- Transducers
- Spatial and Multichannel Audio
- Source Separation and Signal Enhancement
- Room Acoustics and Acoustic System Modeling
- Network Audio
- Audio for Multimedia
- Audio Processing Systems
- Audio Coding
- Audio Analysis and Synthesis
- Active Noise Control
- Auditory Modeling and Hearing Aids
- Bioacoustics and Medical Acoustics
- Music Signal Processing
- Loudspeaker and Microphone Array Signal Processing
- Echo Cancellation
- Content-Based Audio Processing
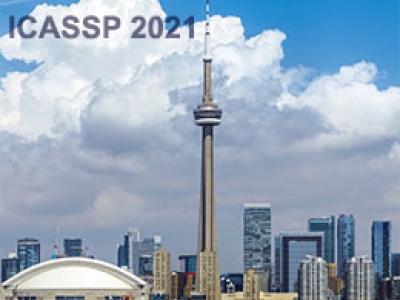
- Read more about JOINT MASKED CPC AND CTC TRAINING FOR ASR
- Log in to post comments
Self-supervised learning (SSL) has shown promise in learning representations of audio that are useful for automatic speech recognition (ASR). But, training SSL models like wav2vec~2.0 requires a two-stage pipeline. In this paper we demonstrate a single-stage training of ASR models that can utilize both unlabeled and labeled data. During training, we alternately minimize two losses: an unsupervised masked Contrastive Predictive Coding (CPC) loss and the supervised audio-to-text alignment loss Connectionist Temporal Classification (CTC).
- Categories:
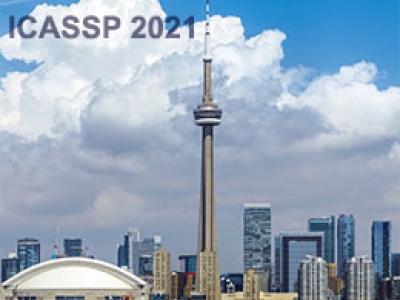
- Read more about VOWEL NON-VOWEL BASED SPECTRAL WARPING AND TIME SCALE MODIFICATION FOR IMPROVEMENT IN CHILDREN’S ASR
- Log in to post comments
Acoustic differences between children’s and adults’ speech causes the degradation in the automatic speech recognition system performance when system trained on adults’ speech and tested on children’s speech. The key acoustic mismatch factors are formant, speaking rate, and pitch. In this paper, we proposed a linear prediction based spectral warping method by using the knowledge of vowel and non-vowel regions in speech signals to mitigate the formant frequencies differences between child and adult speakers.
- Categories:
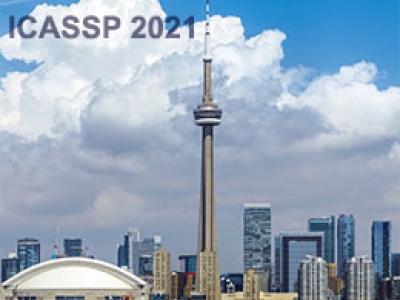
- Read more about Continuous Speech Separation with Conformer
- Log in to post comments
- Categories:
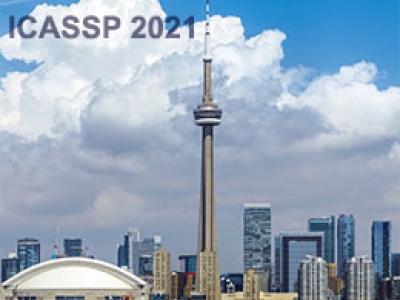
- Read more about ZERO-SHOT AUDIO CLASSIFICATION WITH FACTORED LINEAR AND NONLINEAR ACOUSTIC-SEMANTIC PROJECTIONS
- Log in to post comments
In this paper, we study zero-shot learning in audio classification through factored linear and nonlinear acoustic-semantic projections between audio instances and sound classes. Zero-shot learning in audio classification refers to classification problems that aim at recognizing audio instances of sound classes, which have no available training data but only semantic side information. In this paper, we address zero-shot learning by employing factored linear and nonlinear acoustic-semantic projections.
- Categories:
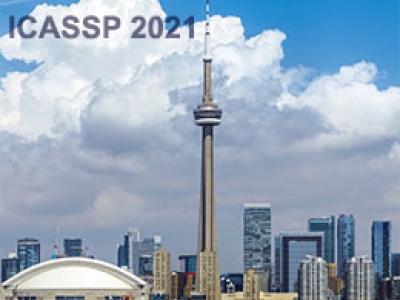
- Read more about A TWO-STAGE APPROACH TO DEVICE-ROBUST ACOUSTIC SCENE CLASSIFICATION
- Log in to post comments
To improve device robustness, a highly desirable key feature of a competitive data-driven acoustic scene classification (ASC) system, a novel two-stage system based on fully convolutional neural networks (CNNs) is proposed. Our two-stage system leverages on an ad-hoc score combination based on two CNN classifiers: (i) the first CNN classifies acoustic inputs into one of three broad classes, and (ii) the second CNN classifies the same inputs into one of ten finergrained classes.
- Categories:
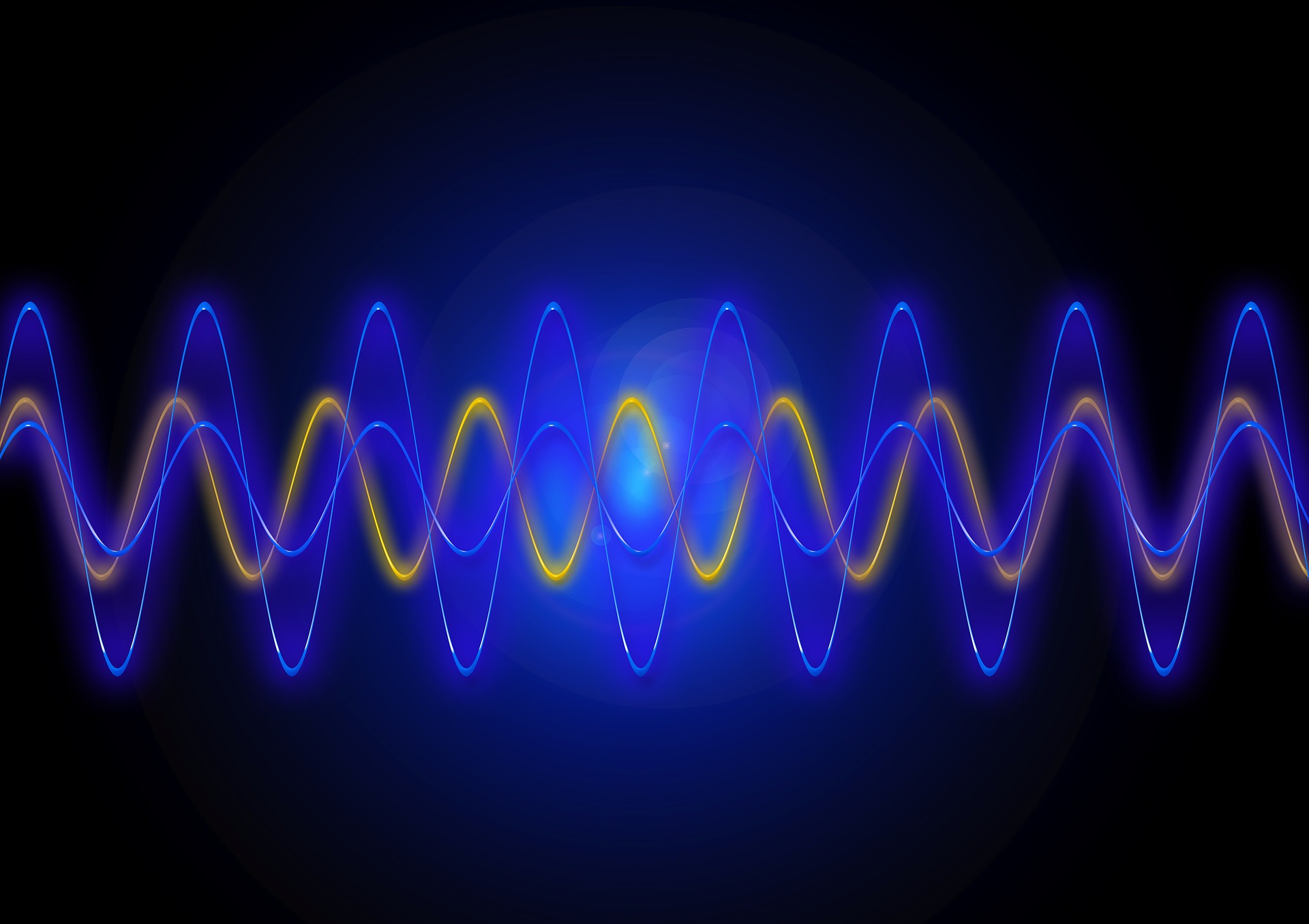
- Read more about Emotion Controllable Speech Synthesis Using Emotion-unlabeled Dataset With The Assistance Of Cross-domain Speech Emotion Recognition
- Log in to post comments
Neural text-to-speech (TTS) approaches generally require a huge number of high quality speech data, which makes it difficult to obtain such a dataset with extra emotion labels. In this paper, we propose a novel approach for emotional TTS synthesis on a TTS dataset without emotion labels. Specifically, our proposed method consists of a cross-domain speech emotion recognition (SER) model and an emotional TTS model. Firstly, we train the cross-domain SER model on both SER and TTS datasets.
- Categories:
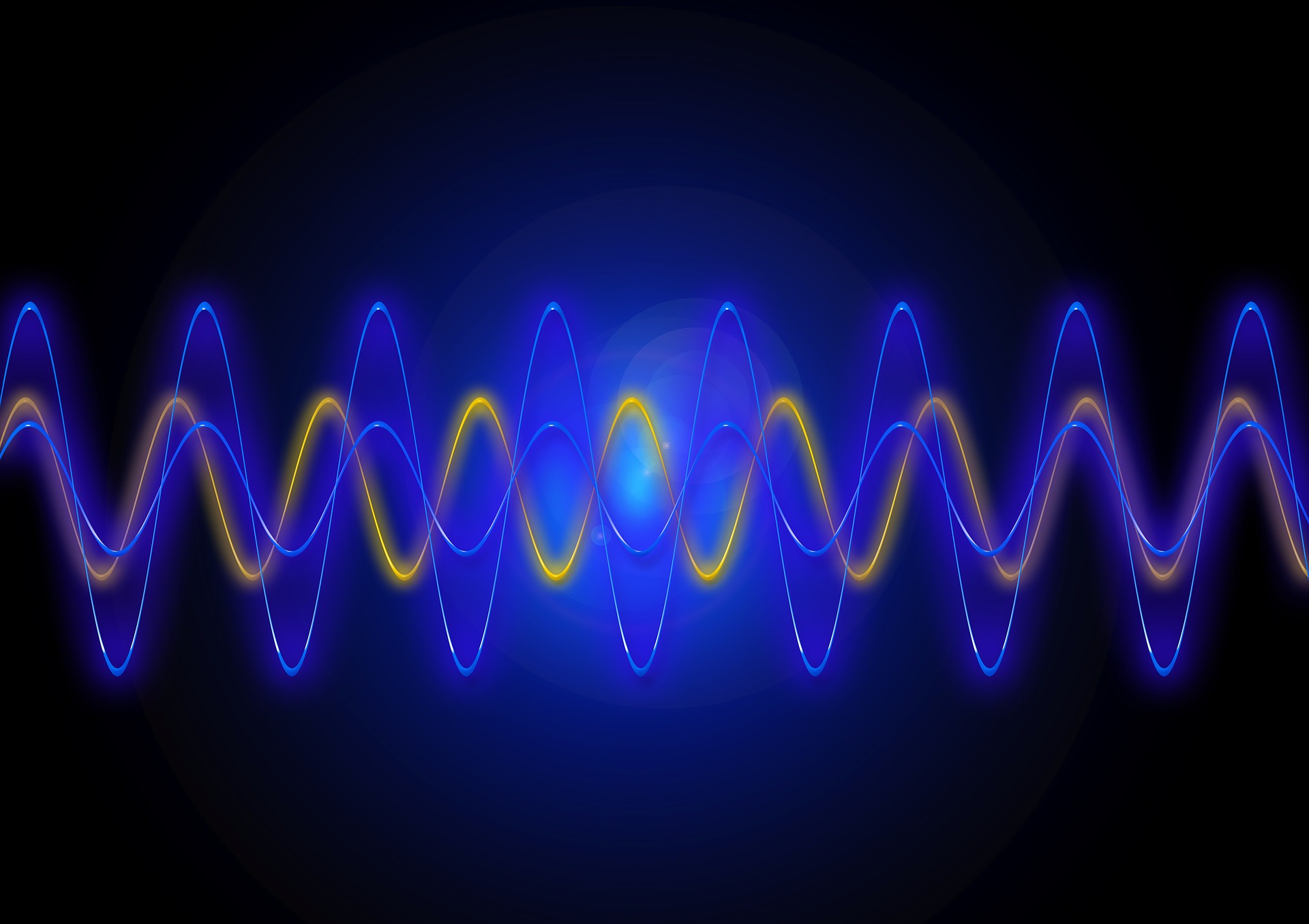
- Read more about ICASSP 2019 presentation slides
- Log in to post comments
We propose a complex-valued deep neural network (cDNN) for speech enhancement and source separation. While existing end-to-end systems use complex-valued gradients to pass the training error to a real-valued DNN used for gain mask estimation, we use the full potential of complex-valued LSTMs, MLPs and activation functions to estimate complex-valued beamforming weights directly from complex-valued microphone array data. By doing so, our cDNN is able to locate and track different moving sources by exploiting the phase information in the data.
- Categories:
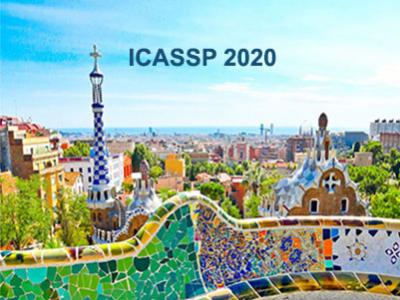
- Read more about Time-Frequency Feature Decomposition Based on Sound Duration for Acoustic Scene Classification
- Log in to post comments
- Categories: