- Bayesian learning; Bayesian signal processing (MLR-BAYL)
- Bounds on performance (MLR-PERF)
- Applications in Systems Biology (MLR-SYSB)
- Applications in Music and Audio Processing (MLR-MUSI)
- Applications in Data Fusion (MLR-FUSI)
- Cognitive information processing (MLR-COGP)
- Distributed and Cooperative Learning (MLR-DIST)
- Learning theory and algorithms (MLR-LEAR)
- Neural network learning (MLR-NNLR)
- Information-theoretic learning (MLR-INFO)
- Independent component analysis (MLR-ICAN)
- Graphical and kernel methods (MLR-GRKN)
- Other applications of machine learning (MLR-APPL)
- Pattern recognition and classification (MLR-PATT)
- Source separation (MLR-SSEP)
- Sequential learning; sequential decision methods (MLR-SLER)
- Read more about Greedy Deep Transform Learning
- Log in to post comments
We introduce deep transform learning – a new
tool for deep learning. Deeper representation is learnt by
stacking one transform after another. The learning proceeds in
a greedy way. The first layer learns the transform and features
from the input training samples. Subsequent layers use the
features (after activation) from the previous layers as training
input. Experiments have been carried out with other deep
representation learning tools – deep dictionary learning,
stacked denoising autoencoder, deep belief network and PCANet
- Categories:
- Read more about AN IMAGE RECONSTRUCTION FRAMEWORK BASED ON DEEP NEURAL NETWORK FOR ELECTRICAL IMPEDANCE TOMOGRAPHY
- Log in to post comments
- Categories:
- Categories:
Although promising results have been achieved in the areas of object detection and classification, few works have provided an end-to-end solution to the perception problems in the autonomous driving field. In this paper, we make two contributions. Firstly, we fully enhanced our previously released TT100K benchmark and provide 16,817 elaborately labeled Tencent Street View panoramas.
- Categories:
- Read more about Phase Retrieval via Coordinate Descent
- Log in to post comments
Phase retrieval refers to recovery of a signal-of-interest given only the intensity measurement samples and has wide applicability including important areas of astronomy, computational biology, crystallography, digital communications, electron microscopy, neutron radiography and optical imaging. The classical problem formulation is to restore the time-domain signal from its power spectrum observations, although the Fourier transform can be generalized to any linear mappings.
- Categories:
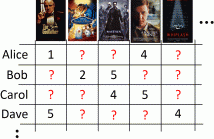
Matrix completion aims to find the missing entries from incomplete observations using the low-rank property. Conventional convex optimization based techniques minimize the nuclear norm subject to a constraint on the Frobenius norm of the residual. However, they are not robust to outliers and have a high computational complexity. Different from the existing schemes based on solving a minimization problem, we formulate matrix completion as a feasibility problem.
- Categories:
- Read more about AFFECT RECOGNITION FROM LIP ARTICULATIONS
- Log in to post comments
- Categories:
- Read more about Disjunctive Normal Shape Boltzmann Machine
- Log in to post comments
Shape Boltzmann machine (a type of Deep Boltzmann machine) is a powerful tool for shape modelling; however, has some drawbacks in representation of local shape parts. Disjunctive Normal Shape Model (DNSM) is a strong shape model that can effectively represent local parts of objects. In this paper, we propose a new shape model based on Shape Boltzmann Machine and Disjunctive Normal Shape Model which we call Disjunctive Normal Shape Boltzmann Machine (DNSBM).
- Categories:
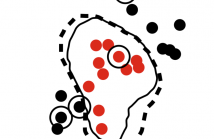
Despite the importance of distributed learning, few fully distributed support vector machines exist. In this paper, not only do we provide a fully distributed nonlinear SVM; we propose the first distributed constrained-form SVM. In the fully distributed context, a dataset is distributed among networked agents that cannot divulge their data, let alone centralize the data, and can only communicate with their neighbors in the network. Our strategy is based on two algorithms: the Douglas-Rachford algorithm and the projection-gradient method.
Poster.pdf

- Categories:
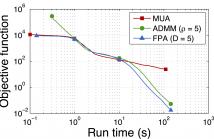
- Read more about PRIMAL-DUAL ALGORITHMS FOR NON-NEGATIVE MATRIX FACTORIZATION WITH THE KULLBACK-LEIBLER DIVERGENCE
- Log in to post comments
Non-negative matrix factorization (NMF) approximates a given matrix as a product of two non-negative matrix factors. Multiplicative algorithms deliver reliable results, but they show slow convergence for high-dimensional data and may be stuck away from local minima. Gradient descent methods have better behavior, but only apply to smooth losses. For non-smooth losses such as the Kullback-Leibler (KL) loss, surprisingly, these methods are lacking.
- Categories: