- Bayesian learning; Bayesian signal processing (MLR-BAYL)
- Bounds on performance (MLR-PERF)
- Applications in Systems Biology (MLR-SYSB)
- Applications in Music and Audio Processing (MLR-MUSI)
- Applications in Data Fusion (MLR-FUSI)
- Cognitive information processing (MLR-COGP)
- Distributed and Cooperative Learning (MLR-DIST)
- Learning theory and algorithms (MLR-LEAR)
- Neural network learning (MLR-NNLR)
- Information-theoretic learning (MLR-INFO)
- Independent component analysis (MLR-ICAN)
- Graphical and kernel methods (MLR-GRKN)
- Other applications of machine learning (MLR-APPL)
- Pattern recognition and classification (MLR-PATT)
- Source separation (MLR-SSEP)
- Sequential learning; sequential decision methods (MLR-SLER)
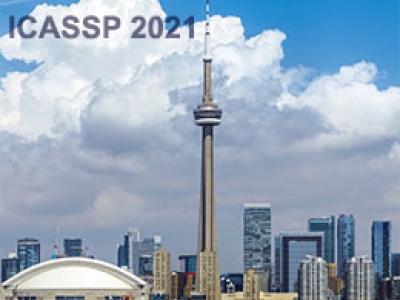
- Read more about SIG2SIG : SIGNAL TRANSLATION NETWORKS TO TAKE THE REMAINS OF THE PAST
- Log in to post comments
- Categories:
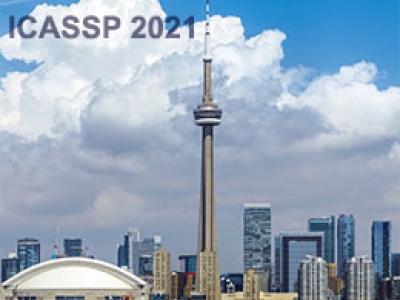
- Read more about Probabilistic Graph Neural Networks for Traffic Signal Control
- Log in to post comments
Intelligent traffic signal control is crucial for efficient
transportation systems. Recent studies use reinforcement
learning (RL) to coordinate traffic signals and improve traffic
signal cooperation. However, they either design the state of
agents in a heuristic manner or model traffic dynamics in a deterministic way. This work presents a variational graph learning model TSC-GNN (Traffic Signal Control via probabilistic
Graph Neural Networks) to learn the latent representations of
agents and generate Q-value while taking traffic uncertainty
- Categories:
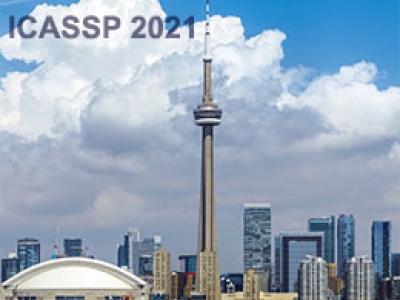
- Read more about Deep Weighted MMSE Downlink Beamforming
- Log in to post comments
- Categories:
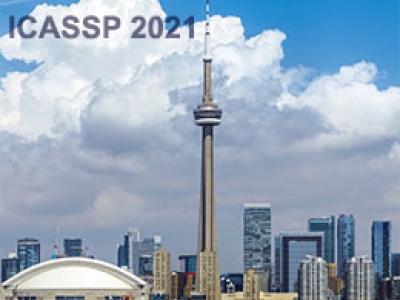
- Read more about Modurec: Recommender Systems with Feature and Time Modulation
- Log in to post comments
Current state of the art algorithms for recommender systems are mainly based on collaborative filtering, which exploits user ratings to discover latent factors in the data. These algorithms unfortunately do not make effective use of other features, which can help solve two well identified problems of collaborative filtering: cold start (not enough data is available for new users or products) and concept shift (the distribution of ratings changes over time).
- Categories:
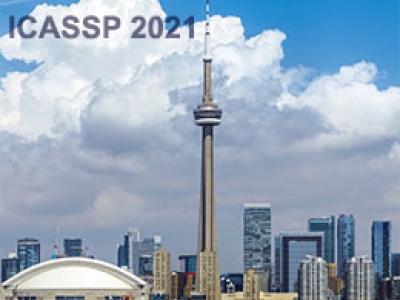
- Read more about Modurec: Recommender Systems with Feature and Time Modulation
- Log in to post comments
Current state of the art algorithms for recommender systems are mainly based on collaborative filtering, which exploits user ratings to discover latent factors in the data. These algorithms unfortunately do not make effective use of other features, which can help solve two well identified problems of collaborative filtering: cold start (not enough data is available for new users or products) and concept shift (the distribution of ratings changes over time).
- Categories:
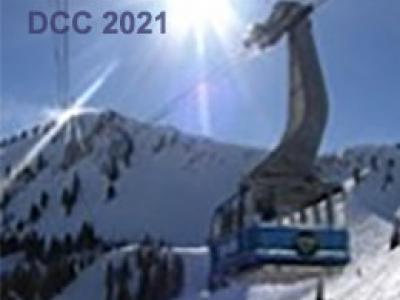
- Read more about An Empirical Analysis of Recurrent Learning Algorithms In Neural Lossy Image Compression Systems.
- 1 comment
- Log in to post comments
Prior work on image compression has focused on optimizing models to achieve better reconstruction at lower bit rates. These approaches are focused on creating sophisticated architectures that enhance encoder or decoder performance. In some cases, there is the desire to jointly optimize both along with a designed form of entropy encoding. In some instances, these approaches result in the creation of many redundant components, which may or may not be useful.
- Categories:
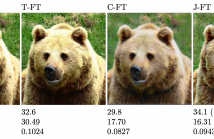
- Read more about End-to-end deep-learning-based image compression for machine tasks, a study
- Log in to post comments
This paper provides an in-depth study of a framework consisting of combining trainable im- age and video codecs with machine task algorithms. The field of video coding optimization for machines is gaining traction, due to the increasing share of image and video content that is dedicated to be analyzed by machines, rather than viewed by humans. Recent works in image compression have demonstrated the potential of end-to-end deep-learning-based auto-encoders that can be trained to optimally compress images and videos with respect to a target rate and any given differentiable quality metric.
- Categories:
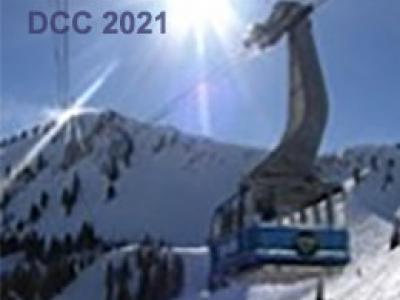
- Read more about Hybrid Intra-Prediction in Lossless Video Coding using Overfitted Neural Networks
- Log in to post comments
- Categories:
- Read more about HH-CompWordNet: Holistic Handwritten Word Recognition in the Compressed Domain
- Log in to post comments
Holistic word recognition in handwritten documents is an important research topic in the field of Document Image Analysis. For some applications, given strong language models, it can be more robust and computationally less expensive than character segmentation and recognition. This paper presents HH-CompWordNet, a novel approach to applying a Convolutional Neural Network (CNN) to directly to the DCT coefficients of the compressed domain word images.
- Categories: