- Transducers
- Spatial and Multichannel Audio
- Source Separation and Signal Enhancement
- Room Acoustics and Acoustic System Modeling
- Network Audio
- Audio for Multimedia
- Audio Processing Systems
- Audio Coding
- Audio Analysis and Synthesis
- Active Noise Control
- Auditory Modeling and Hearing Aids
- Bioacoustics and Medical Acoustics
- Music Signal Processing
- Loudspeaker and Microphone Array Signal Processing
- Echo Cancellation
- Content-Based Audio Processing
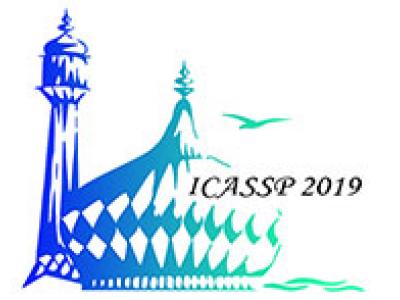
- Read more about Recurrent Neural Networks with Stochastic Layers for Acoustic Novelty Detection
- Log in to post comments
In this paper, we adapt Recurrent Neural Networks with Stochastic Layers, which are the state-of-the-art for generating text, music and speech, to the problem of acoustic novelty detection. By integrating uncertainty into the hidden states, this type of network is able to learn the distribution of complex sequences. Because the learned distribution can be calculated explicitly in terms of probability, we can evaluate how likely an observation is then detect low-probability events as novel.
- Categories:
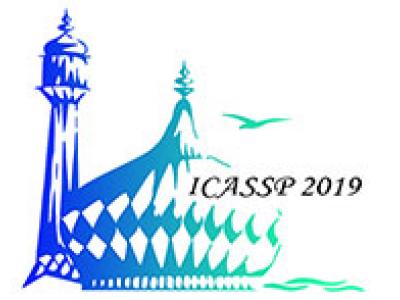
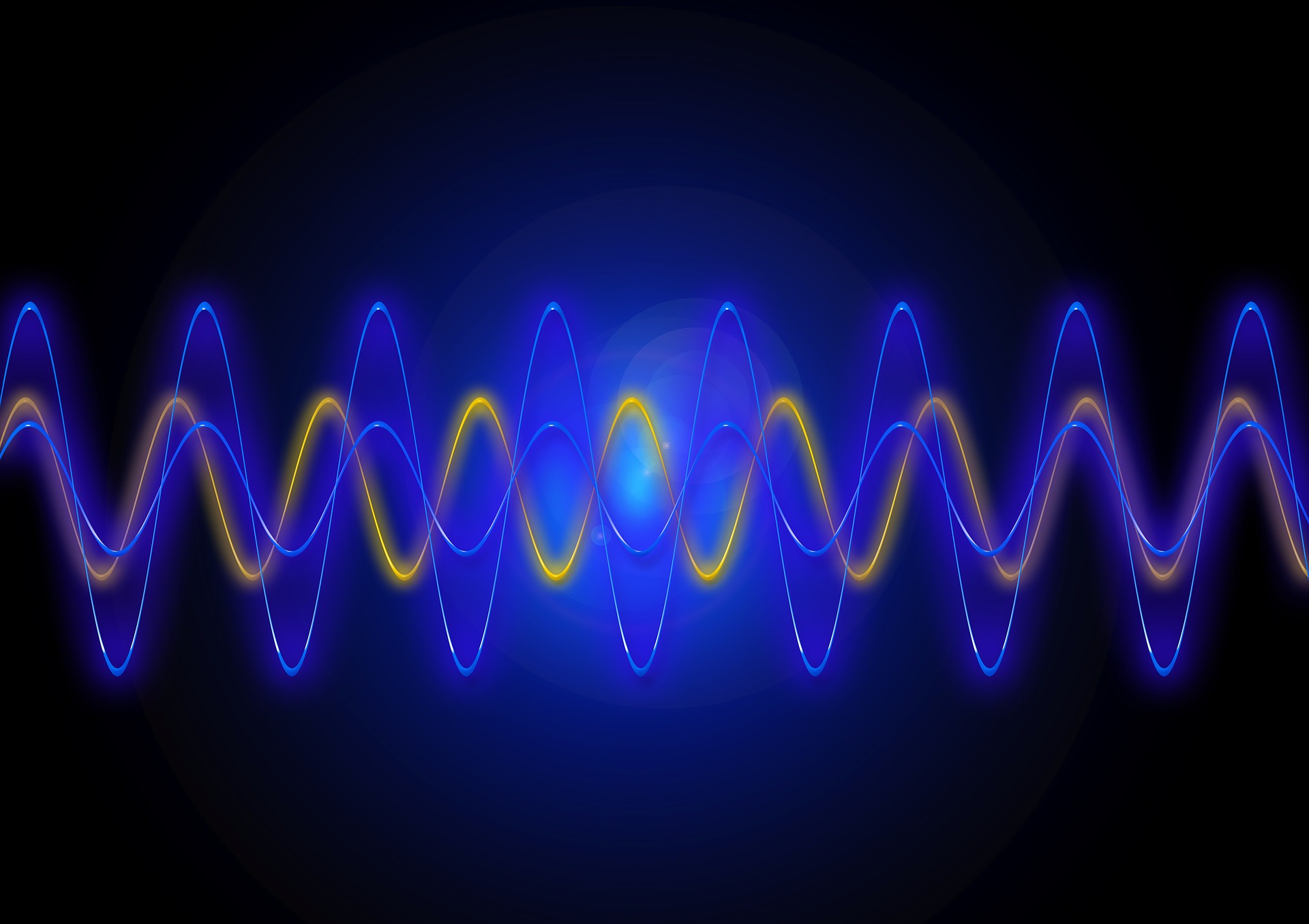
- Read more about POLYPHONIC SOUND EVENT DETECTION USING CONVOLUTIONAL BIDIRECTIONAL LSTM AND SYNTHETIC DATA-BASED TRANSFER LEARNING
- Log in to post comments
- Categories:
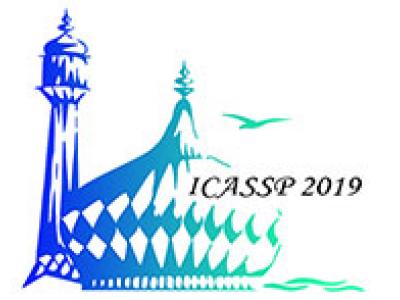
- Read more about SPEAKER AGNOSTIC FOREGROUND SPEECH DETECTION FROM AUDIO RECORDINGS IN WORKPLACE SETTINGS FROM WEARABLE RECORDERS
- Log in to post comments
Audio-signal acquisition as part of wearable sensing adds an important dimension for applications such as understanding human behaviors. As part of a large study on work place behaviors, we collected audio data from individual hospital staff using custom wearable recorders. The audio features collected were limited to preserve privacy of the interactions in the hospital. A first step towards audio processing is to identify the foreground speech of the person wearing the audio badge.
- Categories:
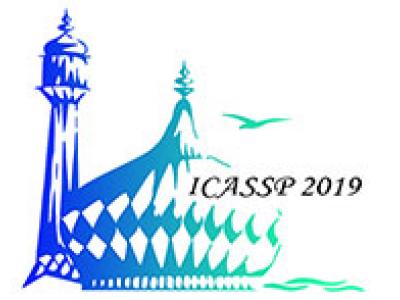
- Read more about EMBEDDING PHYSICAL AUGMENTATION AND WAVELET SCATTERING TRANSFORM TO GENERATIVE ADVERSARIAL NETWORKS FOR AUDIO CLASSIFICATION WITH LIMITED TRAINING RESOURCES
- Log in to post comments
This paper addresses audio classification with limited training resources. We first investigate different types of data augmentation including physical modeling, wavelet scattering transform and Generative Adversarial Networks (GAN). We than propose a novel GAN which allows embedding of physical augmentation and wavelet scattering transform in processing. The experimental results on Google Speech Command show significant improvements of the proposed method when training with limited resources.
- Categories:
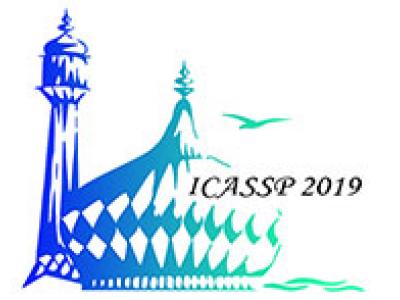
- Read more about MULTI-BAND PIT AND MODEL INTEGRATION FOR IMPROVED MULTI-CHANNEL SPEECH SEPARATION
- Log in to post comments
- Categories:
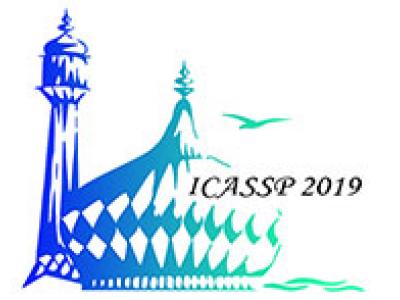
- Read more about Point Cloud Segmentation using Hierarchical Tree for Architectural Models.
- Log in to post comments
Over the past few years, gathering massive volume of 3D data has become straightforward due to the proliferation of laser scanners and acquisition devices. Segmentation of such large data into meaningful segments, however, remains a challenge. Raw scans usually have missing data and varying density. In this work, we present a simple yet effective method to semantically decompose and reconstruct 3D models from point clouds. Using a hierarchical tree approach, we segment and reconstruct planar as well as non-planar scenes in an outdoor environment.
- Categories:
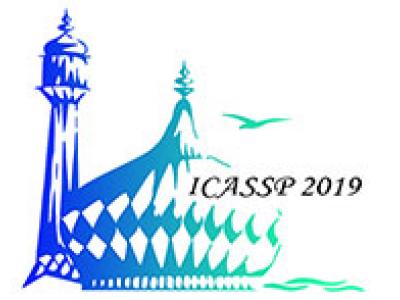
In this paper, we present our novel approach to the 6th Dialogue State Tracking Challenge (DSTC6) track for end-to-end goal-oriented dialogue, in which the goal is to select the best system response from among a list of candidates in a restaurant booking conversation. Our model uses a convolutional neural network (CNN) for semantic tagging of each utterance in the dialogue history to update the dialogue state, and another CNN for predicting the best system action template.
icassp19.pdf

- Categories:
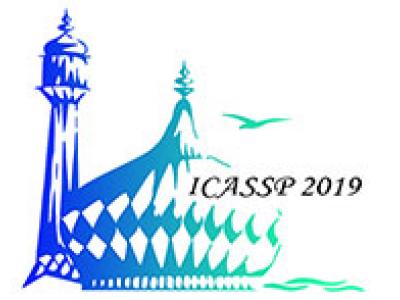
- Read more about INCREMENTAL TRANSFER LEARNING IN TWO-PASS INFORMATION BOTTLENECK BASED SPEAKER DIARIZATION SYSTEM FOR MEETINGS
- Log in to post comments
The two-pass information bottleneck (TPIB) based speaker diarization system operates independently on different conversational recordings. TPIB system does not consider previously learned speaker discriminative information while diarizing new conversations. Hence, the real time factor (RTF) of TPIB system is high owing to the training time required for the artificial neural network (ANN).
- Categories:
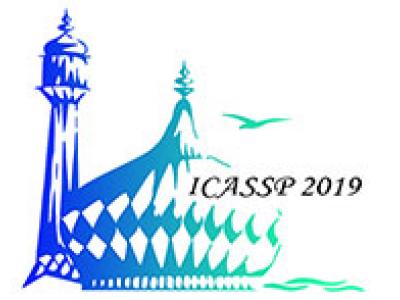
- Read more about Robust Room Equalization Using Sparse Sound-Field Reconstruction
- Log in to post comments
- Categories: