- Bayesian learning; Bayesian signal processing (MLR-BAYL)
- Bounds on performance (MLR-PERF)
- Applications in Systems Biology (MLR-SYSB)
- Applications in Music and Audio Processing (MLR-MUSI)
- Applications in Data Fusion (MLR-FUSI)
- Cognitive information processing (MLR-COGP)
- Distributed and Cooperative Learning (MLR-DIST)
- Learning theory and algorithms (MLR-LEAR)
- Neural network learning (MLR-NNLR)
- Information-theoretic learning (MLR-INFO)
- Independent component analysis (MLR-ICAN)
- Graphical and kernel methods (MLR-GRKN)
- Other applications of machine learning (MLR-APPL)
- Pattern recognition and classification (MLR-PATT)
- Source separation (MLR-SSEP)
- Sequential learning; sequential decision methods (MLR-SLER)
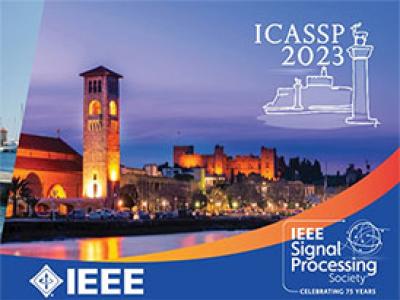
- Read more about Cross-site Generalization for imbalanced epileptic classification
- Log in to post comments
Recently, many studies have been conducted on automated epileptic seizures detection. However, few of these techniques are applied in clinical settings for several reasons. One of them is the imbalanced nature of the seizure detection task. Additionally, the current detection techniques do not really generalize to other patient populations. To address these issues, we present in this paper a hybrid CNN-LSTM model robust to cross-site variability. We investigate the use of data augmentation (DA) methods as an efficient tool to solve imbalanced training problems.
- Categories:
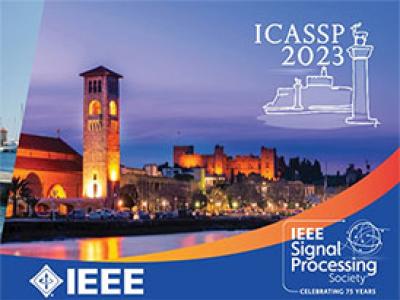
- Read more about Cross-site Generalization for imbalanced epileptic classification
- Log in to post comments
Recently, many studies have been conducted on automated epileptic seizures detection. However, few of these techniques are applied in clinical settings for several reasons. One of them is the imbalanced nature of the seizure detection task. Additionally, the current detection techniques do not really generalize to other patient populations. To address these issues, we present in this paper a hybrid CNN-LSTM model robust to cross-site variability. We investigate the use of data augmentation (DA) methods as an efficient tool to solve imbalanced training problems.
- Categories:
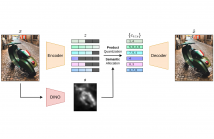
Vector-quantized autoencoders have recently gained interest in image compression, generation and self-supervised learning. However, as a neural compression method, they lack the possibility to allocate a variable number of bits to each image location, e.g. according to the semantic content or local saliency. In this paper, we address this limitation in a simple yet effective way. We adopt a product quantizer (PQ) that produces a set of discrete codes for each image patch rather than a single index.
- Categories:

- Read more about Gluformer: Transformer-Based Personalized Glucose Forecasting with Uncertainty Quantification
- Log in to post comments
Deep learning models achieve state-of-the art results in predicting blood glucose trajectories, with a wide range of architectures being proposed. However, the adaptation of such models in clinical practice is slow, largely due to the lack of uncertainty quantification of provided predictions. In this work, we propose to model the future glucose trajectory conditioned on the past as an infinite mixture of basis distributions (i.e., Gaussian, Laplace, etc.).
- Categories:
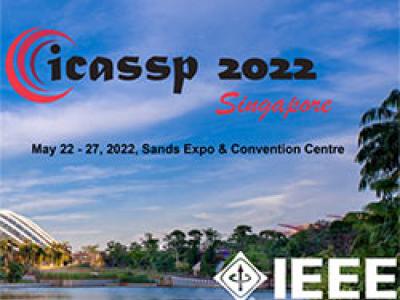
- Read more about ROBUST NONPARAMETRIC DISTRIBUTION FORECAST WITH BACKTEST-BASED BOOTSTRAP AND ADAPTIVE RESIDUAL SELECTION
- Log in to post comments
Distribution forecast can quantify forecast uncertainty and provide various forecast scenarios with their corresponding estimated probabilities. Accurate distribution forecast is crucial for planning - for example when making production capacity or inventory allocation decisions. We propose a practical and robust distribution forecast framework that relies on backtest-based bootstrap and adaptive residual selection.
- Categories:
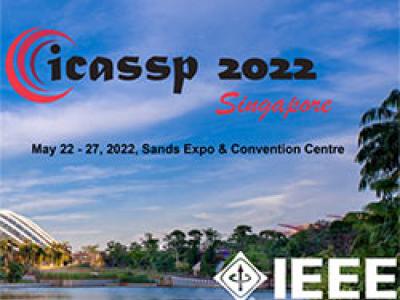
- Read more about Hand Gesture Recognition Using Temporal Convolutions and Attention Mechanism
- Log in to post comments
- Categories:
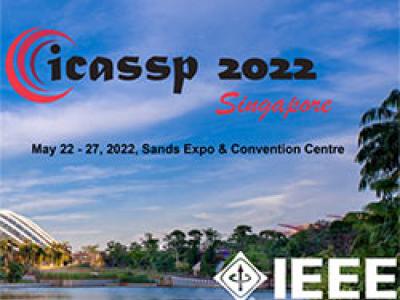
- Read more about PEER COLLABORATIVE LEARNING FOR POLYPHONIC SOUND EVENT DETECTION
- Log in to post comments
This paper describes how semi-supervised learning, called peer collaborative learning (PCL), can be applied to the polyphonic sound event detection (PSED) task, which is one of the tasks in the Detection and Classification of Acoustic Scenes and Events (DCASE) challenge. Many deep learning models have been studied to determine what kind of sound events occur where and for how long in a given audio clip.
- Categories:
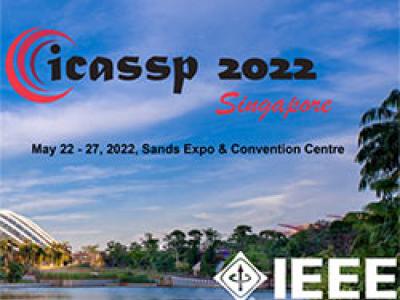
- Read more about Spatio-Temporal PRRS Epidemic Forecasting via Factorized Deep Generative Modeling
- Log in to post comments
Abstract:
- Categories:
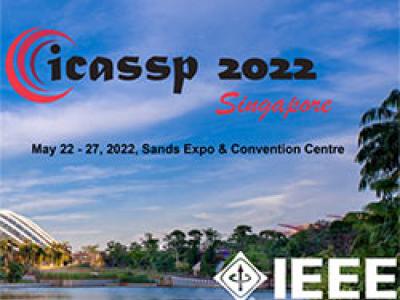
In this paper, we focus on learning sparse graphs with a core-periphery structure. We propose a generative model for data associated with core-periphery structured networks to model the dependence of node attributes on core scores of the nodes of a graph through a latent graph structure. Using the proposed model, we jointly infer a sparse graph and nodal core scores that induce dense (sparse) connections in core (respectively, peripheral) parts of the network.
- Categories:
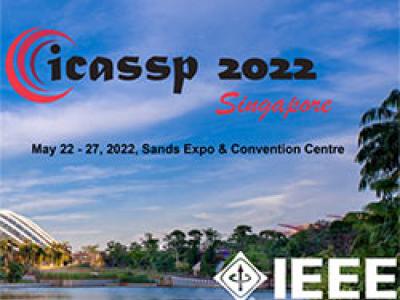
- Read more about MTAF: SHOPPING GUIDE MICRO-VIDEOS POPULARITY PREDICTION USING MULTIMODAL AND TEMPORAL ATTENTION FUSION APPROACH
- Log in to post comments
Predicting the popularity of shopping guide micro-videos incorporating merchandise is crucial for online advertising. What are the significant factors affecting the popularity of the micro-video? How to extract and effectively fuse multiple modalities for the micro-video popularity prediction? This is a question that needs to be urgently answered to better provide insights for advertisers. In this paper, we propose a Multimodal and Temporal Attention Fusion (MTAF) framework to represent and combine multi-modal features.
- Categories: