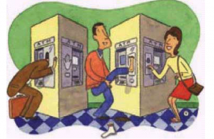
- Read more about Discriminant Correlation Analysis for Feature Level Fusion with Application to Multimodal Biometrics
- Log in to post comments
In this paper, we present Discriminant Correlation Analysis (DCA), a feature level fusion technique that incorporates the class associations in correlation analysis of the feature sets. DCA performs an effective feature fusion by maximizing the pair-wise correlations across the two feature sets, and at the same time, eliminating the between-class correlations and restricting the correlations to be within classes.
- Categories:
- Read more about A NOVEL GENERALIZED ASSIGNMENT FRAMEWORK FOR THE CLASSIFICATION OF HYPERSPECTRAL IMAGE
- Log in to post comments
Recently, sparse representation based classification has been widely used in pattern recognition. Most of existing methods exploit the recovered representation coefficients to reconstruct the inputs, and the classwise reconstruction errors are used to identify the class of the sample based on the subspace assumption. Different from the reconstruction pipeline, an assignment framework is built on the representation coefficients in this paper.
- Categories:
- Read more about EPILEPTIFORM SPIKE DETECTION VIA CONVOLUTIONAL NEURAL NETWORKS
- Log in to post comments
- Categories:
- Read more about Characterization and Classification of Sonar Targets Using Ellipsoid Features
- Log in to post comments
We present a geometry-inspired characterization of
target response for active sonar that exploits similarity between
intra-class features to distinguish between different targets
against environmental objects such as a rock. Key innovation is to
represent feature manifolds as a set of ellipsoids, each of which
geometrically encompasses a unique physical characteristic of
the target’s response. We have demonstrated over experimental
field data that for a given target class, these feature ellipsoids
- Categories:
- Read more about Kernel-based low-rank feature extraction on a budget for Big data streams
- Log in to post comments
- Categories:
- Read more about 3D Object Modeling and Recognition via Online Hierarchical Pitman-Yor Process Mixture Learning
- Log in to post comments
- Categories:
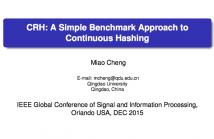
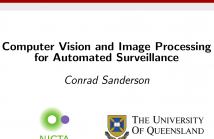
Presentation slides covering:
- robust foreground detection / background subtraction via patch-based analysis
- person re-identification based on representations on Riemannian manifolds
- robust object tracking via Grassmann manifolds
- adapting the lessons from big data to computer vision
- future paradigm shifts: computer vision based on networks of neurosynaptic cores
- Categories: