- Bayesian learning; Bayesian signal processing (MLR-BAYL)
- Bounds on performance (MLR-PERF)
- Applications in Systems Biology (MLR-SYSB)
- Applications in Music and Audio Processing (MLR-MUSI)
- Applications in Data Fusion (MLR-FUSI)
- Cognitive information processing (MLR-COGP)
- Distributed and Cooperative Learning (MLR-DIST)
- Learning theory and algorithms (MLR-LEAR)
- Neural network learning (MLR-NNLR)
- Information-theoretic learning (MLR-INFO)
- Independent component analysis (MLR-ICAN)
- Graphical and kernel methods (MLR-GRKN)
- Other applications of machine learning (MLR-APPL)
- Pattern recognition and classification (MLR-PATT)
- Source separation (MLR-SSEP)
- Sequential learning; sequential decision methods (MLR-SLER)
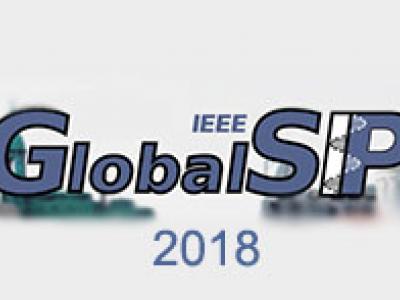
- Read more about Set-Theoretic Learning for Detection in Cell-Less C-RAN Systems
- Log in to post comments
- Categories:
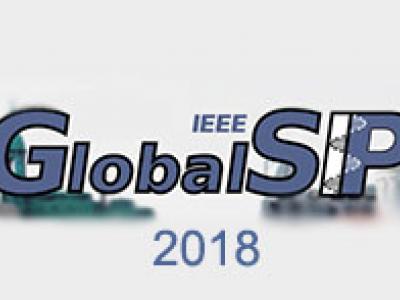
- Read more about On The Utility of Conditional Generation Based Mutual Information For Characterizing Adversarial Subspaces
- Log in to post comments
- Categories:
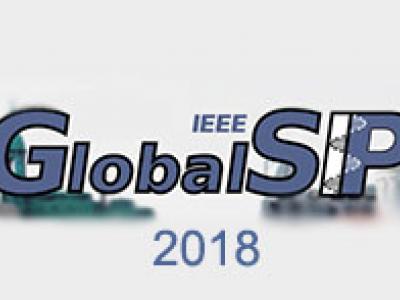
- Read more about GAN-NL: UNSUPERVISED REPRESENTATION LEARNING FOR REMOTE SENSING IMAGE CLASSIFICATION
- Log in to post comments
- Categories:
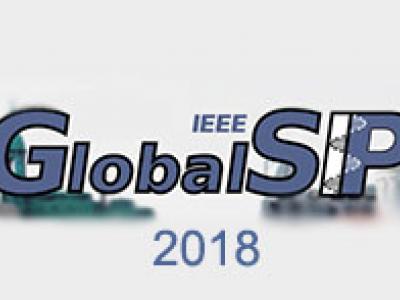
- Read more about ON THE BEHAVIOR OF THE EXPECTATION-MAXIMIZATION ALGORITHM FOR MIXTURE MODELS
- Log in to post comments
Globalsip.pdf

- Categories:
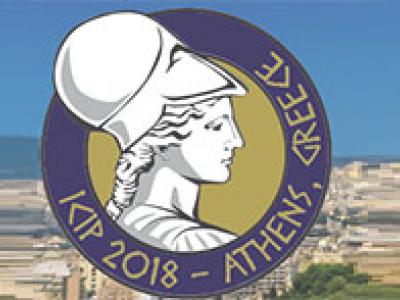
- Read more about Deep-learning-based pipe leak detection using image-based leak features
- Log in to post comments
- Categories:
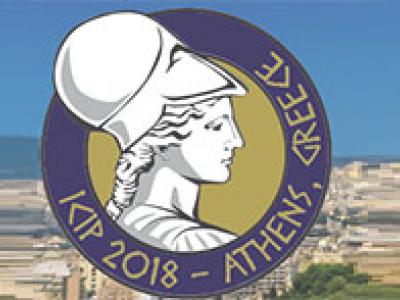
- Read more about Sequential Knowledge Transfer in Teacher-Student Framework using Densely Distilled Flow-Base Information
- Log in to post comments
- Categories:
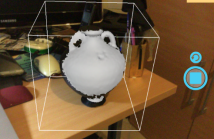
- Read more about AN INTERACTIVE CONTENT-BASED 3D SHAPE RETRIEVAL SYSTEM FOR ON-SITE CULTURAL HERITAGE ANALYSIS
- Log in to post comments
In this paper, we analyse the process of designing a Content-
Based 3D shape Retrieval (CB3DR) adapted for non-experts.
Our CB3DR solution aims at scanning an object on the fly
with a low-cost 3D sensor and retrieve similar shapes from
a database using the 3D point cloud acquired. Our system
should meet the requirements of archaeologists who would
like to be able to acquire artefacts without prior expertise in
scanning, then query easily from the field knowledge bases
for Cultural Heritage, and thus retrieve artefacts (i.e. objects
Icip2018.pptx

- Categories:
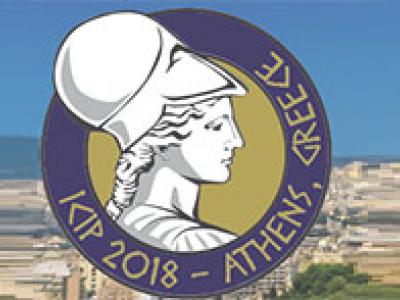
- Read more about BOTTOM-UP ATTENTION GUIDANCE FOR RECURRENT IMAGE RECOGNITION
- Log in to post comments
- Categories:
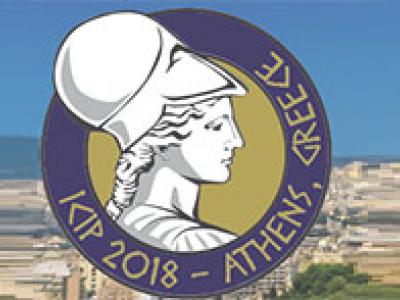
- Read more about GRADIENT BASED EVOLUTION TO OPTIMIZE THE STRUCTURE OF CONVOLUTIONAL NEURAL NETWORKS
- Log in to post comments
- Categories: