- Transducers
- Spatial and Multichannel Audio
- Source Separation and Signal Enhancement
- Room Acoustics and Acoustic System Modeling
- Network Audio
- Audio for Multimedia
- Audio Processing Systems
- Audio Coding
- Audio Analysis and Synthesis
- Active Noise Control
- Auditory Modeling and Hearing Aids
- Bioacoustics and Medical Acoustics
- Music Signal Processing
- Loudspeaker and Microphone Array Signal Processing
- Echo Cancellation
- Content-Based Audio Processing
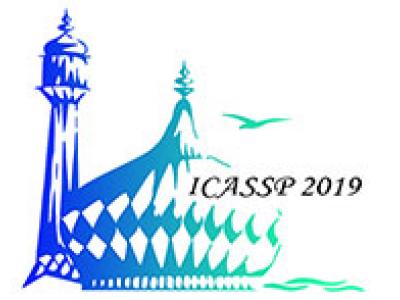
- Read more about Conditional Teacher-Student Learning
- Log in to post comments
The teacher-student (T/S) learning has been shown to be effective for a variety of problems such as domain adaptation and model compression. One shortcoming of the T/S learning is that a teacher model, not always perfect, sporadically produces wrong guidance in form of posterior probabilities that misleads the student model towards a suboptimal performance.
- Categories:
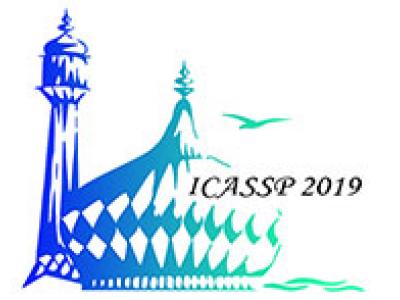
- Read more about Universal Acoustic Using Neural Mixture Models
- Log in to post comments
UAM_v3.pdf

- Categories:
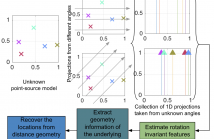
In this paper, we study a 2D tomography problem for point source models with random unknown view angles. Rather than recovering the projection angles, we reconstruct the model through a set of rotation-invariant features that are estimated from the projection data. For a point source model, we show that these features reveal geometric information about the model such as the radial and pairwise distances. This establishes a connection between unknown view tomography and unassigned distance geometry problem (uDGP).
- Categories:
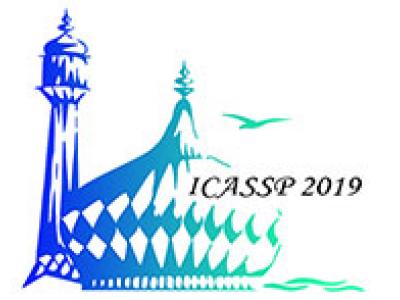
- Read more about ONLINE ESTIMATION AND SMOOTHING OF A TARGET TRAJECTORY IN MIXED STATIONARY/MOVING CONDITIONS
- Log in to post comments
A novel maximum likelihood trajectory estimation algorithm for targets in mixed stationary/moving conditions is presented. The proposed approach is able to estimate position and velocity of the target over arbitrary complex trajectories, while explicitly taking into account the possibility of stop&go motion. Moreover, a novel trajectory reconstruction method based on the theory of Bezier curve is developed for online smoothing of the trajectory, which keeps the advantages of Bayesian smoothing while introducing only a fixed lag in the estimation process.
poster.pdf

- Categories:
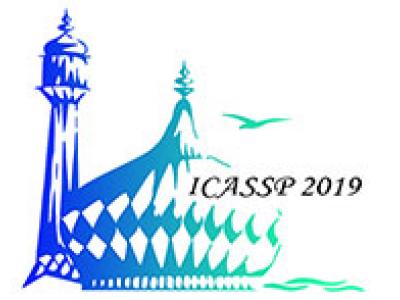
Increasingly, post-secondary instructors are incorporating innovative teaching practices into their classrooms to improve student learning outcomes. In order to assess the effect of these techniques, it is helpful to quantify the types of activity being conducted in the classroom. Unfortunately, self-reporting is unreliable and manual annotation is tedious and scales poorly.
- Categories:
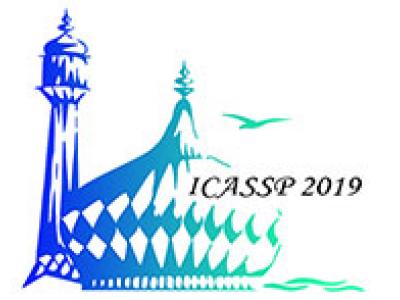
- Read more about Methodical Design and Trimming of Deep Learning Networks: Enhancing External BP learning with Internal Omnipresent-Supervision Training Paradigm
- Log in to post comments
Back-propagation (BP) is now a classic learning paradigm
whose source of supervision is exclusively from the external
(input/output) nodes. Consequently, BP is easily vulnerable
to curse-of-depth in (very) Deep Learning Networks
(DLNs). This prompts us to advocate Internal Neuron’s
Learnablility (INL) with (1)internal teacher labels (ITL); and
(2)internal optimization metrics (IOM) for evaluating hidden
layers/nodes. Conceptually, INL is a step beyond the notion
of Internal Neuron’s Explainablility (INE), championed by
ICASSP2019.pdf

- Categories:
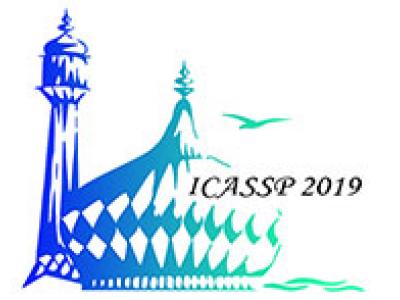
- Read more about Frequency-selective hybrid precoding and combining for mmWave MIMO systems with per-antenna power constraints
- Log in to post comments
Configuring hybrid precoders and combiners is the main challenge to be solved to operate at millimeter wave (mmWave) frequencies. The use of hybrid architectures imposse hardware constraints on the analog precoder that need to be carefully dealt with. In this paper, we develop hybrid precoders and combiners aiming at minimizing the Euclidean distance with respect to the approximate all-digital precoders and combiners maximizing the spectral efficiency under per-antenna power constraints.
- Categories:
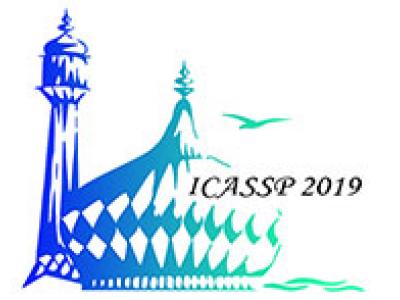
- Categories:
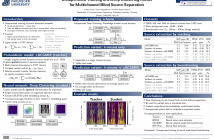
- Read more about UNSUPERVISED TRAINING OF A DEEP CLUSTERING MODEL FOR MULTICHANNEL BLIND SOURCE SEPARATION
- Log in to post comments
We propose a training scheme to train neural network-based source separation algorithms from scratch when parallel clean data is unavailable. In particular, we demonstrate that an unsupervised spatial clustering algorithm is sufficient to guide the training of a deep clustering system. We argue that previous work on deep clustering requires strong supervision and elaborate on why this is a limitation.
- Categories: