- Transducers
- Spatial and Multichannel Audio
- Source Separation and Signal Enhancement
- Room Acoustics and Acoustic System Modeling
- Network Audio
- Audio for Multimedia
- Audio Processing Systems
- Audio Coding
- Audio Analysis and Synthesis
- Active Noise Control
- Auditory Modeling and Hearing Aids
- Bioacoustics and Medical Acoustics
- Music Signal Processing
- Loudspeaker and Microphone Array Signal Processing
- Echo Cancellation
- Content-Based Audio Processing
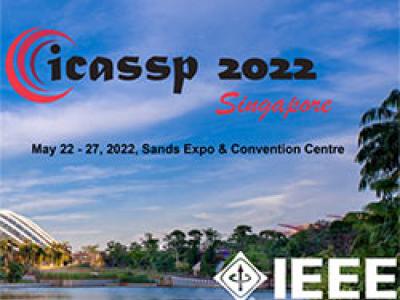
- Read more about A ROBUST DEEP AUDIO SPLICING DETECTION METHOD VIA SINGULARITY DETECTION FEATURE
- Log in to post comments
There are many methods for detecting forged audio produced by conversion and synthesis. However, as a simpler method of forgery, splicing has not attracted widespread attention.
Based on the characteristic that the tampering operation will cause singularities at high-frequency components, we propose a high-frequency singularity detection feature obtained
- Categories:
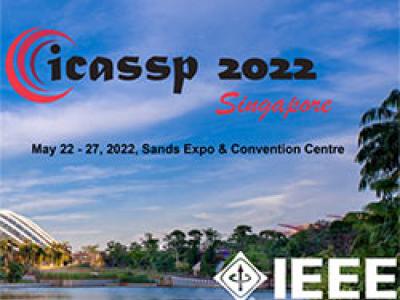
- Read more about A ROBUST DEEP AUDIO SPLICING DETECTION METHOD VIA SINGULARITY DETECTION FEATURE
- Log in to post comments
There are many methods for detecting forged audio produced by conversion and synthesis. However, as a simpler method of forgery, splicing has not attracted widespread attention.
Based on the characteristic that the tampering operation will cause singularities at high-frequency components, we propose a high-frequency singularity detection feature obtained
- Categories:
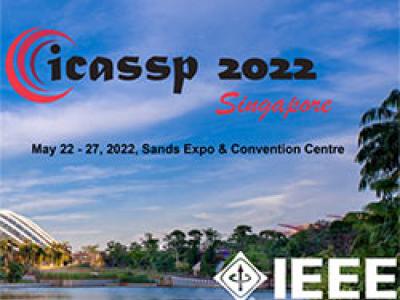
- Read more about INTERPRETING INTERMEDIATE CONVOLUTIONAL LAYERS IN UNSUPERVISED ACOUSTIC WORD CLASSIFICATION
- Log in to post comments
Understanding how deep convolutional neural networks classify data has been subject to extensive research. This paper proposes a technique to visualize and interpret intermediate layers of unsupervised deep convolutional networks by averaging over individual feature maps in each convolutional layer and inferring underlying distributions of words with non-linear regression techniques. A GAN-based architecture (ciwGAN [1]) that includes a Generator, a Discriminator, and a classifier was trained on unlabeled sliced lexical items from TIMIT.
- Categories:
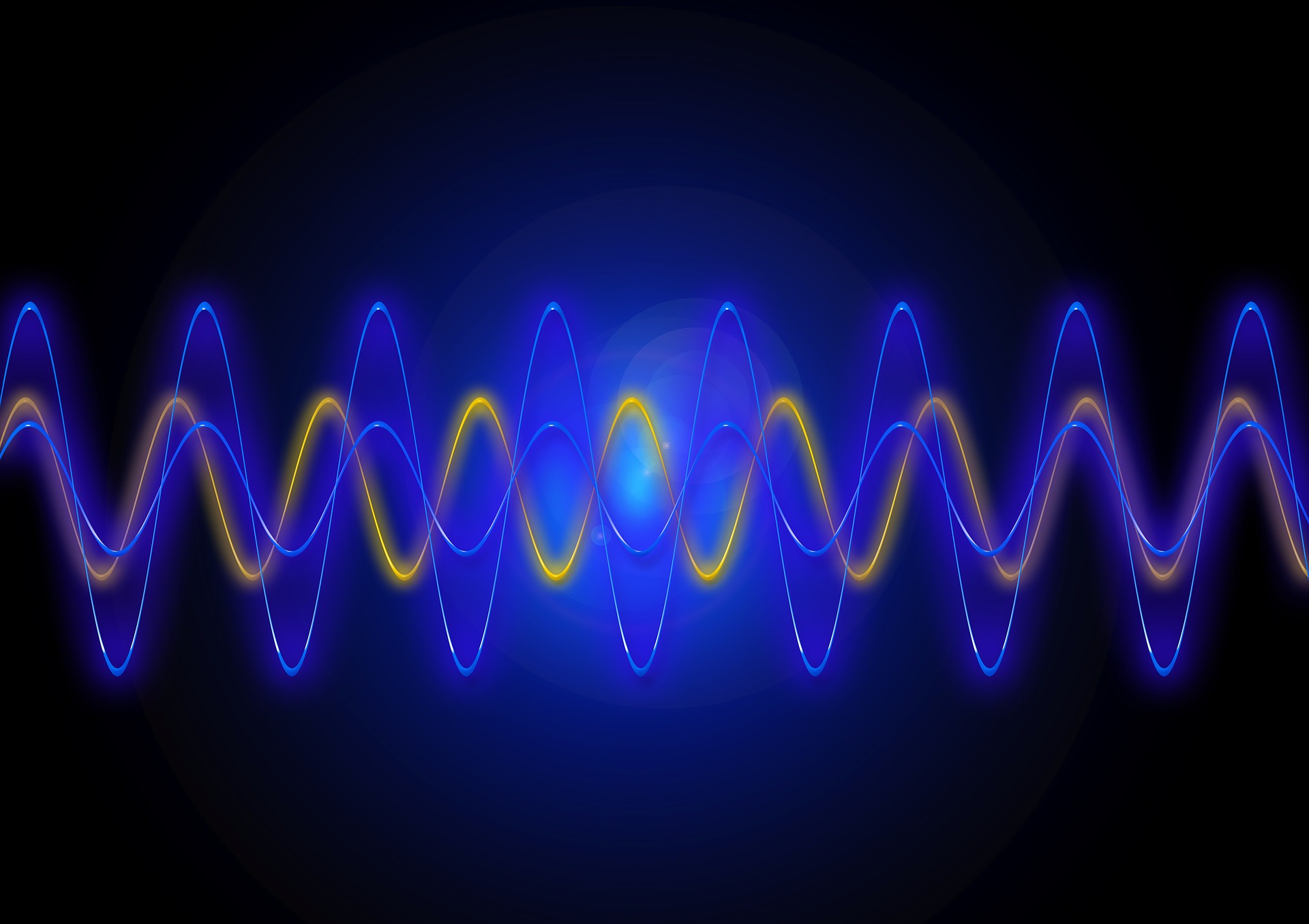
- Read more about Don’t Separate, Learn to Remix: End-to-End Neural Remixing with Joint Optimization
- Log in to post comments
- Categories:
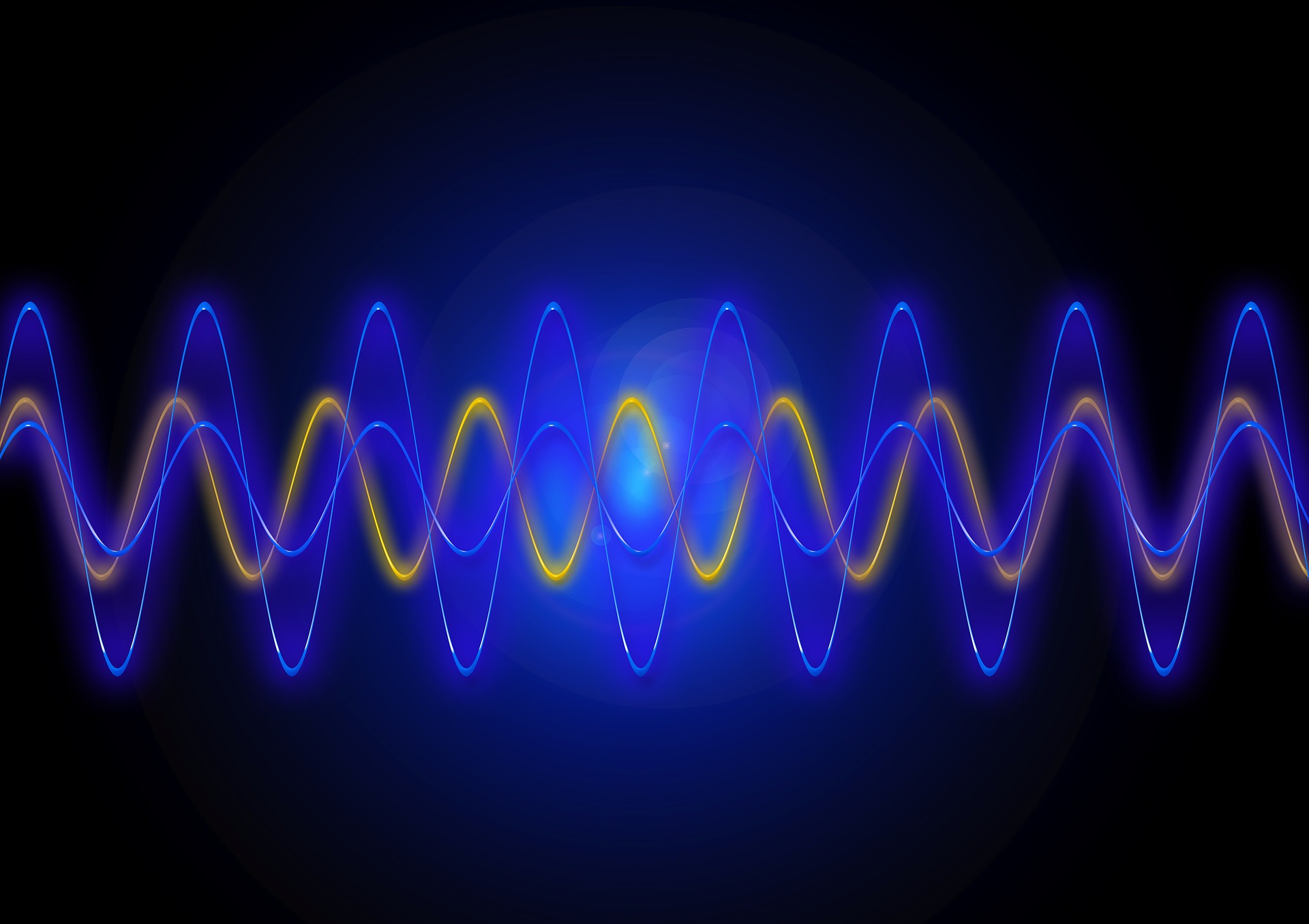
- Read more about Upmixing via Style Transfer: A Variational Autoencoder for Disentangling Spatial Images and Musical Content
- Log in to post comments
- Categories:
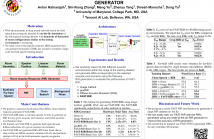
- Read more about FAST-RIR: FAST NEURAL DIFFUSE ROOM IMPULSE RESPONSE GENERATOR
- Log in to post comments
We present a neural-network-based fast diffuse room impulse response generator (FAST-RIR) for generating room impulse responses (RIRs) for a given acoustic environment. Our FAST-RIR takes rectangular room dimensions, listener and speaker positions, and reverberation time as inputs and generates specular and diffuse reflections for a given acoustic environment. Our FAST-RIR is capable of generating RIRs for a given input reverberation time with an average error of 0.02s.
- Categories:
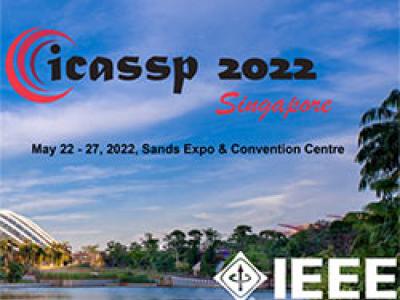
- Read more about Generalization Ability of MOS Prediction Networks
- Log in to post comments
Automatic methods to predict listener opinions of synthesized speech remain elusive since listeners, systems being evaluated, characteristics of the speech, and even the instructions given and the rating scale all vary from test to test. While automatic predictors for metrics such as mean opinion score (MOS) can achieve high prediction accuracy on samples from the same test, they typically fail to generalize well to new listening test contexts.
- Categories:
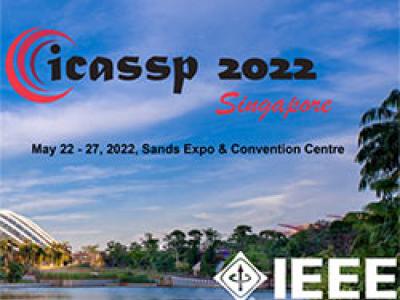
Named Entity Recognition (NER) from speech is among Spoken Language Understanding (SLU) tasks, aiming to extract semantic information from the speech signal. NER from speech is usually made through a two-step pipeline that consists of (1) processing the audio using an Automatic Speech Recognition (ASR) system and (2) applying an NER tagger to the ASR outputs. Recent works have shown the capability of the End-to-End (E2E) approach for NER from English and French speech, which is essentially entity-aware ASR.
Poster.pdf

- Categories:
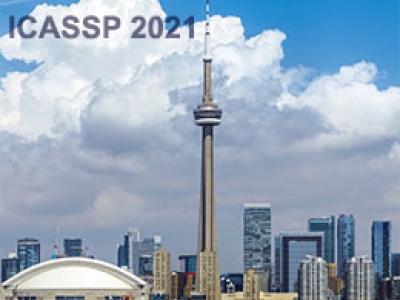
- Read more about PPT- Room Impulse Response Interpolation from a Sparse Set of Measurements Using a Modal Architecture
- Log in to post comments
In augmented reality applications, where room geometries and material properties are not readily available, it is desirable to get a representation of the sound field in a room from a limited set of available room impulse response measurements. In this paper, we propose a novel method for 2D interpolation of room modes from a sparse set of RIR measurements that are non-uniformly sampled within a space. We first obtain the mode parameters of a measured room.
ICASSP21_ppt_1473.pdf

- Categories:
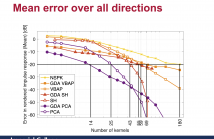
- Read more about Processing pipelines for efficient, physically-accurate simulation of microphone array signals in dynamic sound scenes
- Log in to post comments
Multichannel acoustic signal processing is predicated on the fact that the interchannel relationships between the received signals can be exploited to infer information about the acoustic scene. Recently there has been increasing interest in algorithms which are applicable in dynamic scenes, where the source(s) and/or microphone array may be moving. Simulating such scenes has particular challenges which are exacerbated when real-time, listener-in-the-loop evaluation of algorithms is required.
- Categories: