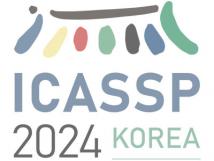
IEEE ICASSP 2024 - IEEE International Conference on Acoustics, Speech and Signal Processing (ICASSP) is the world’s largest and most comprehensive technical conference focused on signal processing and its applications. The IEEE ICASSP 2024 conference will feature world-class presentations by internationally renowned speakers, cutting-edge session topics and provide a fantastic opportunity to network with like-minded professionals from around the world. Visit the website.
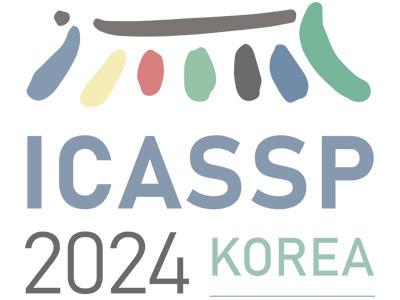
- Read more about Noisy-ArcMix: Additive Noisy Angular Margin Loss Combined With Mixup for Anomalous Sound Detection
- Log in to post comments
Unsupervised anomalous sound detection (ASD) aims to identify anomalous sounds by learning the features of normal operational sounds and sensing their deviations. Recent approaches have focused on the self-supervised task utilizing the classification of normal data, and advanced models have shown that securing representation space for anomalous data is important through representation learning yielding compact intra-class and well-separated intra-class distributions.
- Categories:
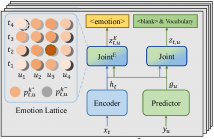
- Read more about Emotion Neural Transducer for Fine-Grained Speech Emotion Recognition
- Log in to post comments
The mainstream paradigm of speech emotion recognition (SER) is identifying the single emotion label of the entire utterance. This line of works neglect the emotion dynamics at fine temporal granularity and mostly fail to leverage linguistic information of speech signal explicitly. In this paper, we propose Emotion Neural Transducer for fine-grained speech emotion recognition with automatic speech recognition (ASR) joint training. We first extend typical neural transducer with emotion joint network to construct emotion lattice for fine-grained SER.
- Categories:
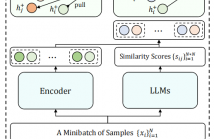
- Read more about Robust Self-Supervised Learning With Contrast Samples For Natural Language Understanding
- Log in to post comments
To improve the robustness of pre-trained language models (PLMs), previous studies have focused more on how to efficiently obtain adversarial samples with similar semantics, but less attention has been paid to the perturbed samples that change the gold label. Therefore, to fully perceive the effects of these different types of small perturbations on robustness, we propose a RObust Self-supervised leArning (ROSA) method, which incorporates different types of perturbed samples and the robustness improvements into a unified framework.
- Categories:
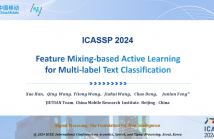
- Read more about Feature Mixing-based Active Learning for Multi-label Text Classification
- Log in to post comments
Active learning (AL) aims to reduce labeling costs by selecting the most valuable samples to annotate from a set of unlabeled data. However, recognizing these samples is particularly challenging in multi-label text classification tasks due to the high dimensionality but sparseness of label spaces. Existing AL techniques either fail to sufficiently capture label correlations, resulting in label imbalance in the selected samples, or suffer significant computing costs when analyzing the informative potential of unlabeled samples across all labels.
- Categories:
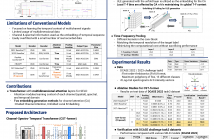
- Read more about CST-FORMER: TRANSFORMER WITH CHANNEL-SPECTRO-TEMPORAL ATTENTION FOR SOUND EVENT LOCALIZATION AND DETECTION
- Log in to post comments
Sound event localization and detection (SELD) is a task for the classification of sound events and the localization of direction of arrival (DoA) utilizing multichannel acoustic signals. Prior studies employ spectral and channel information as the embedding for temporal attention. However, this usage limits the deep neural network from extracting meaningful features from the spectral or spatial domains.
- Categories:
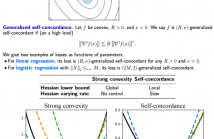
- Read more about The Rao, Wald, and Likelihood-Ratio Tests under Generalized Self-Concordance
- Log in to post comments
Three classical approaches to goodness-of-fit testing are Rao’s test, Wald’s test, and the likelihood-ratio test. The asymptotic equivalence of these three tests under the null hypothesis is a famous connection in statistical detection theory. We revisit these three likelihood-related tests from a non-asymptotic viewpoint under self-concordance assumptions. We recover the equivalence of the three tests and characterize the critical sample size beyond which the equivalence holds asymptotically. We also investigate their behavior under local alternatives.
- Categories:
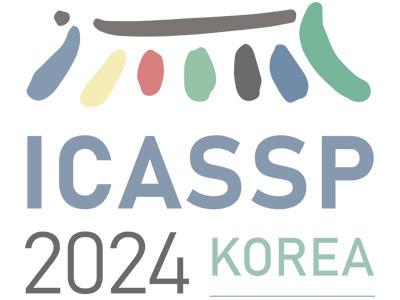
- Read more about SICRN: Advancing Speech Enhancement through State Space Model and Inplace Convolution Techniques
- 1 comment
- Log in to post comments
Speech enhancement aims to improve speech quality and intelligibility, especially in noisy environments where background noise degrades speech signals. Currently, deep learning methods achieve great success in speech enhancement, e.g. the representative convolutional recurrent neural network (CRN) and its variants. However, CRN typically employs consecutive downsampling and upsampling convolution for frequency modeling, which destroys the inherent structure of the signal over frequency. Additionally, convolutional layers lacks of temporal modelling abilities.
- Categories:
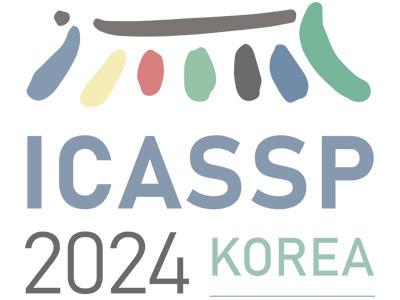
- Read more about Federated PAC-Bayesian Learning on Non-IID Data
- Log in to post comments
Existing research has either adapted the Probably Approximately Correct (PAC) Bayesian framework for federated learning (FL) or used information-theoretic PAC-Bayesian bounds while introducing their theorems, but few consider the non-IID challenges in FL. Our work presents the first non-vacuous federated PAC-Bayesian bound tailored for non-IID local data. This bound assumes unique prior knowledge for each client and variable aggregation weights. We also introduce an objective function and an innovative Gibbs-based algorithm for the optimization of the derived bound.
- Categories:
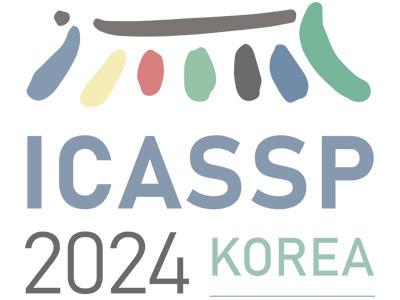
- Read more about Adaptive speech emotion representation learning based on dynamic graph
- 1 comment
- Log in to post comments
Graph representation learning has become a hot research topic due to its powerful nonlinear fitting capability in extracting representative node embeddings. However, for sequential data such as speech signals, most traditional methods merely focus on the static graph created within a sequence, and largely overlook the intrinsic evolving patterns of these data. This may reduce the efficiency of graph representation learning for sequential data.
- Categories:
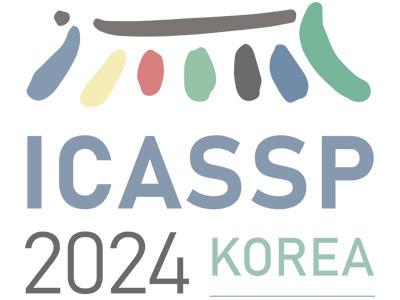
- Read more about Unsupervised Continual Learning of Image Representation via Rememory-based SimSiam
- Log in to post comments
Unsupervised continual learning (UCL) of image representation has garnered attention due to practical need. However, recent UCL methods focus on mitigating the catastrophic forgetting with a replay buffer (i.e., rehearsal-based strategy), which needs much extra storage. To overcome this drawback, we propose a novel rememory-based SimSiam (RM-SimSiam) method to reduce the dependency on replay buffer. The core idea of RM-SimSiam is to store and remember the old knowledge with a data-free historical module instead of replay buffer.
- Categories: