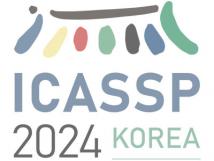
IEEE ICASSP 2024 - IEEE International Conference on Acoustics, Speech and Signal Processing (ICASSP) is the world’s largest and most comprehensive technical conference focused on signal processing and its applications. The IEEE ICASSP 2024 conference will feature world-class presentations by internationally renowned speakers, cutting-edge session topics and provide a fantastic opportunity to network with like-minded professionals from around the world. Visit the website.
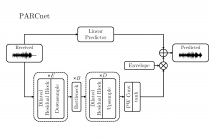
- Read more about Hybrid Packet Loss Concealment for Real-Time Networked Music Applications
- Log in to post comments
Real-time audio communications over IP have become essential to our daily lives. Packet-switched networks, however, are inherently prone to jitter and data losses, thus creating a strong need for effective packet loss concealment (PLC) techniques.
- Categories:
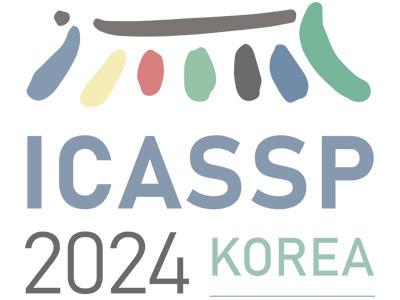
- Read more about Photovoltaic power forecasting using sky images and sun motion
- Log in to post comments
Solar energy adoption is moving at a rapid pace. The variability in solar energy production causes grid stability issues and hinders mass adoption. To solve these issues, more accurate photovoltaic power forecasting systems are needed. In intra-hour forecasting, the most challenging issue is high output fluctuations due to cloud motion, which can occlude the sun.
- Categories:
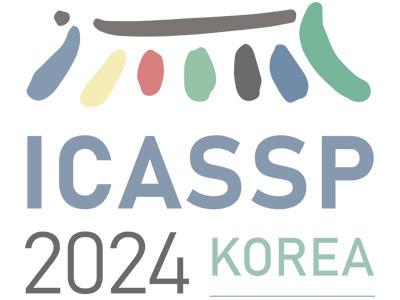
- Read more about Gridless Parameter Estimation in Partly Calibrated Rectangular Arrays
- Log in to post comments
Poster for the paper SAM-P6.7: GRIDLESS PARAMETER ESTIMATION IN PARTLY CALIBRATED RECTANGULAR ARRAYS in IEEE ICASSP 2024
- Categories:
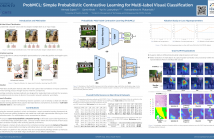
- Read more about PROBMCL: SIMPLE PROBABILISTIC CONTRASTIVE LEARNING FOR MULTI-LABEL VISUAL CLASSIFICATION
- Log in to post comments
Multi-label image classification presents a challenging task in many domains, including computer vision and medical imaging. Recent advancements have introduced graph-based and transformer-based methods to improve performance and capture label dependencies. However, these methods often include complex modules that entail heavy computation and lack interpretability. In this paper, we propose Probabilistic Multi-label Contrastive Learning (ProbMCL), a novel framework to address these challenges in multi-label image classification tasks.
- Categories:
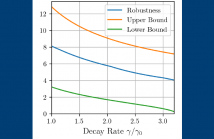
Nuclear magnetic resonance (NMR) spectroscopy is routinely used to study the properties of matter. Therefore, different materials can be classified according to their NMR spectra. However, the NMR spectra cannot be observed directly, and only the NMR signal, which is a sum of complex exponentials, is directly observable in practice. A popular approach to recover the spectrum is to perform harmonic retrieval, i.e., to reconstruct exactly the spectrum from the NMR signal. However, even when this approach fails, the spectrum might still be classified accurately.
- Categories:
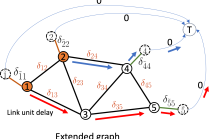
- Read more about Congestion-aware Distributed Task Offloading in Wireless Multi-hop Networks using Graph Neural Networks
- Log in to post comments
Computational offloading has become an enabling component for edge intelligence in mobile and smart devices. Existing offloading schemes mainly focus on mobile devices and servers, while ignoring the potential network congestion caused by tasks from multiple mobile devices, especially in wireless multi-hop networks. To fill this gap, we propose a low-overhead, congestion-aware distributed task offloading scheme by augmenting a distributed greedy framework with graph-based machine learning.
- Categories:
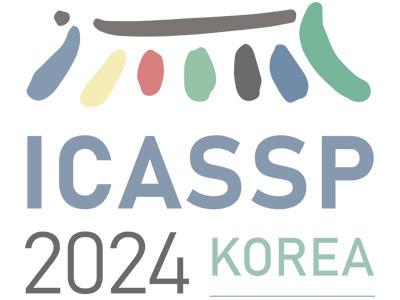
- Read more about Self-Supervised Face Image Restoration with a One-Shot Reference
- Log in to post comments
For image restoration, methods leveraging priors from generative models have been proposed and demonstrated a promising capacity to robustly restore photorealistic and high-quality results. However, these methods are susceptible to semantic ambiguity, particularly with images that have obviously correct semantics, such as facial images. In this paper, we propose a semantic-aware latent space exploration method for image restoration (SAIR).
- Categories:
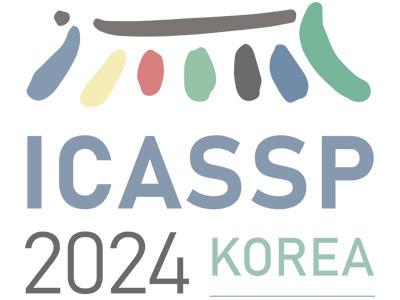
We introduce the Generative Barankin Bound (GBB), a learned Barankin Bound, for evaluating the achievable performance in estimating the direction of arrival (DOA) of a source in non-asymptotic conditions, when the statistics of the measurement are unknown. We first learn the measurement distribution using a conditional normalizing flow (CNF) and then use it to derive the GBB.
- Categories:
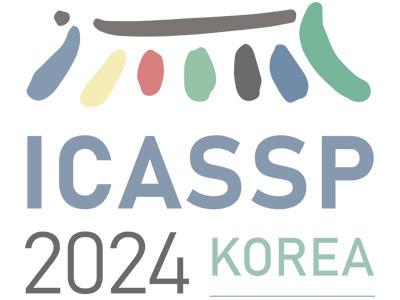
- Read more about Stage-Regularized Neural Stein Critics for Testing Goodness-of-Fit of Generative Models
- Log in to post comments
Learning to differentiate model distributions from observed data is a fundamental problem in statistics and machine learning, and high-dimensional data remains a challenging setting for such problems. Metrics that quantify the disparity in probability distributions, such as the Stein discrepancy, play an important role in high-dimensional statistical testing. This paper presents a method based on neural network Stein critics to distinguish between data sampled from an unknown probability distribution and a nominal model distribution with a novel staging of the weight of regularization.
- Categories:
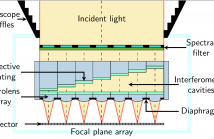
- Read more about Spectro-spatial hyperspectral image reconstruction from interferometric acquisitions
- Log in to post comments
In the last decade, novel hyperspectral cameras have been developed with particularly desirable characteristics of compactness and short acquisition time, retaining their potential to obtain spectral/spatial resolution competitive with respect to traditional cameras. However, a computational effort is required to recover an interpretable data cube.
poster.pdf

- Categories: