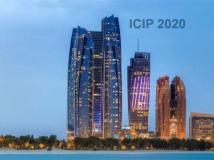
ICIP 2020 is a fully virtual conference. The International Conference on Image Processing (ICIP), sponsored by the IEEE Signal Processing Society, is the premier forum for the presentation of technological advances and research results in the fields of theoretical, experimental, and applied image and video processing. ICIP has been held annually since 1994, brings together leading engineers and scientists in image and video processing from around the world. Visit website.
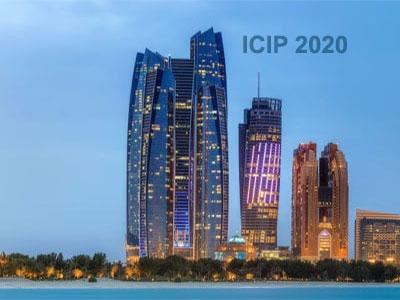
This paper presents a soft-label anonymous gastric X-ray image distillation method based on a gradient descent approach. The sharing of medical data is demanded to construct high-accuracy computer-aided diagnosis (CAD) systems. However, the large size of the medical dataset and privacy protection are remaining problems in medical data sharing, which hindered the research of CAD systems. The idea of our distillation method is to extract the valid information of the medical dataset and generate a tiny distilled dataset that has a different data distribution.
- Categories:
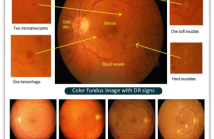
- Read more about SEA-NET: SQUEEZE-AND-EXCITATION ATTENTION NET FOR DIABETIC RETINOPATHY GRADING
- Log in to post comments
Diabetes is one of the most common disease in individuals. Diabetic retinopathy (DR) is a complication of diabetes, which could lead to blindness. Automatic DR grading based on retinal images provides a great diagnostic and prognostic value for treatment planning. However, the subtle differences among severity levels make it difficult to capture important features using conventional methods.
- Categories:
- Read more about TYPE I ATTACK FOR GENERATIVE MODELS
- Log in to post comments
Generative models are popular tools with a wide range of applications. Nevertheless, it is as vulnerable to adversarial samples as classifiers. The existing attack methods mainly focus on generating adversarial examples by adding imperceptible perturbations to input, which leads to wrong result. However, we focus on another aspect of attack, i.e., cheating models by significant changes. The former induces Type II error and the latter causes Type I error. In this paper, we propose Type I attack to generative models such as VAE and GAN.
- Categories:
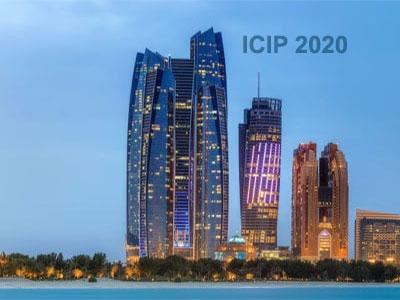
- Read more about VISUAL RELATIONSHIP CLASSIFICATION WITH NEGATIVE-SAMPLE MINING
- Log in to post comments
This paper introduces the application of a visual relationship classifier as a standalone system that is meant to be used with external detectors. Through these lens, we propose a training scheme that uses unannotated pairs of objects as negative samples in order to improve precision. The proposed network architecture incorporates common techniques presented in related state-of-the-art solutions with a novel positional encoding scheme.
estevao.pdf

- Categories:
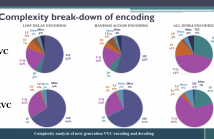
- Read more about Complexity Analysis Of Next-Generation VVC Encoding and Decoding
- Log in to post comments
While the next generation video compression standard, Versatile Video Coding (VVC), provides a superior compression efficiency, its computational complexity dramatically increases. This paper thoroughly analyzes this complexity for both encoder and decoder of VVC Test Model 6, by quantifying the complexity break-down for each coding tool and measuring the complexity and memory requirements for VVC encoding/decoding.
- Categories:
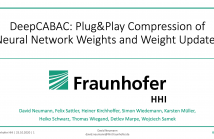
- Read more about DeepCABAC - Plug&Play Compression of Neural Network Weights and Weight Updates
- Log in to post comments
An increasing number of distributed machine learning applications require efficient communication of neural network parameterizations. DeepCABAC, an algorithm in the current working draft of the emerging MPEG-7 part 17 standard for compression of neural networks for multimedia content description and analysis, has demonstrated high compression gains for a variety of neural network models. In this paper we propose a method for employing DeepCABAC in a Federated Learning scenario for the exchange of intermediate differential parameterizations.
- Categories:
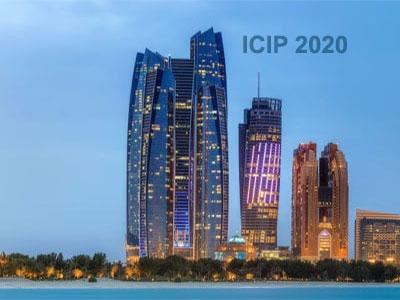
- Categories:
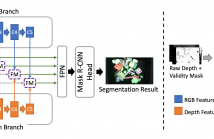
- Read more about Segmenting Unseen Industrial Components In A Heavy Clutter Using RGB-D Fusion And Synthetic Data
- Log in to post comments
Segmentation of unseen industrial parts is essential for autonomous industrial systems. However, industrial components are texture-less, reflective, and often found in cluttered and unstructured environments with heavy occlusion, which makes it more challenging to deal with unseen objects. To tackle this problem, we present a synthetic data generation pipeline that randomizes textures via domain randomization to focus on the shape information.
- Categories:
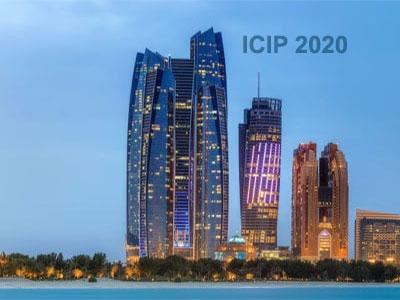
- Read more about DOES SUPER-RESOLUTION IMPROVE OCR PERFORMANCE IN THE REAL WORLD? A CASE STUDY ON IMAGES OF RECEIPTS
- Log in to post comments
Recently, many deep learning methods have been used to handle single image super-resolution (SISR) tasks and often achieve state-of-the-art performance. From a visual point of view, the results look convincing. Yet, does it mean that those techniques are reliable and robust enough to be implemented in real business cases to enhance the performance of other computer vision tasks?
- Categories:
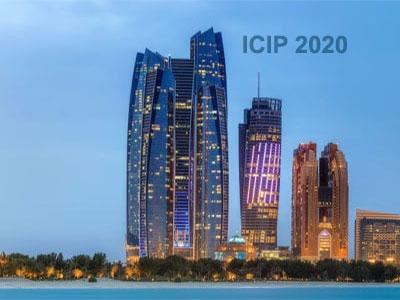
- Read more about Lossless Video Coding Based on Probability Model Optimization Utilizing Example Search and Adaptive Prediction
- Log in to post comments
- Categories: