- Bayesian learning; Bayesian signal processing (MLR-BAYL)
- Bounds on performance (MLR-PERF)
- Applications in Systems Biology (MLR-SYSB)
- Applications in Music and Audio Processing (MLR-MUSI)
- Applications in Data Fusion (MLR-FUSI)
- Cognitive information processing (MLR-COGP)
- Distributed and Cooperative Learning (MLR-DIST)
- Learning theory and algorithms (MLR-LEAR)
- Neural network learning (MLR-NNLR)
- Information-theoretic learning (MLR-INFO)
- Independent component analysis (MLR-ICAN)
- Graphical and kernel methods (MLR-GRKN)
- Other applications of machine learning (MLR-APPL)
- Pattern recognition and classification (MLR-PATT)
- Source separation (MLR-SSEP)
- Sequential learning; sequential decision methods (MLR-SLER)
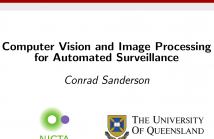
Presentation slides covering:
- robust foreground detection / background subtraction via patch-based analysis
- person re-identification based on representations on Riemannian manifolds
- robust object tracking via Grassmann manifolds
- adapting the lessons from big data to computer vision
- future paradigm shifts: computer vision based on networks of neurosynaptic cores
- Categories:
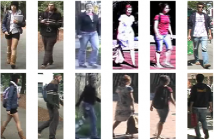
Slides from the Tutorial on Riemannian Geometry in Computer Vision, presented at the Asian Conference on Computer Vision (ACCV), Singapore, 2014.
The slides show (1) how objects can be interpreted as points on Riemannian and Grassmann manifolds, and (2) various distance measures on manifolds. Demonstrates usefulness of manifold techniques in applications such as object tracking and person re-identification.
- Categories: