- Bayesian learning; Bayesian signal processing (MLR-BAYL)
- Bounds on performance (MLR-PERF)
- Applications in Systems Biology (MLR-SYSB)
- Applications in Music and Audio Processing (MLR-MUSI)
- Applications in Data Fusion (MLR-FUSI)
- Cognitive information processing (MLR-COGP)
- Distributed and Cooperative Learning (MLR-DIST)
- Learning theory and algorithms (MLR-LEAR)
- Neural network learning (MLR-NNLR)
- Information-theoretic learning (MLR-INFO)
- Independent component analysis (MLR-ICAN)
- Graphical and kernel methods (MLR-GRKN)
- Other applications of machine learning (MLR-APPL)
- Pattern recognition and classification (MLR-PATT)
- Source separation (MLR-SSEP)
- Sequential learning; sequential decision methods (MLR-SLER)
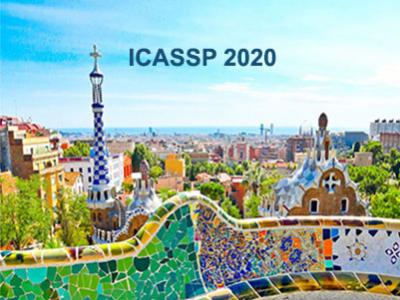
- Read more about AN ATTENTION ENHANCED MULTI-TASK MODEL FOR OBJECTIVE SPEECH ASSESSMENT IN REAL-WORLD ENVIRONMENTS
- Log in to post comments
slide_1603.pdf

- Categories:
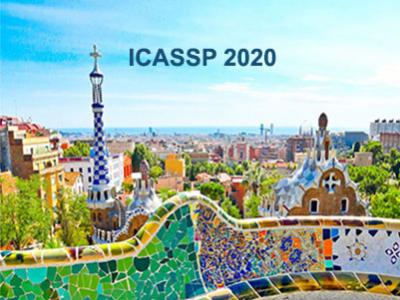
- Read more about Deep geometric knowledge distillation with graphs
- Log in to post comments
- Categories:
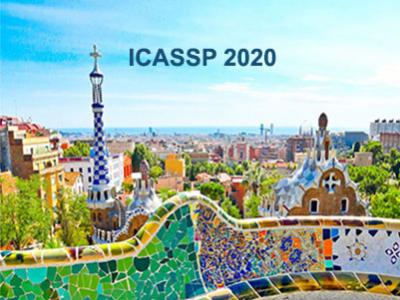
- Read more about A NOVEL TWO-PATHWAY ENCODER-DECODER NETWORK FOR 3D FACE RECONSTRUCTION
- Log in to post comments
3D Morphable Model (3DMM) is a statistical tool widely employed in reconstructing 3D face shape. Existing methods are aimed at predicting 3DMM shape parameters with a single encoder but suffer from unclear distinction of different attributes. To address this problem, Two-Pathway Encoder-Decoder Network (2PEDN) is proposed to regress the identity and expression components via global and local pathways. Specifically, each 2D face image is cropped into global face and local details as the inputs for the corresponding pathways.
- Categories:
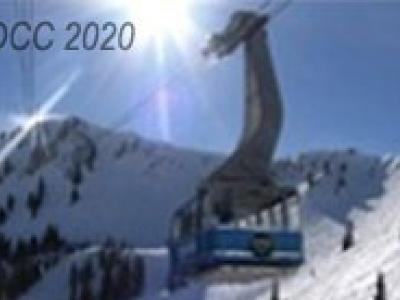
- Categories:
- Read more about Lossless Multi-Component Image Compression based on Integer Wavelet Coefficient Prediction using Convolutional Neural Networks
- Log in to post comments
- Categories:
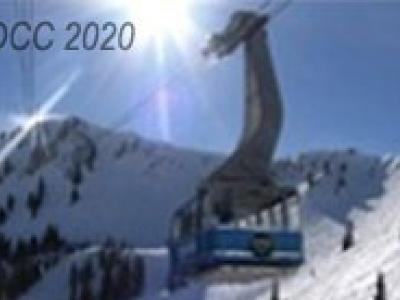
- Read more about Segmentation of Text-Lines and Words from JPEG Compressed Printed Text Documents Using DCT Coefficients
- Log in to post comments
Segmenting a document image into text-lines and words finds applications in many research areas of DIA(Document Image Analysis) such as OCR, Word Spotting, and document retrieval. However, carrying out segmentation operation directly in the compressed document images is still an unexplored and challenging research area. Since JPEG is most widely accepted compression algorithm, this research paper attempts to segment a JPEG compressed printed text document image into text-lines and words, without fully decompressing the image.
dccv.pdf

- Categories:
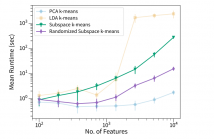
- Read more about Improved Subspace K-Means Performance via a Randomized Matrix Decomposition
- Log in to post comments
Subspace clustering algorithms provide the capability
to project a dataset onto bases that facilitate clustering.
Proposed in 2017, the subspace k-means algorithm simultaneously
performs clustering and dimensionality reduction with the goal
of finding the optimal subspace for the cluster structure; this
is accomplished by incorporating a trade-off between cluster
and noise subspaces in the objective function. In this study,
we improve subspace k-means by estimating a critical transformation
- Categories:
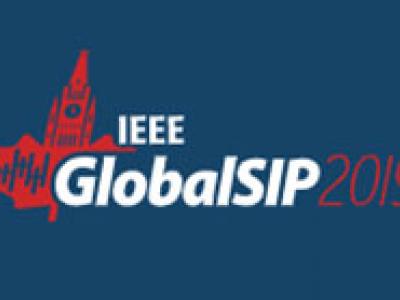
- Read more about Poster: Generative-Discriminative Crop Type Identification using Satellite Images
- Log in to post comments
Crop type identification refers to distinguishing certain crop from other landcovers, which is an essential and crucial task in agricultural monitoring. Satellite images are good data input for identifying different crops since satellites capture relatively wider area and more spectral information. Based on prior knowledge of crop phenology, multi-temporal images are stacked to extract the growth pattern of varied crops.
- Categories:
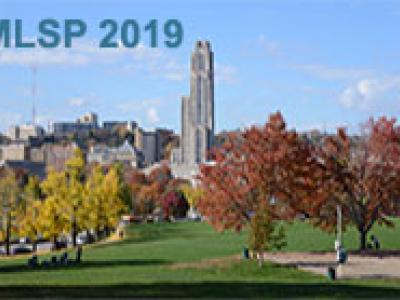
- Read more about A deep network for single-snapshot direction of arrival estimation
- Log in to post comments
This paper examines a deep feedforward network for beamforming with the single--snapshot Sample Covariance Matrix (SCM). The Conventional beamforming formulation, typically quadratic in the complex weight space, is reformulated as real and linear in the weight covariance and SCM. The reformulated SCMs are used as input to a deep feed--forward neural network (FNN) for two source localization. Simulations demonstrate the effect of source incoherence and performance in a noisy tracking example.
- Categories:
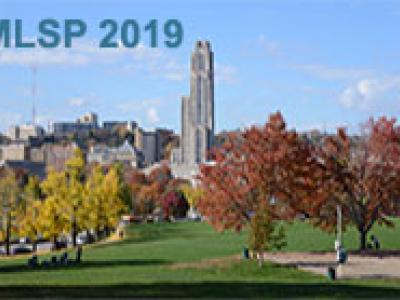
- Read more about Deep Reinforcement Learning Based Energy Beamforming for Powering Sensor Networks
- 1 comment
- Log in to post comments
We focus on a wireless sensor network powered with an energy beacon, where sensors send their measurements to the sink using the harvested energy. The aim of the system is to estimate an unknown signal over the area of interest as accurately as possible. We investigate optimal energy beamforming at the energy beacon and optimal transmit power allocation at the sensors under non-linear energy harvesting models. We use a deep reinforcement learning (RL) based approach where multi-layer neural networks are utilized.
- Categories: