- Bayesian learning; Bayesian signal processing (MLR-BAYL)
- Bounds on performance (MLR-PERF)
- Applications in Systems Biology (MLR-SYSB)
- Applications in Music and Audio Processing (MLR-MUSI)
- Applications in Data Fusion (MLR-FUSI)
- Cognitive information processing (MLR-COGP)
- Distributed and Cooperative Learning (MLR-DIST)
- Learning theory and algorithms (MLR-LEAR)
- Neural network learning (MLR-NNLR)
- Information-theoretic learning (MLR-INFO)
- Independent component analysis (MLR-ICAN)
- Graphical and kernel methods (MLR-GRKN)
- Other applications of machine learning (MLR-APPL)
- Pattern recognition and classification (MLR-PATT)
- Source separation (MLR-SSEP)
- Sequential learning; sequential decision methods (MLR-SLER)
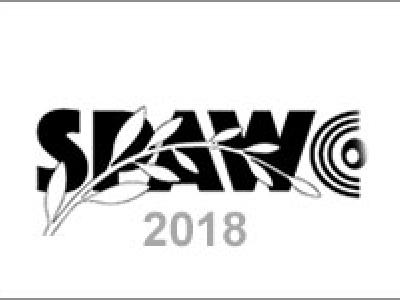
- Read more about Communication efficient coreset sampling for distributed learning
- Log in to post comments
In this paper, distributed learning is studied using the approach of coreset. In the context of classification, an algorithm of coreset construction is proposed to reduce the redundancy of data and thus the communication requirement, similarly to source coding in traditional data communications. It is shown that the coreset based boosting has a high convergence rate and small sample complexity. Moreover, it is robust to adversary distribution, thus leading to potential applications in distributed learning systems.
poster_v.pdf

- Categories:
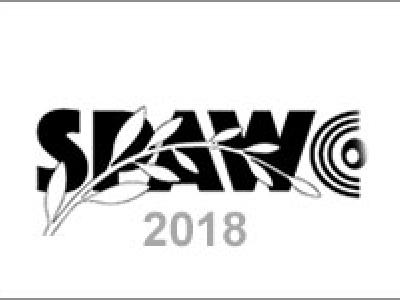
- Read more about Improved LDA Classifier based on Spiked Models
- Log in to post comments
poster.pdf

- Categories:
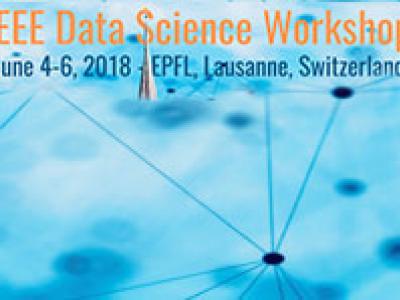
- Read more about Alternating autoencoders for matrix completion
- Log in to post comments
We consider autoencoders (AEs) for matrix completion (MC) with application to collaborative filtering (CF) for recommedation systems. It is observed that for a given sparse user-item rating matrix, denoted asM, an AE performs matrix factorization so that the recovered matrix is represented as a product of user and item feature matrices.
Poster_Lee.pdf

- Categories:
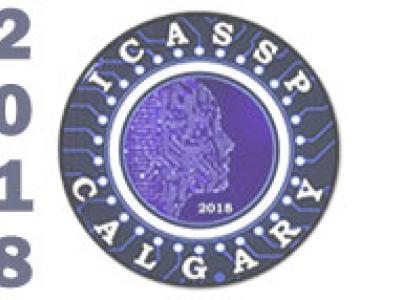
Clustering is widely used for exploratory data analysis in a variety of applications. Traditionally clustering is studied as an unsupervised task where no human inputs are provided. A recent trend in clustering is to leverage user provided side information to better infer the clustering structure in data. In this paper, we propose a probabilistic graphical model that allows user to provide as input the desired cluster sizes, namely the cardinality constraints. Our model also incorporates a flexible mechanism to inject control of the crispness of the clusters.
- Categories:
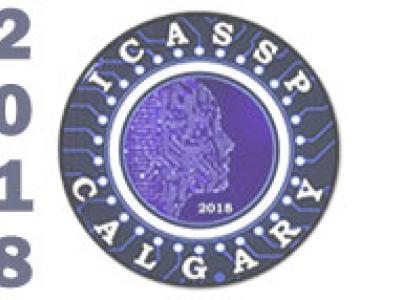
- Read more about A Constant Step Stochastic Douglas Rachford Algorithm with Application to Non Separable Regularizations
- Log in to post comments
- Categories:
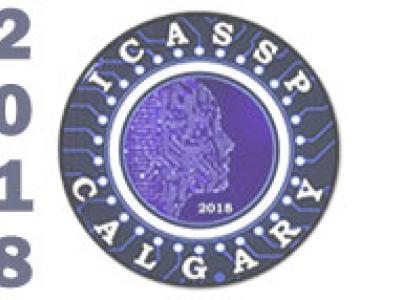
- Categories:
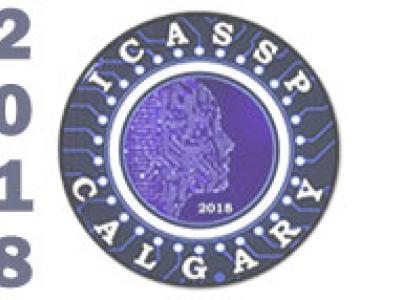
- Read more about Hi, BCD! Hybrid Inexact Block Coordinate Descent for Hyperspectral Super-Resolution
- Log in to post comments
Hyperspectral super-resolution (HSR) is a problem of recovering a high-spectral-spatial-resolution image from a multispectral measurement and a hyperspectral measurement, which have low spectral and spatial resolutions, respectively. We consider a low-rank structured matrix factorization formulation for HSR, which is a non-convex large-scale optimization problem. Our contributions contain both computational and theoretical aspects.
- Categories:
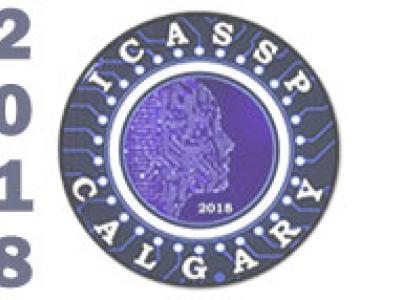
- Read more about Language and Noise Transfer in Speech Enhancement Generative Adversarial Network
- Log in to post comments
Speech enhancement deep learning systems usually require large amounts of training data to operate in broad conditions or real applications. This makes the adaptability of those systems into new, low resource environments an important topic. In this work, we present the results of adapting a speech enhancement generative adversarial network by fine-tuning the generator with small amounts of data. We investigate the minimum requirements to obtain a stable behavior in terms of several objective metrics in two very different languages: Catalan and Korean.
- Categories:
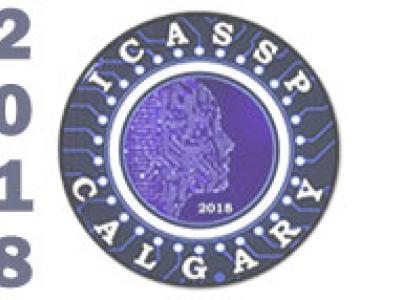
- Read more about Language and Noise Transfer in Speech Enhancement Generative Adversarial Network
- Log in to post comments
Speech enhancement deep learning systems usually require large amounts of training data to operate in broad conditions or real applications. This makes the adaptability of those systems into new, low resource environments an important topic. In this work, we present the results of adapting a speech enhancement generative adversarial network by fine-tuning the generator with small amounts of data. We investigate the minimum requirements to obtain a stable behavior in terms of several objective metrics in two very different languages: Catalan and Korean.
- Categories:
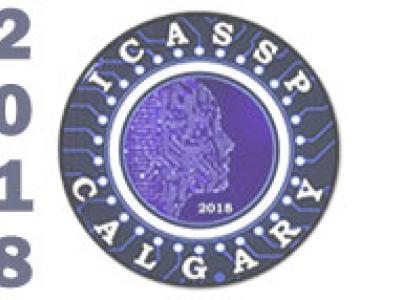
- Read more about Mobile Bayesian Spectrum Learning for Heterogeneous Networks
- Log in to post comments
Spectrum sensing in heterogeneous networks is very challenging as it usually requires a large number of static secondary users (SUs) to obtain the global spectrum states. In this paper, we tackle the spectrum sensing in heterogeneous networks from a new perspective. We exploit the mobility of multiple SUs to simultaneously collect spatial-temporal spectrum sensing data. Then, we propose a novel non-parametric Bayesian learning model, referred to as beta process hidden Markov model to capture the spatio-temporal correlation in the collected spectrum data.
- Categories: