- Bayesian learning; Bayesian signal processing (MLR-BAYL)
- Bounds on performance (MLR-PERF)
- Applications in Systems Biology (MLR-SYSB)
- Applications in Music and Audio Processing (MLR-MUSI)
- Applications in Data Fusion (MLR-FUSI)
- Cognitive information processing (MLR-COGP)
- Distributed and Cooperative Learning (MLR-DIST)
- Learning theory and algorithms (MLR-LEAR)
- Neural network learning (MLR-NNLR)
- Information-theoretic learning (MLR-INFO)
- Independent component analysis (MLR-ICAN)
- Graphical and kernel methods (MLR-GRKN)
- Other applications of machine learning (MLR-APPL)
- Pattern recognition and classification (MLR-PATT)
- Source separation (MLR-SSEP)
- Sequential learning; sequential decision methods (MLR-SLER)
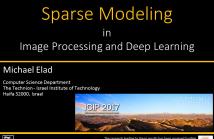
- Read more about Sparse Modeling
- Log in to post comments
Sparse Modeling in Image Processing and Deep LearningSparse approximation is a well-established theory, with a profound impact on the fields of signal and image processing. In this talk we start by presenting this model and its features, and then turn to describe two special cases of it – the convolutional sparse coding (CSC) and its multi-layered version (ML-CSC). Amazingly, as we will carefully show, ML-CSC provides a solid theoretical foundation to … deep-learning.
- Categories:
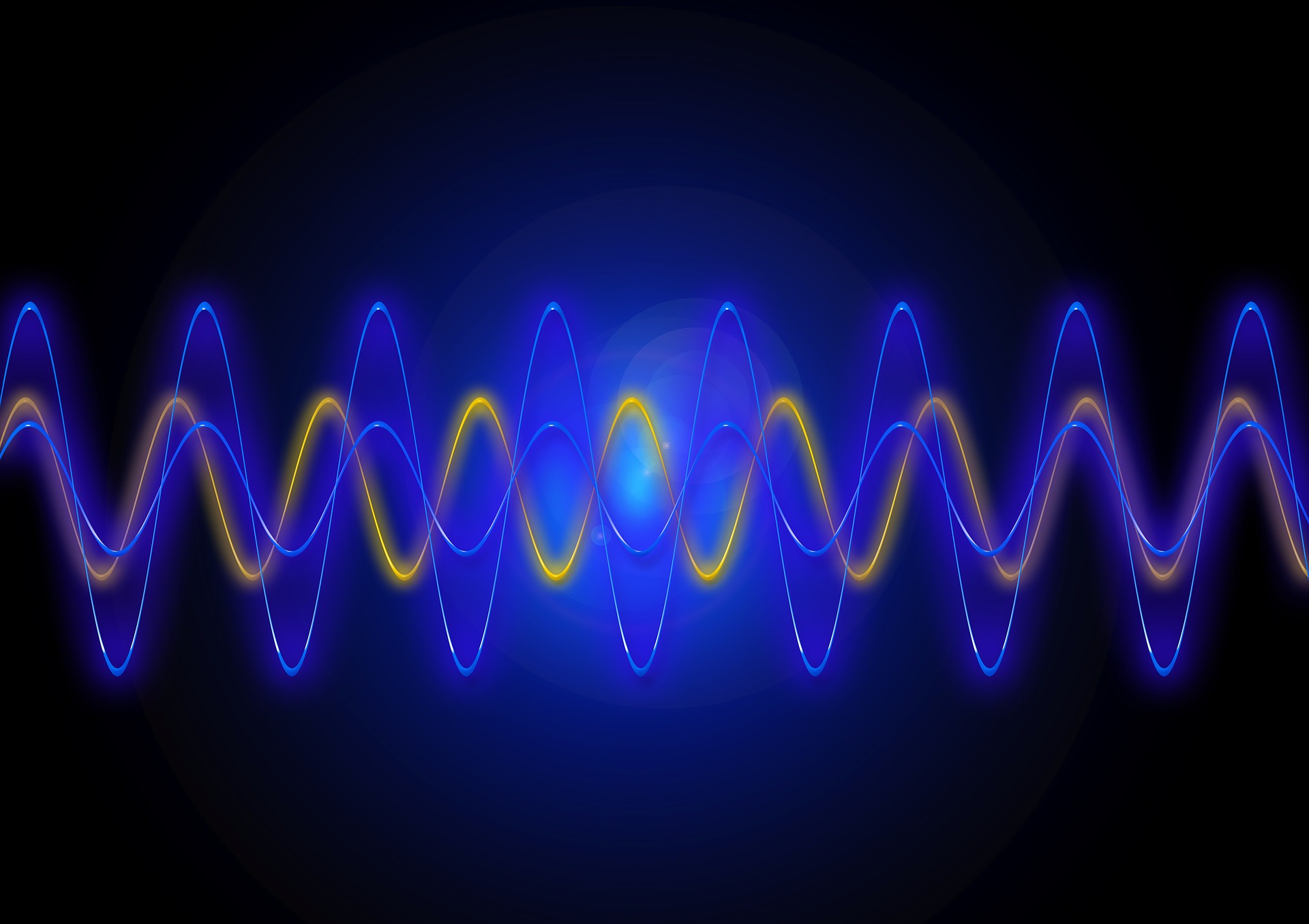
- Read more about SUPPLEMENTARYMATERIALSFOREVENTDENOISINGBASEDONITERATIVE TREE-STRUCTUREDINFORMATIONAGGREGATION
- Log in to post comments
SUPPLEMENTARY_MATERIALS_FOR__EVENT_DENOISING_BASED_ON_ITERATIVE_TREE_STRUCTURED_INFORMATION_AGGREGATION
- Categories:
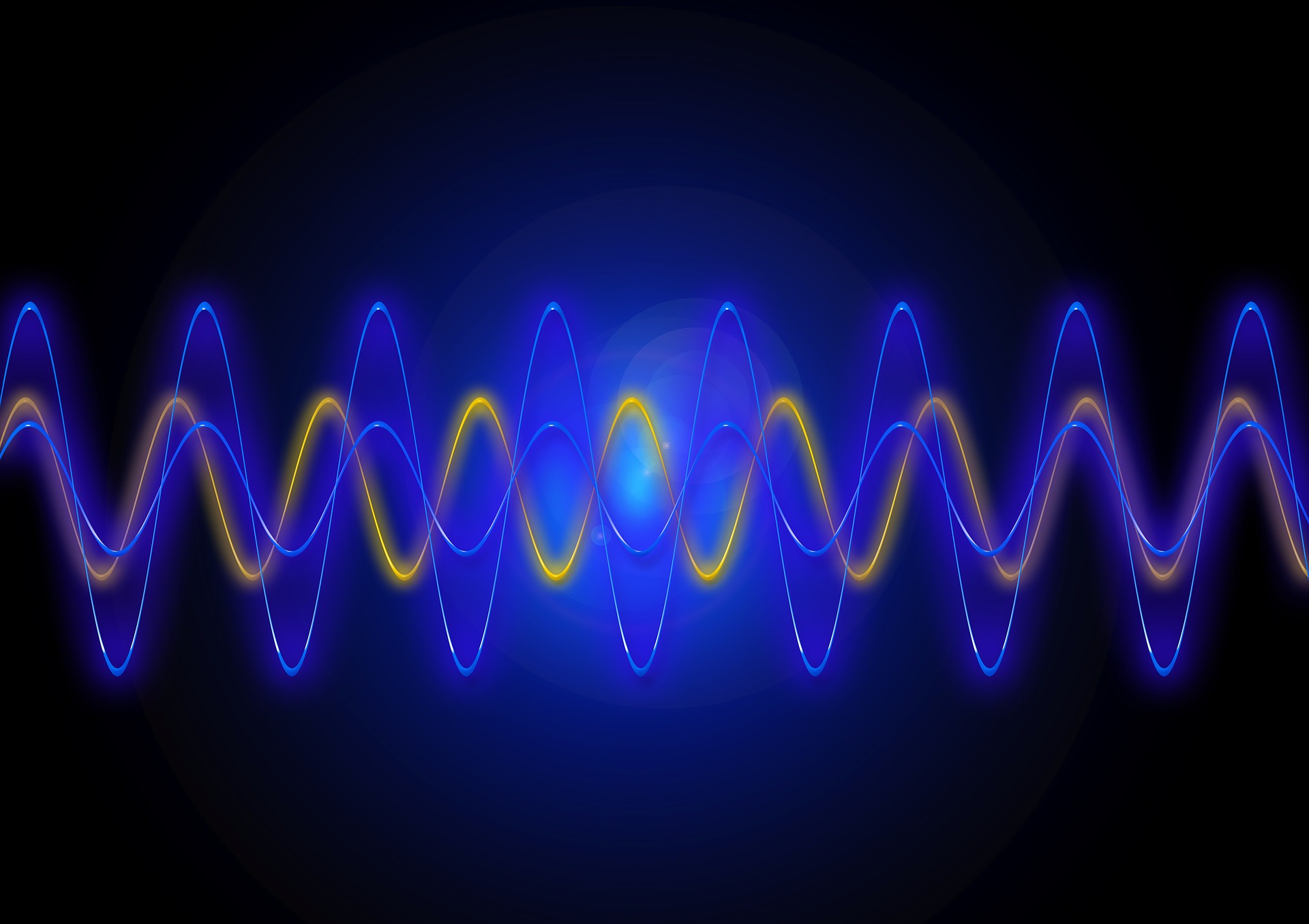
- Read more about Supplementary material
- Log in to post comments
Supplementary material for the paper titled "TARGET DRIVEN ADAPTIVE LOSS FOR INFRARED SMALL TARGET DETECTION"
- Categories:
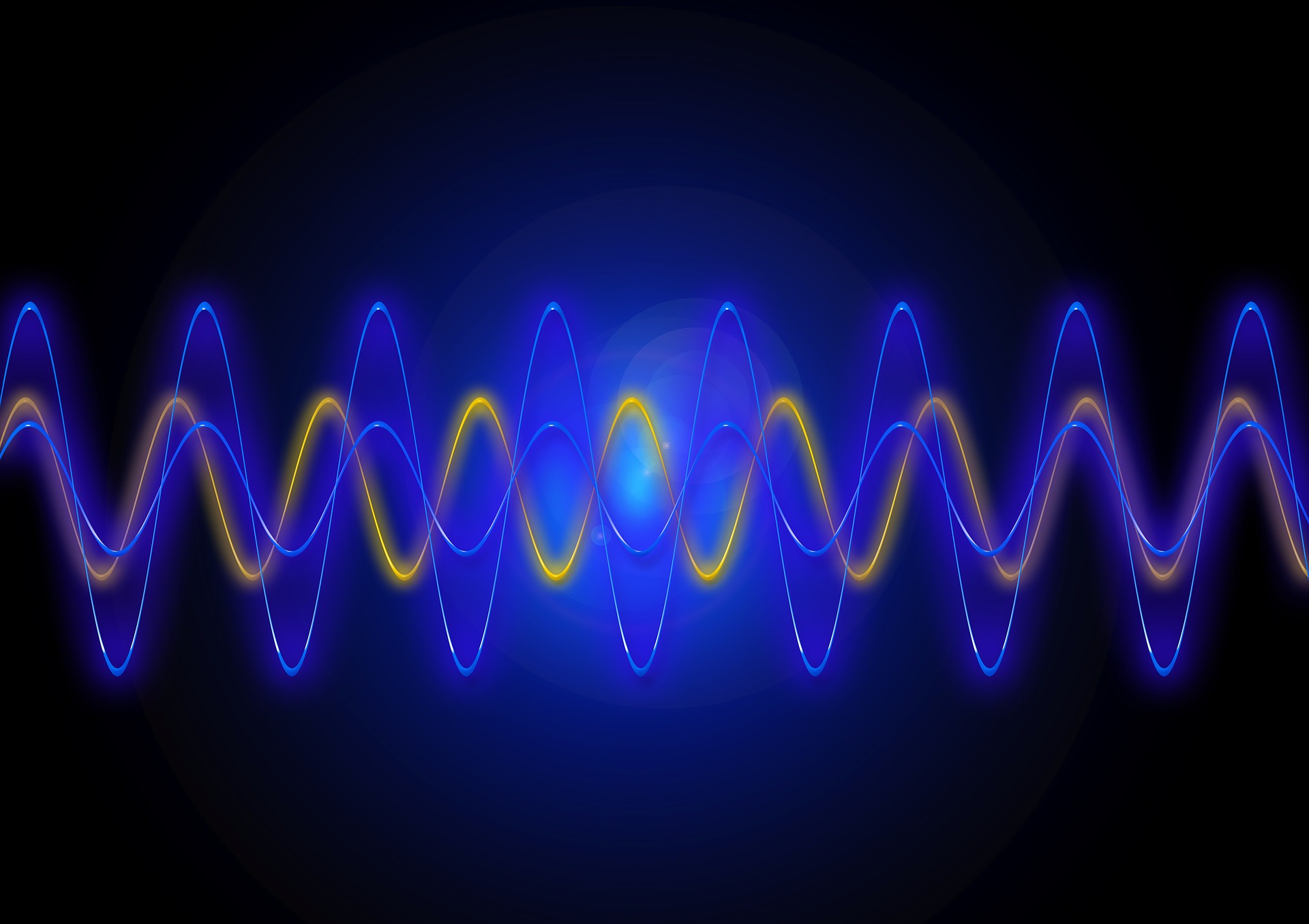
- Read more about SUPPLEMENTARY MATERIALS FOR EVENT DENOISING BASED ON ITERATIVE TREE-STRUCTURED INFORMATION AGGREGATIO
- Log in to post comments
SUPPLEMENTARY MATERIALS FOR EVENT DENOISING BASED ON ITERATIVE
TREE-STRUCTURED INFORMATION AGGREGATIO
- Categories:
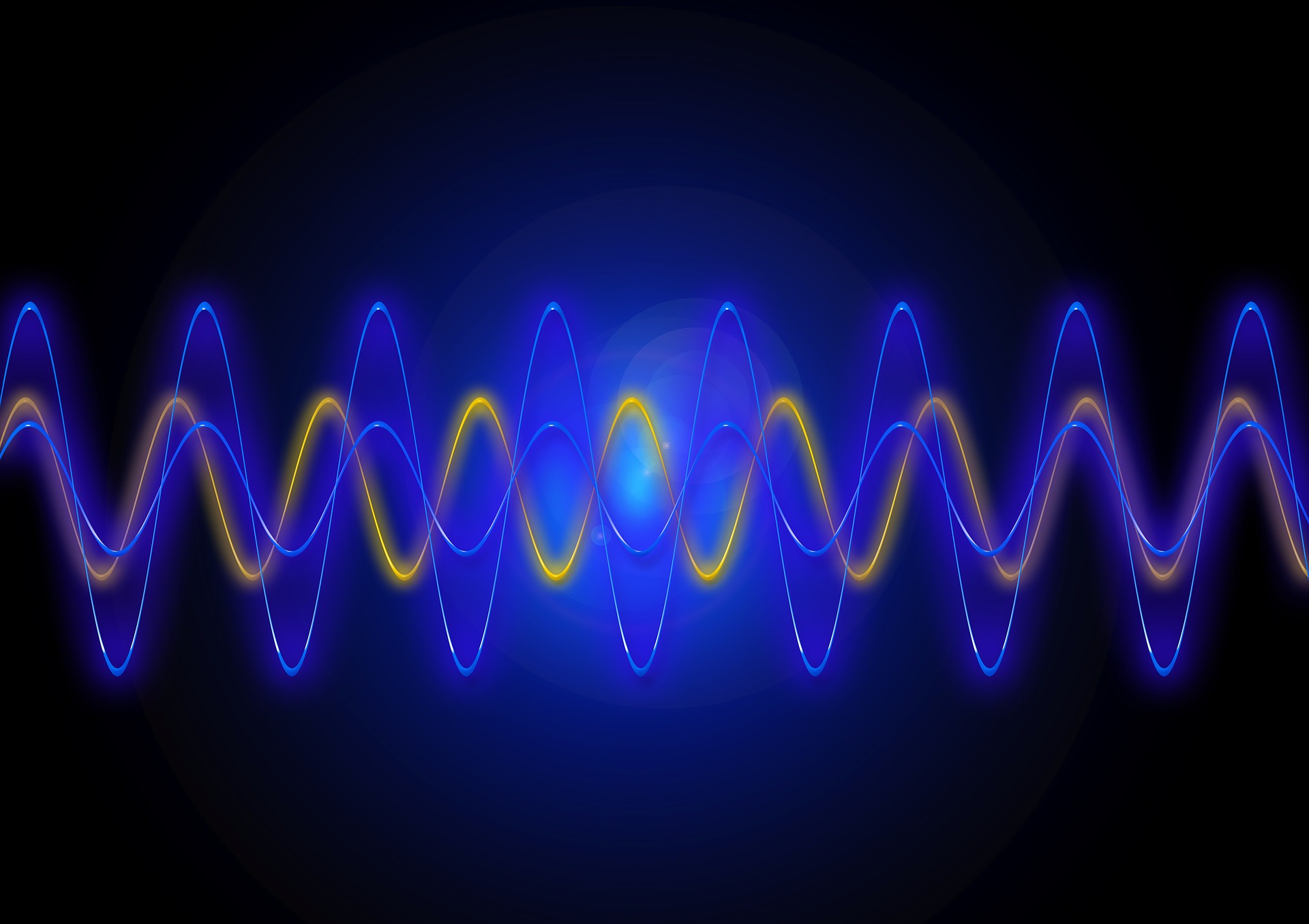
- Read more about ICIP2025_SUPPLEMENTARY_MODEL_ATTACK
- Log in to post comments
Recent advancements in diffusion models have enabled high-fidelity and photorealistic image generation across diverse applications. However, these models also present security and privacy risks, including copyright violations, sensitive information leakage, and the creation of harmful or offensive content that could be exploited maliciously. In this study, we uncover a novel security threat where an attacker leverages diffusion model APIs to generate synthetic images, which are then used to train a high-performing substitute model.
- Categories:
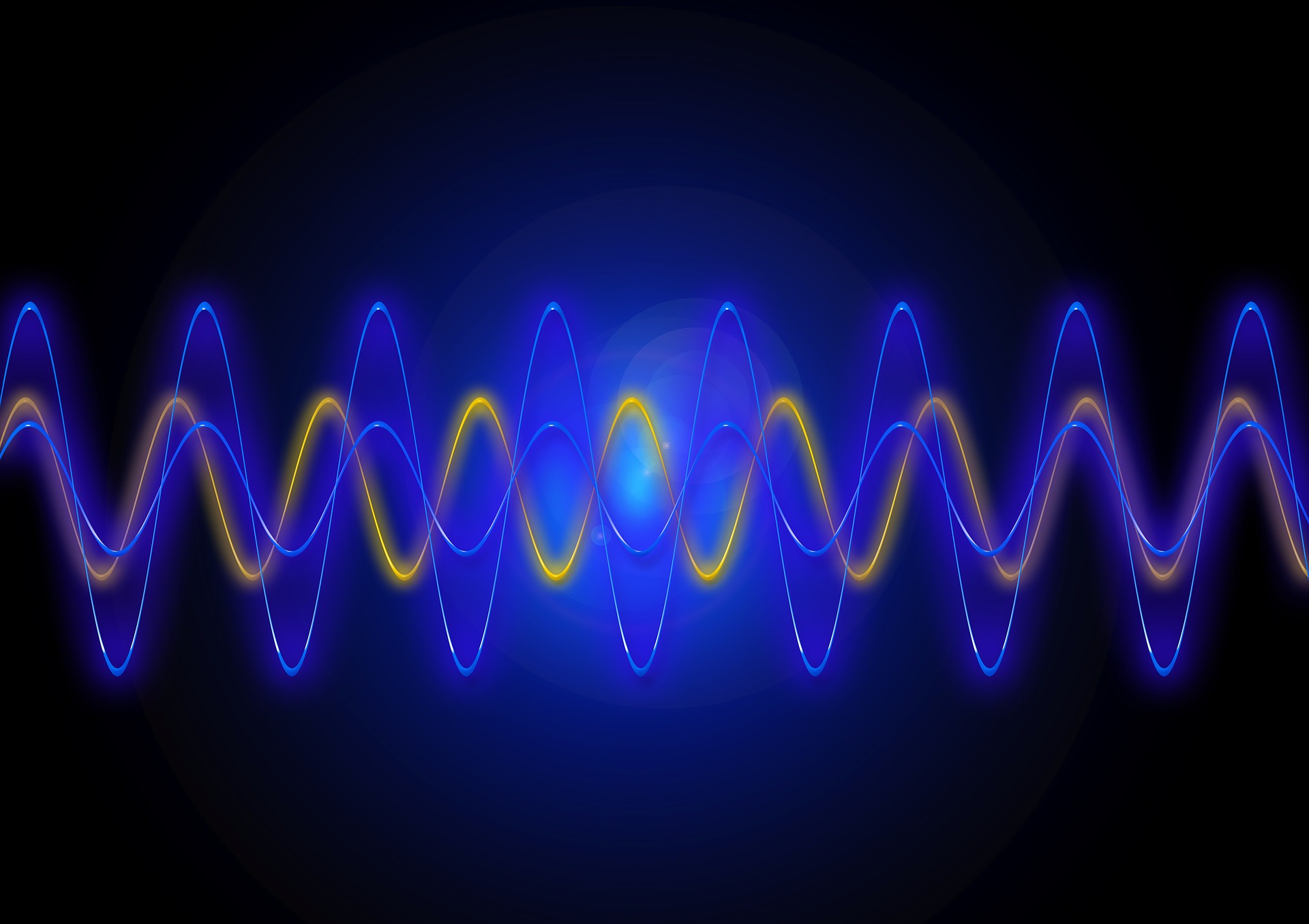
- Read more about Handling Multiple Hypotheses in Coarse-to-Fine Dense Image Matching Supp Mat
- Log in to post comments
Dense image matching aims to find a correspondent for every pixel of a source image in a partially overlapping target image. State-of-the-art methods typically rely on a coarse-to-fine mechanism where a single correspondent hypothesis is produced per source location at each scale. In challenging cases -- such as at depth discontinuities or when the target image is a strong zoom-in of the source image -- the correspondents of neighboring source locations are often widely spread and predicting a single correspondent hypothesis per source location at each scale may lead to erroneous matches.
- Categories:
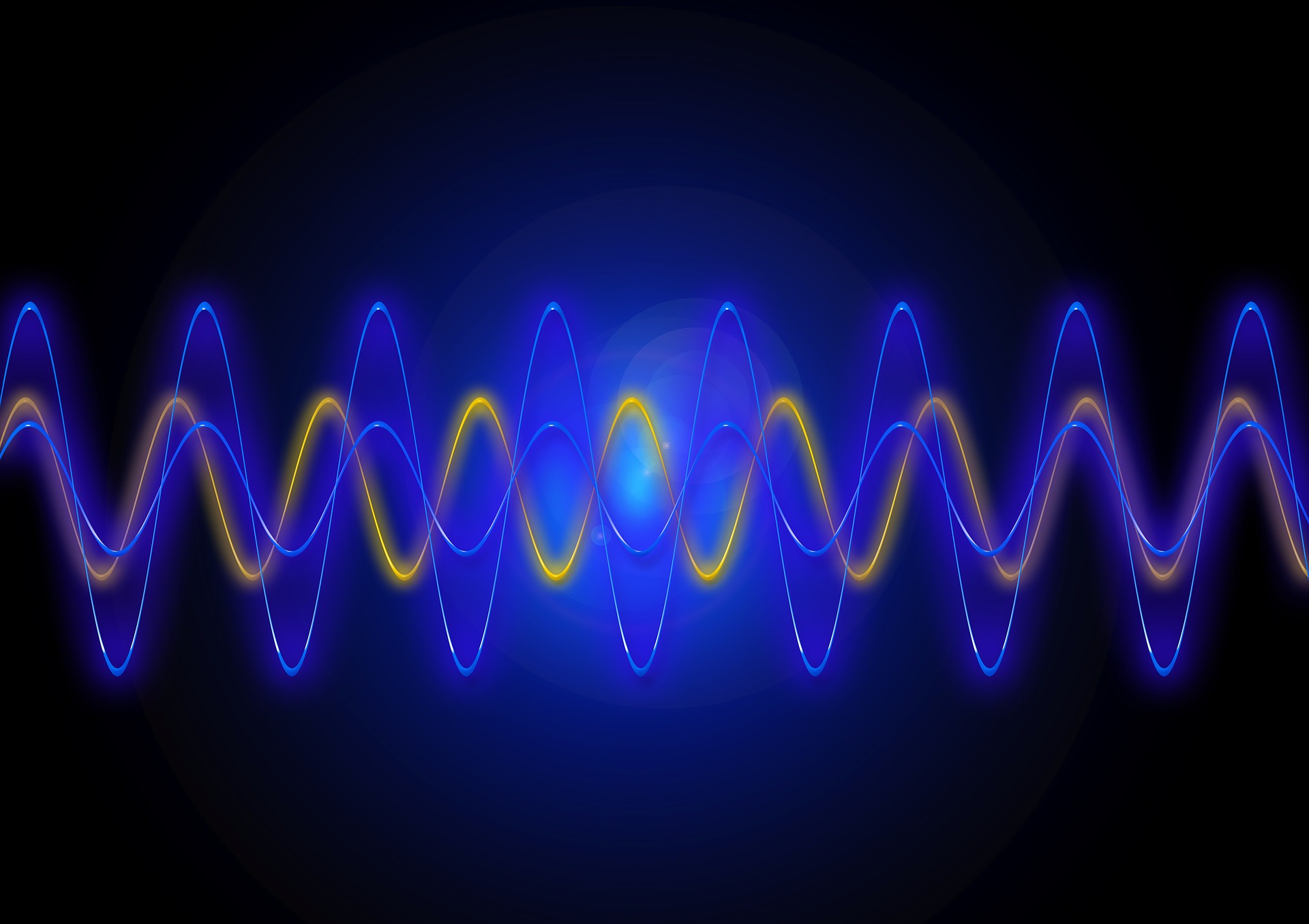
- Read more about Supplementary Material: A Novel Context-adaptive Fusion of Shadow and Highlight Regions for Efficient Sonar Image Classification
- Log in to post comments
Sonar imaging is fundamental to underwater exploration, with critical applications in defense, navigation, and marine research. Shadow regions, in particular, provide essential cues for object detection and classification, yet existing studies primarily focus on highlight-based analysis, leaving shadow-based classification underexplored. To bridge this gap, we propose a Context-adaptive sonar image classification framework that leverages advanced image processing techniques to extract and integrate discriminative shadow and highlight features.
- Categories:
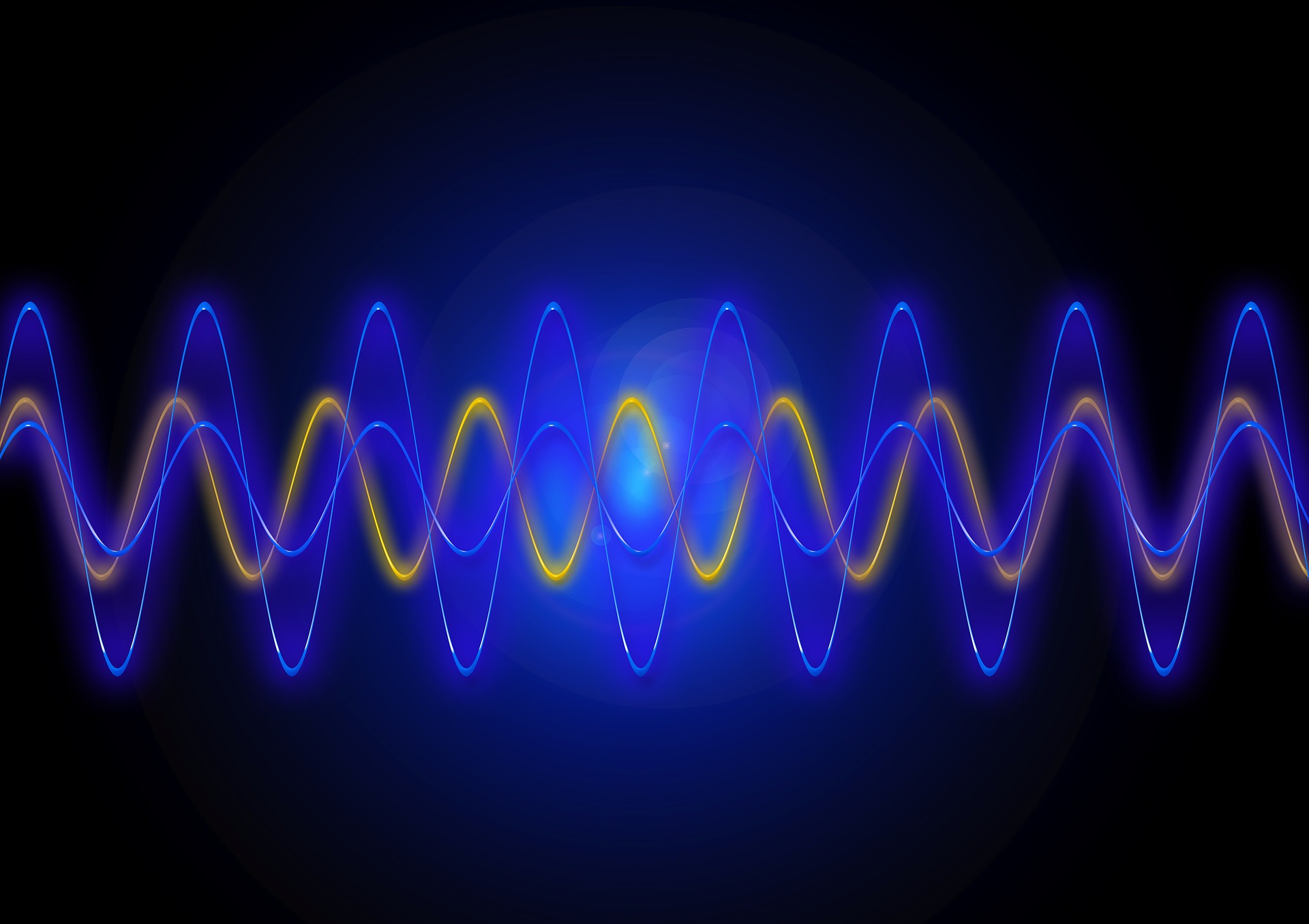
Despeckling of Synthetic Aperture Radar (SAR) images has seen significant progress in recent years, largely driven by advancements in deep learning techniques. However, many of these approaches face challenges when applied to new SAR datasets, primarily due to their dependence on ground truth images, which are often unavailable for real-world sensors. In this paper, we address this limitation by extending the concept of unbiased risk estimation in the presence of Gamma-distributed multiplicative speckle.
- Categories:
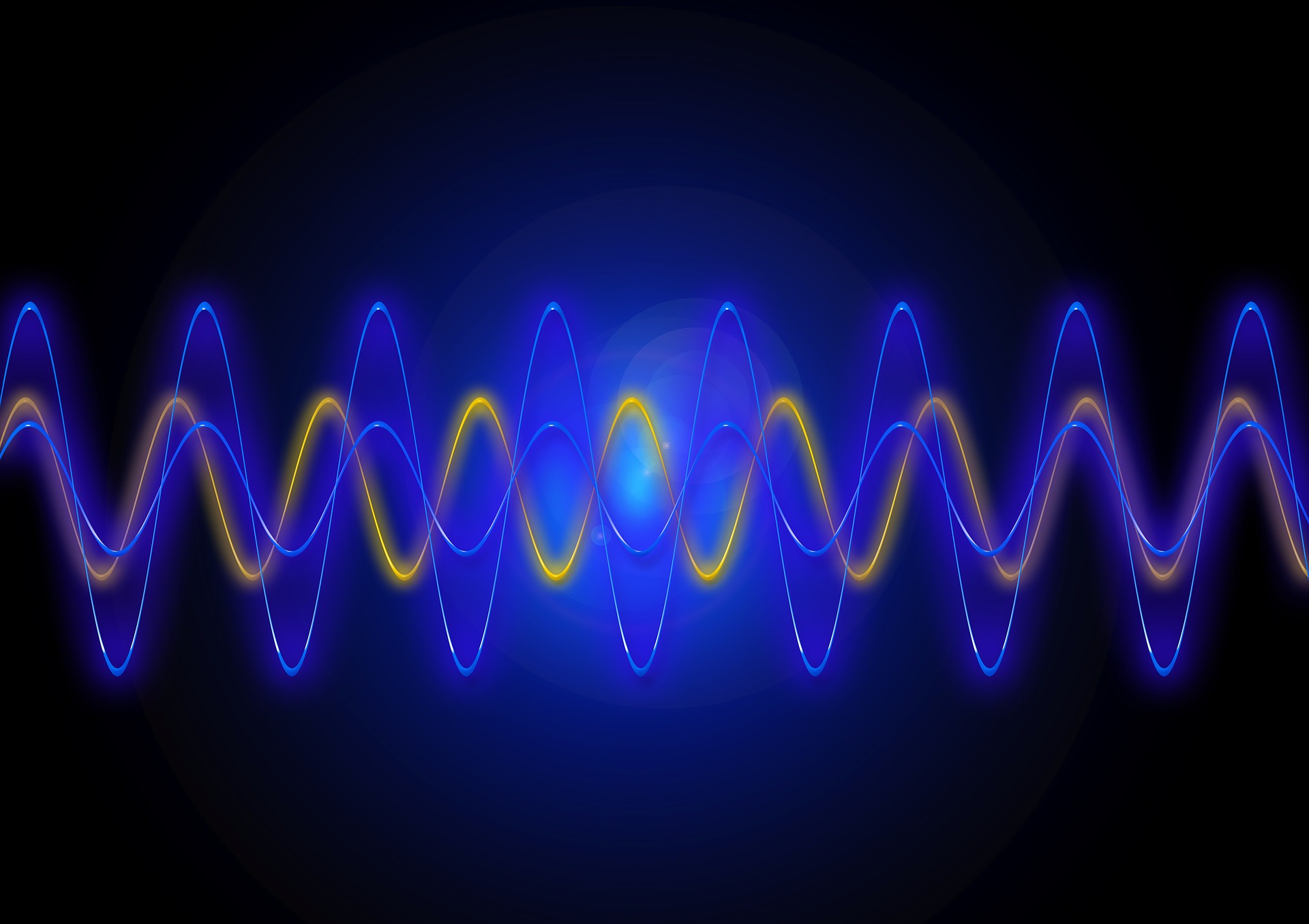
- Read more about Supplementary Materials for Diffusion Pretraining for Gait Recognition in the WIld
- Log in to post comments
Supplementary Materials for Diffusion Pretraining for Gait Recognition in the Wild
- Categories:
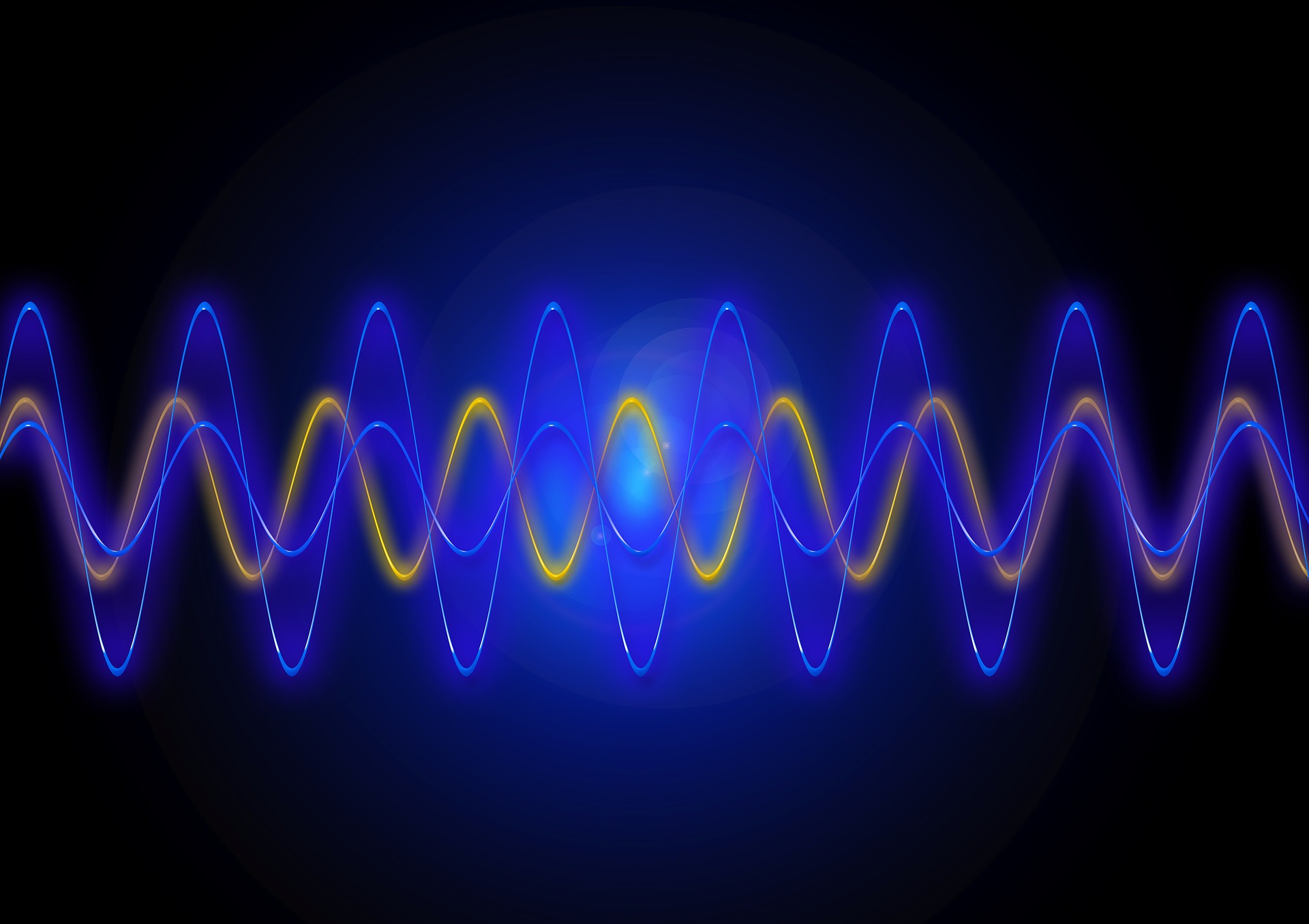
- Read more about Supplementary Material for EventEgoHands: Event-based Egocentric 3D Hand Mesh Reconstruction
- Log in to post comments
Supplementary Material for EventEgoHands: Event-based Egocentric 3D Hand Mesh Reconstruction
- Categories: