MULTI-CHANNEL HRTF MEASUREMENTS BASED ON LEARNING SYSTEM MODELS
- Log in to post comments
Measuring personal head-related transfer functions (HRTFs) is essential in binaural audio. Personal HRTFs are not only required for binaural rendering and for loudspeaker-based binaural reproduction using crosstalk cancellation, but they also serve as a basis for data-driven HRTF individualization techniques and psychoacoustic experiments. Although many attempts have been made to expedite HRTF measurements, the rotational velocities in today’s measurement systems remain lower than those in natural head movements.
- Categories:
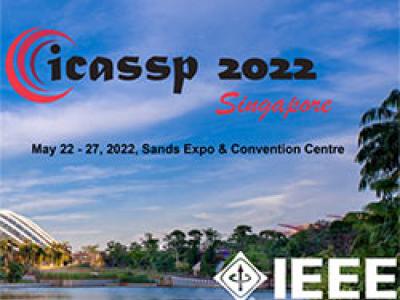
- Read more about AECMOS: A speech quality assessment metric for echo impairment
- Log in to post comments
Traditionally, the quality of acoustic echo cancellers is evaluated using intrusive speech quality assessment measures such as ERLE \cite{g168} and PESQ \cite{p862}, or by carrying out subjective laboratory tests. Unfortunately, the former are not well correlated with human subjective measures, while the latter are time and resource consuming to carry out. We provide a new tool for speech quality assessment for echo impairment which can be used to evaluate the performance of acoustic echo cancellers.
- Categories:
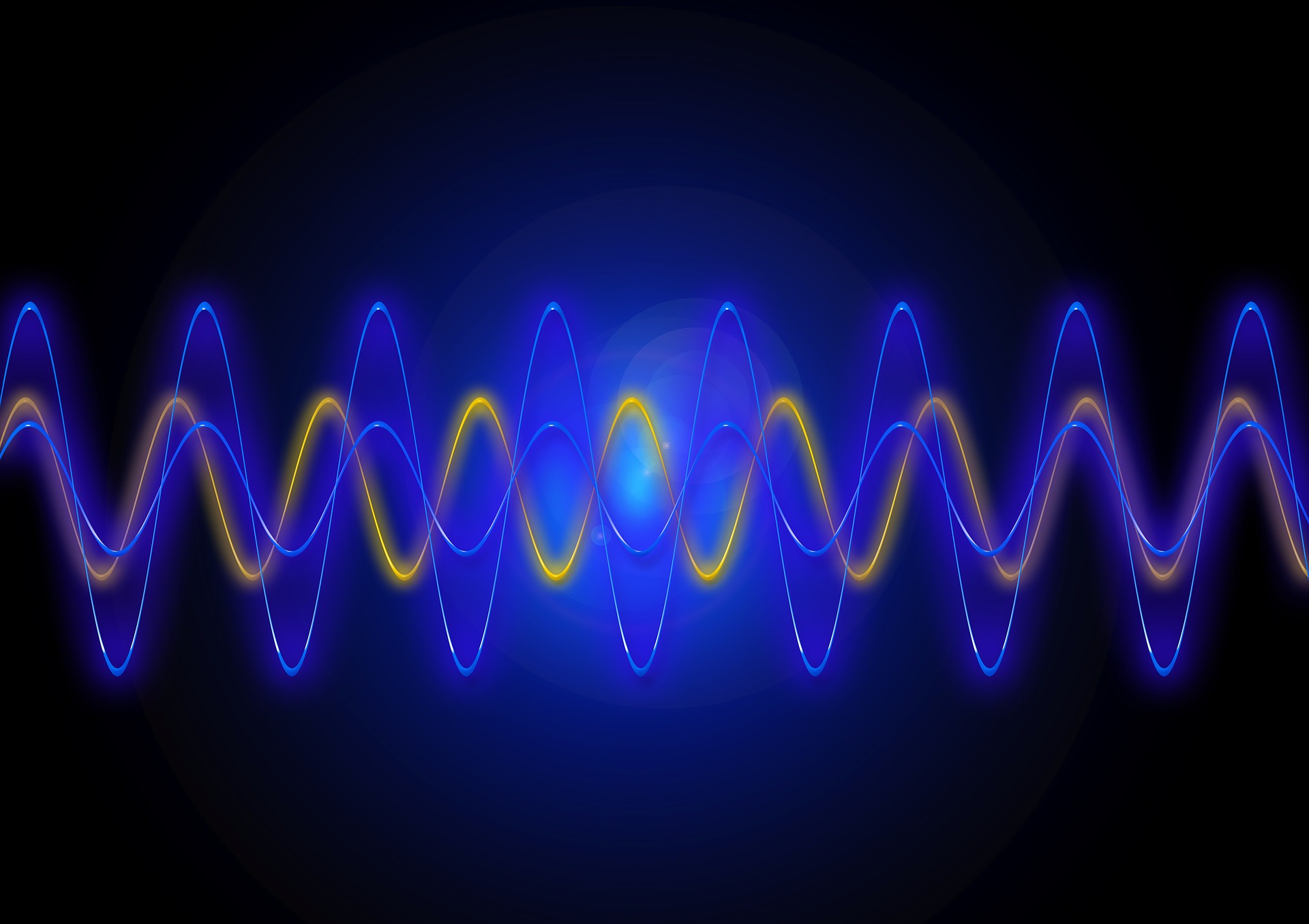
- Read more about ICASSP 2022 DEEP NOISE SUPPRESSION CHALLENGE
- Log in to post comments
- Categories:
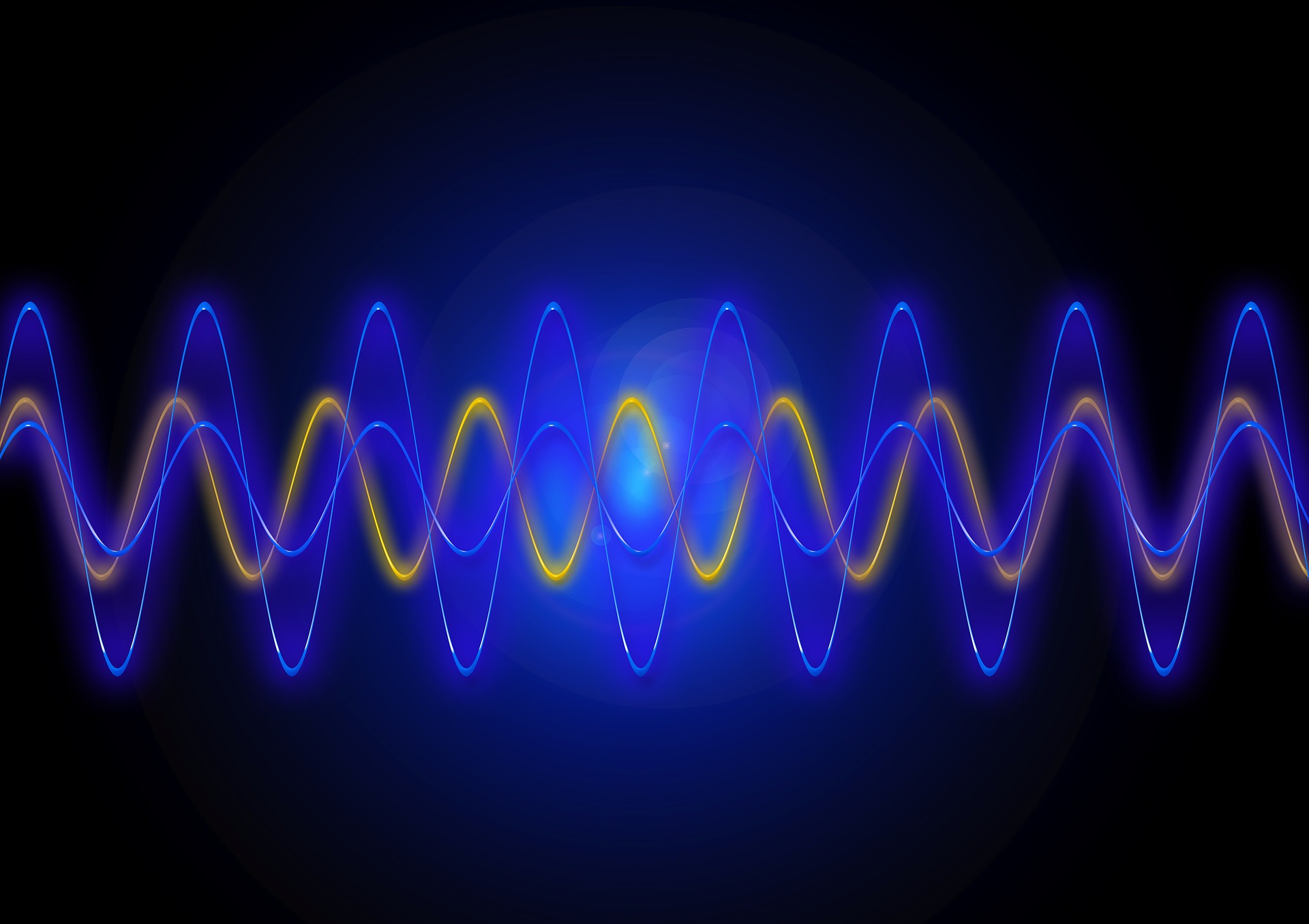
- Read more about ICASSP 2022 DEEP NOISE SUPPRESSION CHALLENGE
- Log in to post comments
- Categories:
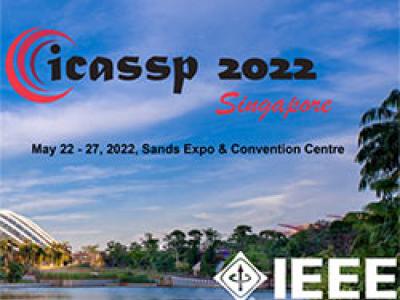
- Read more about Modeling Beats And Downbeats With A Time-frequency Transformer
- Log in to post comments
- Categories: